Guest Editorial Special Issue on Geometric Deep Learning in Medical Imaging
IEEE Transactions on Medical Imaging(2023)
摘要
In recent years, more and more attention has been devoted to geometric deep learning (GDL) and its applications to various problems in medical imaging. Unlike convolutional neural networks (CNNs) limited to 2-D/3-D grid-structured data, GDL can handle non-Euclidean data (i.e., graphs and manifolds) and is hence well-suited for medical imaging data such as structure-function connectivity networks, imaging genetics and omics, spatio-temporal anatomical representations, physics-informed GDL for optimal imaging sampling and acquisition, GDL in imaging inverse problems, etc. However, despite recent advances in GDL research, questions remain on how best to learn representations of non-Euclidean medical imaging data; how to convolve effectively on graphs; how to perform graph pooling/unpooling; how to handle heterogeneous data; and how to improve the interpretability of GDL. After discussing many other domain experts, we identify the need for a special issue that brings to the attention of the medical imaging community these interesting topics.
更多查看译文
关键词
geometric deep learning,deep learning,imaging
AI 理解论文
溯源树
样例
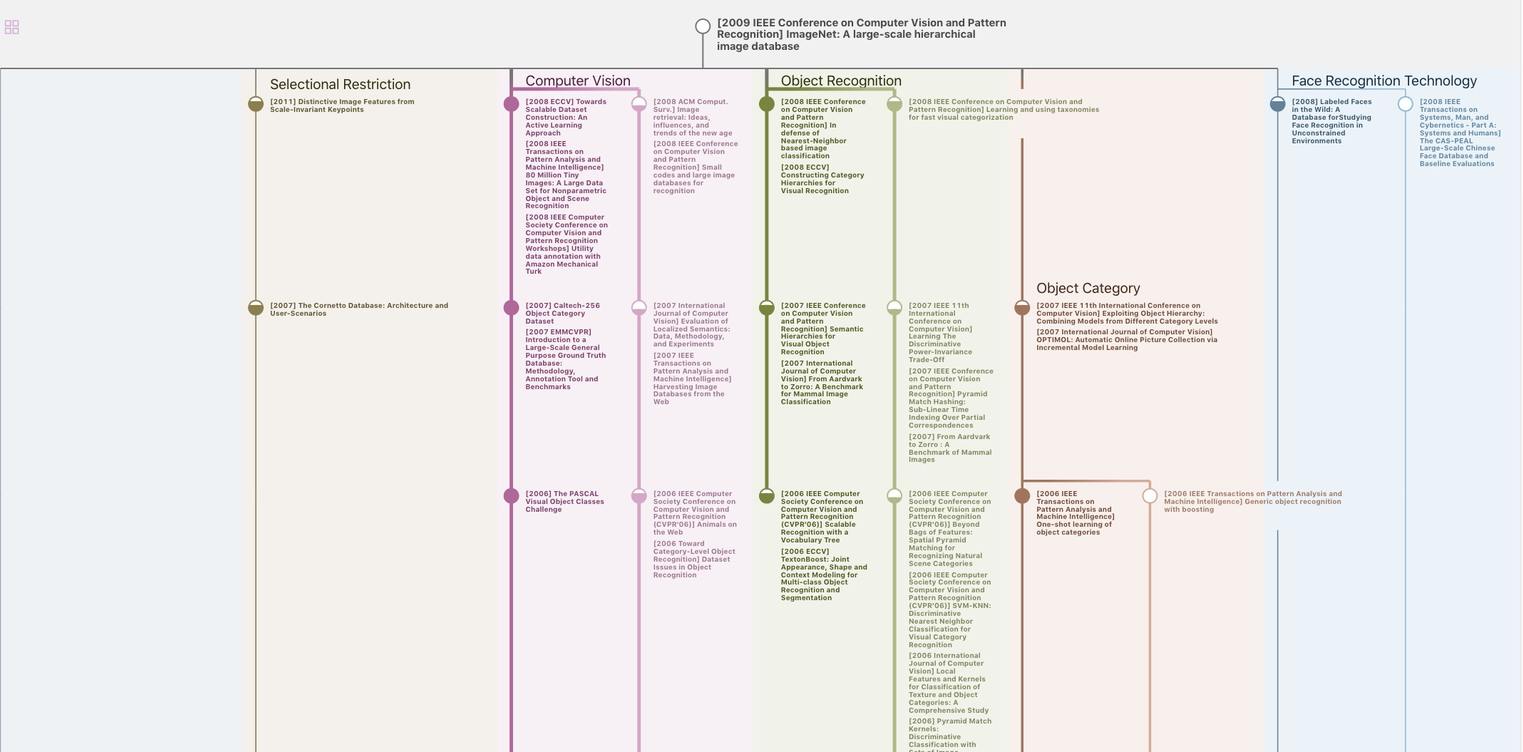
生成溯源树,研究论文发展脉络
Chat Paper
正在生成论文摘要