Siamese-Based Twin Attention Network for Visual Tracking
IEEE Transactions on Circuits and Systems for Video Technology(2023)
摘要
Recently, object tracking have achieved remarkable progress in terms of both efficiency and accuracy. However, exiting methods still cannot satisfy challenging tasks under complicated scenarios, such as occlusion, scale variations, and etc. To this end, we propose a novel Siamese-based Twin Attention Network for visual tracking. First, a multi-branch fusion module is presented. By leveaging the fusion scheme, we merge the low-level features with the high-level features extracted from different convolution layers. Then, the representation ability of the target can be enhanced effectively. Second, to fully capture the contextual information in the tracking process, we introduced a global context module into the search branch. Third, to attain robust performance, a saliency mine scheme is employed in the proposed network. Specifically, the self-attention operation is utilized to capture the contextual information from the spatial and channel domain, while the cross-attention operation is to enrich the contextual information relevance by fusing the features between the template and search region. By utilizing these schemes, our tracker can cope well with different challenging scenes. Extensive experiments were conducted on several popular benchmarks, including VOT2016, VOT2018, VOT2019, VOT2021, OTB2013, OTB2015, GOT10k, LaSOT, and NFS. The results demonstrate that the proposed method is effective and achieves competitive results.
更多查看译文
关键词
Siamese network,multi-branch fusion module,global context module,self-attention,cross-attention
AI 理解论文
溯源树
样例
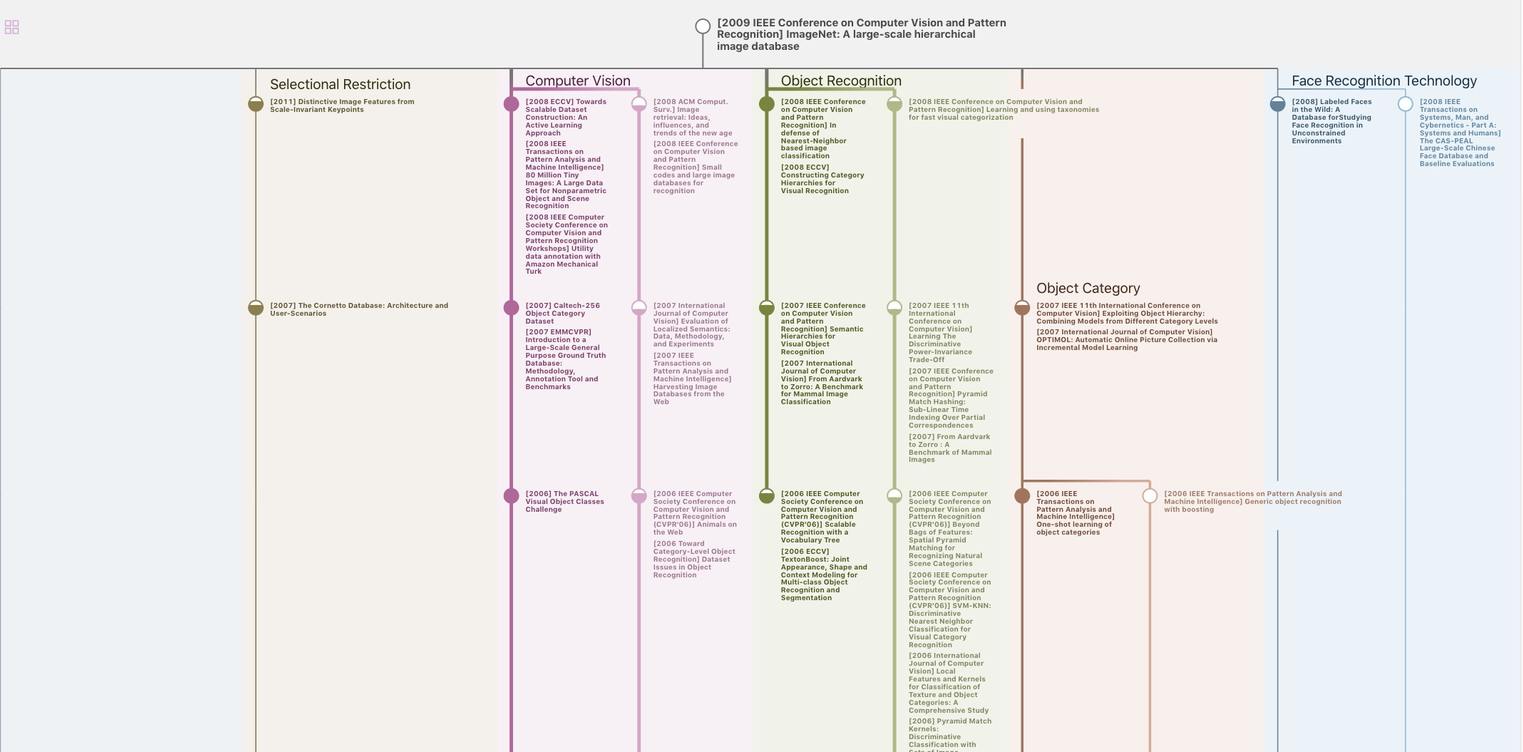
生成溯源树,研究论文发展脉络
Chat Paper
正在生成论文摘要