On Subgrouping Continuous Processes in Discrete Time
Multivariate behavioral research(2023)
摘要
The vector autoregression (VAR) and its flavors have seen a boom in the behavioral sciences over the past decades due to their relative simplicity for analyzing N = 1 time-series and calls to bring the person back to the scientific study of psychology. The rise in N = 1 analyses is then met by a commensurate rise in methods attempting to reconcile person- and group-specific processes for diagnostic purposes and overall generalizability. While these methods have found great success in the last decade, some questions still remain. Some known challenges inherent to VAR-based approaches include the assumption of equal interval between successive observations, and potential inferential confounds when researchers encounter empirical time intervals vary within or across studies. All of these challenges can be ameliorated by fitting models in continuous-time. Yet, methods for finding nomothetic trends in idiographic processes are mainly developed in the discrete time framework. Building on the work of Molenaar and collaborators on subgrouping individual time series by means of the subgrouped chain graphical VAR (scgVAR) and the subgrouping option in the group iterative multiple model estimation (S-GIMME), we present results from a Monte Carlo study aimed at addressing the implications of identifying subgroups using these discrete-time methods when the underlying processes are continuous in nature, and providing insights to guide future design and analytic decisions.
更多查看译文
关键词
continuous processes,discrete time
AI 理解论文
溯源树
样例
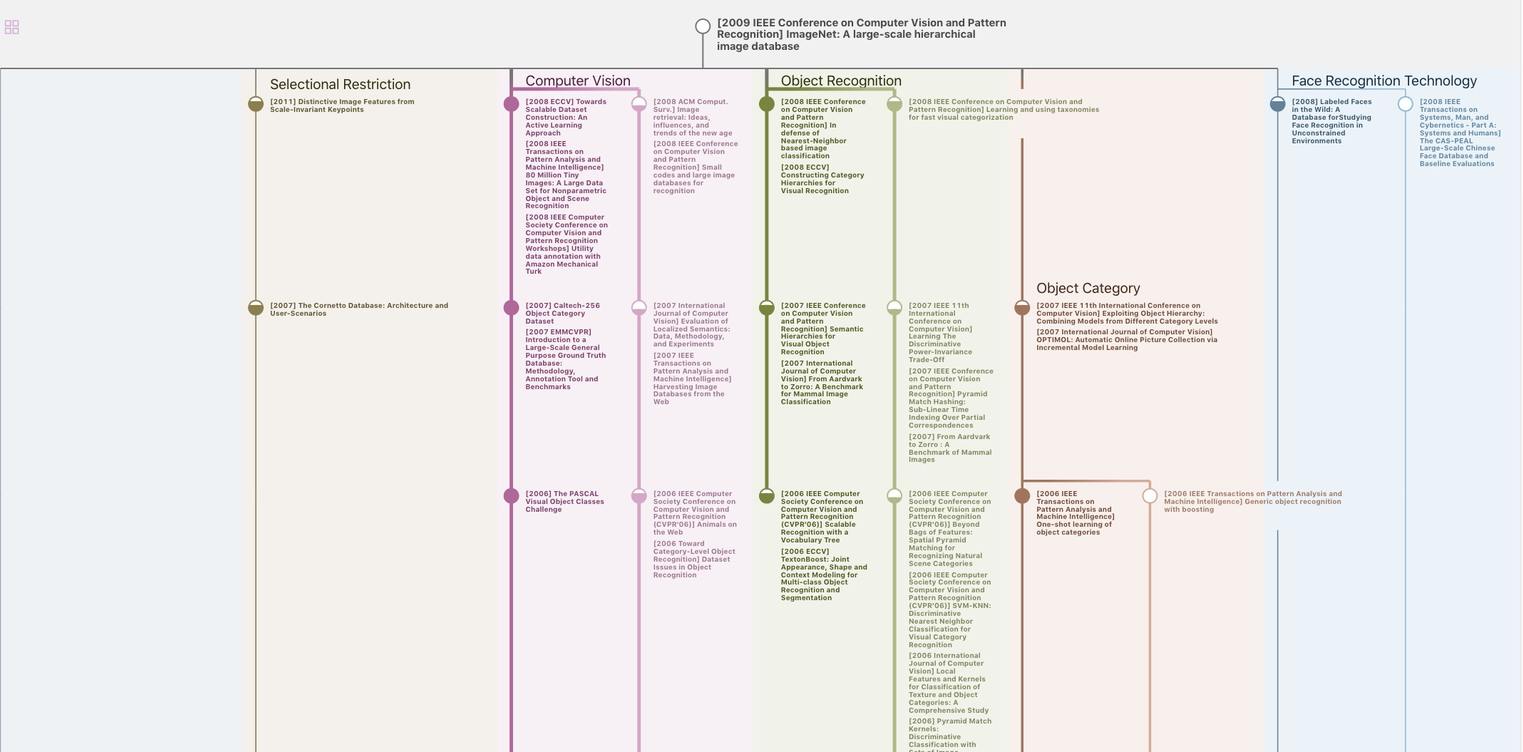
生成溯源树,研究论文发展脉络
Chat Paper
正在生成论文摘要