Spike Calibration: Bridging the Gap between ANNs and SNNs in ANN-SNN Conversion
ICLR 2023(2023)
摘要
Spiking Neural Networks (SNNs) have attracted great attention due to the distinctive characteristics of low power consumption and temporal information processing. ANN-SNN conversion, as the most commonly used method, can make converted SNNs achieve comparable performance as ANNs on large-scale datasets. However, the performance degrades severely under low time-steps, which hampers the practical applications of SNNs on neuromorphic chips. In this paper, instead of evaluating different conversion errors and then eliminating these errors, we define offset spike to measure the deviation degree of actual and desired firing rates of SNNs. We make a detailed analysis of offset spike and point out that the case of firing one more (or less) spike is the main reason for conversion error. Based on this, we propose an optimization strategy based on shifting initial membrane potential and theoretically prove the corresponding optimal shifting distance to calibrate the spike. In addition, we also note that our method has a unique iterative property to further reduce conversion error. The experimental results show that our proposed method achieves state-of-the-art performance on CIFAR-10, CIFAR-100, and ImageNet datasets. For example, we reach top-1 accuracy of 67.12% on ImageNet with 6 time-steps. To the best of our knowledge, this is the first time ANN-SNN conversion can simultaneously achieve high accuracy and ultra-low latency on the complex dataset.
更多查看译文
关键词
Spiking Neural Networks,Spike Calibration,Ultra-low-latency Conversion
AI 理解论文
溯源树
样例
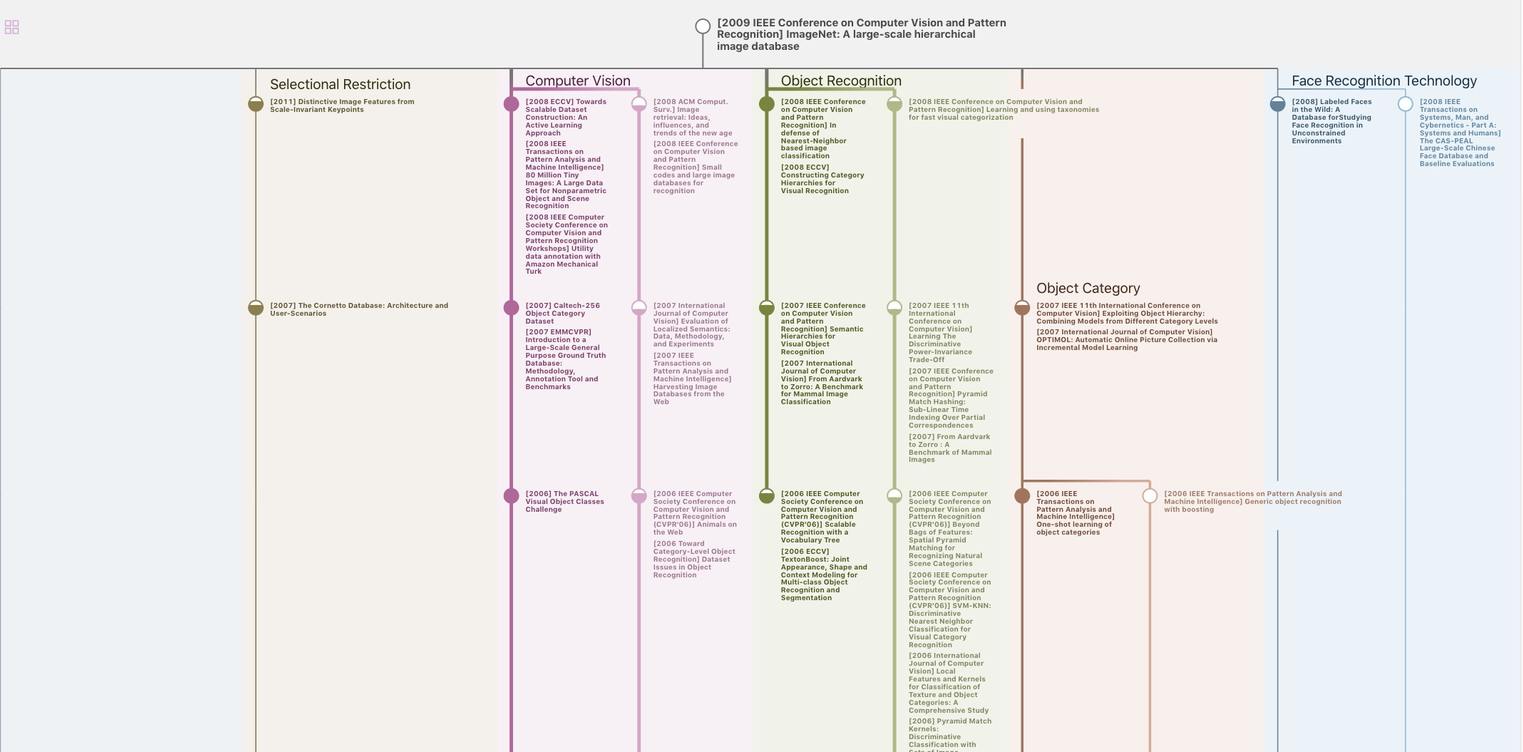
生成溯源树,研究论文发展脉络
Chat Paper
正在生成论文摘要