Reduce the User Burden of Multiuser Myoelectric Interface via Few-Shot Domain Adaptation
IEEE Transactions on Neural Systems and Rehabilitation Engineering(2023)
摘要
Due to physiological and anatomical variations across users, myoelectric interfaces trained by multiple users cannot be adapted to the unique hand movement patterns of the new user. Most current work requires the new user to provide one or more trials per gesture (dozens to hundreds of samples), applying domain adaptation methods to calibrate the model and achieve promising movement recognition performance. However, the user burden associated with time-consuming electromyography signal acquisition and annotation is a key factor hindering the practical application of myoelectric control. As shown in this work, once the number of calibration samples is reduced, the performance of previous cross-user myoelectric interfaces will degrade due to the lack of enough statistics to characterize the distributions. In this paper, a few-shot supervised domain adaptation (FSSDA) framework is proposed to address this issue. It aligns the distributions of different domains by calculating the distribution distances of point-wise surrogates. Specifically, we introduce a positive-negative pair distance loss to find a shared embedding subspace where each scarce sample from the new user will be closer to the positive samples and away from the negative samples of multiple users. Thus, FSSDA allows every target domain sample to be paired with all source domain samples and optimizes the feature distance between each target domain sample and the source domain samples within the same batch, instead of direct estimation of the data distribution of the target domain. The proposed method is validated on two high-density EMG datasets, which achieves the averaged recognition accuracies of 97.59% and 82.78% with only 5 samples per gesture. In addition, FSSDA is also effective even when only one sample per gesture is provided. The experimental results show that FSSDA greatly reduces the user burden and further facilitates the development of myoelectric pattern recognition techniques.
更多查看译文
关键词
Electromyography (EMG),myoelectric pattern recognition,gesture recognition,few-shot domain adaptation
AI 理解论文
溯源树
样例
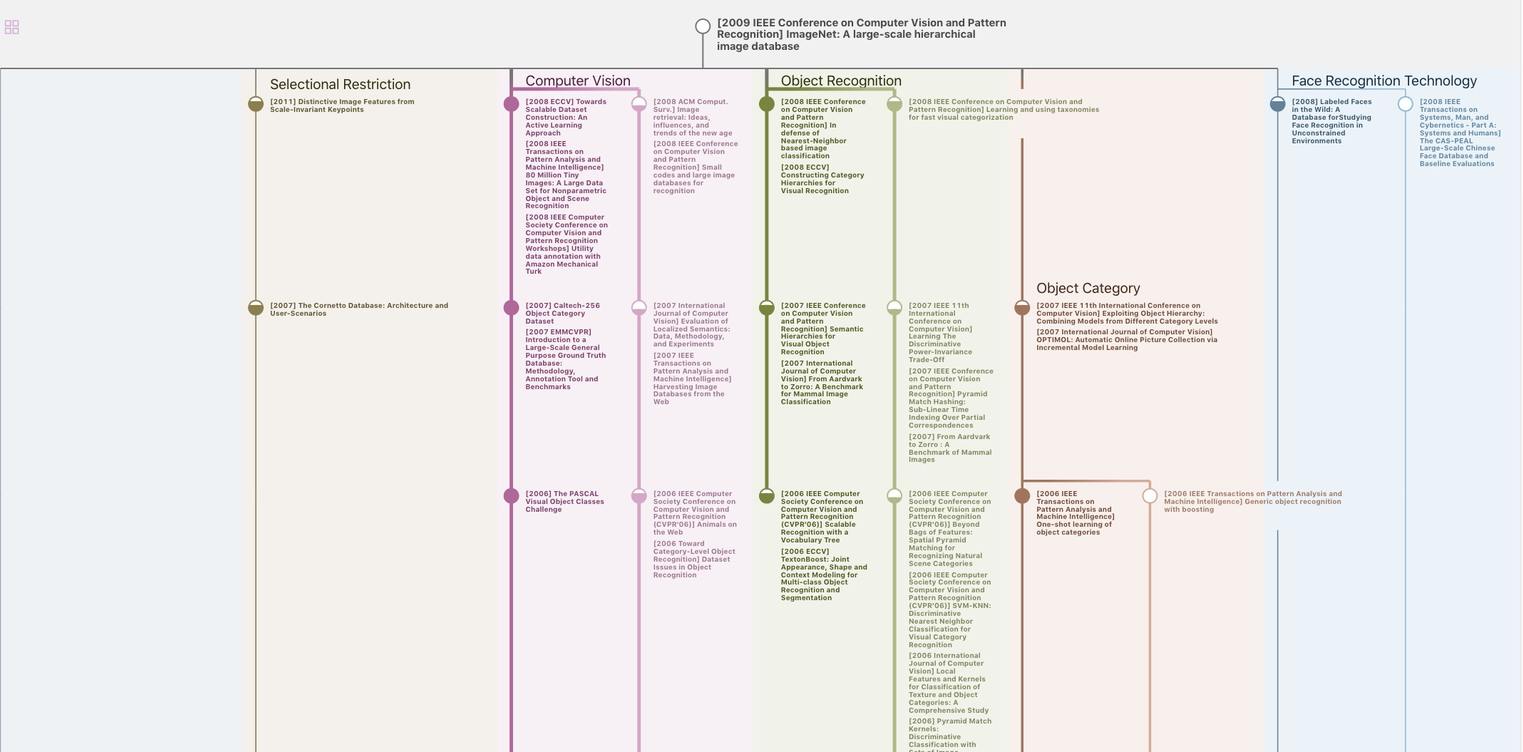
生成溯源树,研究论文发展脉络
Chat Paper
正在生成论文摘要