D4AM: A General Denoising Framework for Downstream Acoustic Models
ICLR 2023(2023)
摘要
The performance of acoustic models degrades notably in noisy environments. Speech enhancement (SE) can be used as a front-end strategy to serve automatic speech recognition (ASR) systems. However, the training objectives of existing SE approaches do not consider the generalization ability to unseen ASR systems. In this study, we propose a general denoising framework for various downstream acoustic models, called D4AM. Our framework fine-tunes the SE model with the backward gradient according to a specific acoustic model and the corresponding classification objective. At the same time, our method aims to take the regression objective as an auxiliary loss to make the SE model generalize to other unseen acoustic models. To jointly train an SE unit with regression and classification objectives, D4AM uses an adjustment scheme to directly estimate suitable weighting coefficients instead of going through a grid search process with additional training costs. The adjustment scheme consists of two parts: gradient calibration and regression objective weighting.
Experimental results show that D4AM can consistently and effectively provide improvements to various unseen acoustic models and outperforms other combination setups. To the best of our knowledge, this is the first work that deploys an effective combination scheme of regression (denoising) and classification (ASR) objectives to derive a general pre-processor applicable to various unseen ASR systems.
更多查看译文
关键词
audio processing,speech enhancement,robust automatic speech recognition,auxiliary task learning
AI 理解论文
溯源树
样例
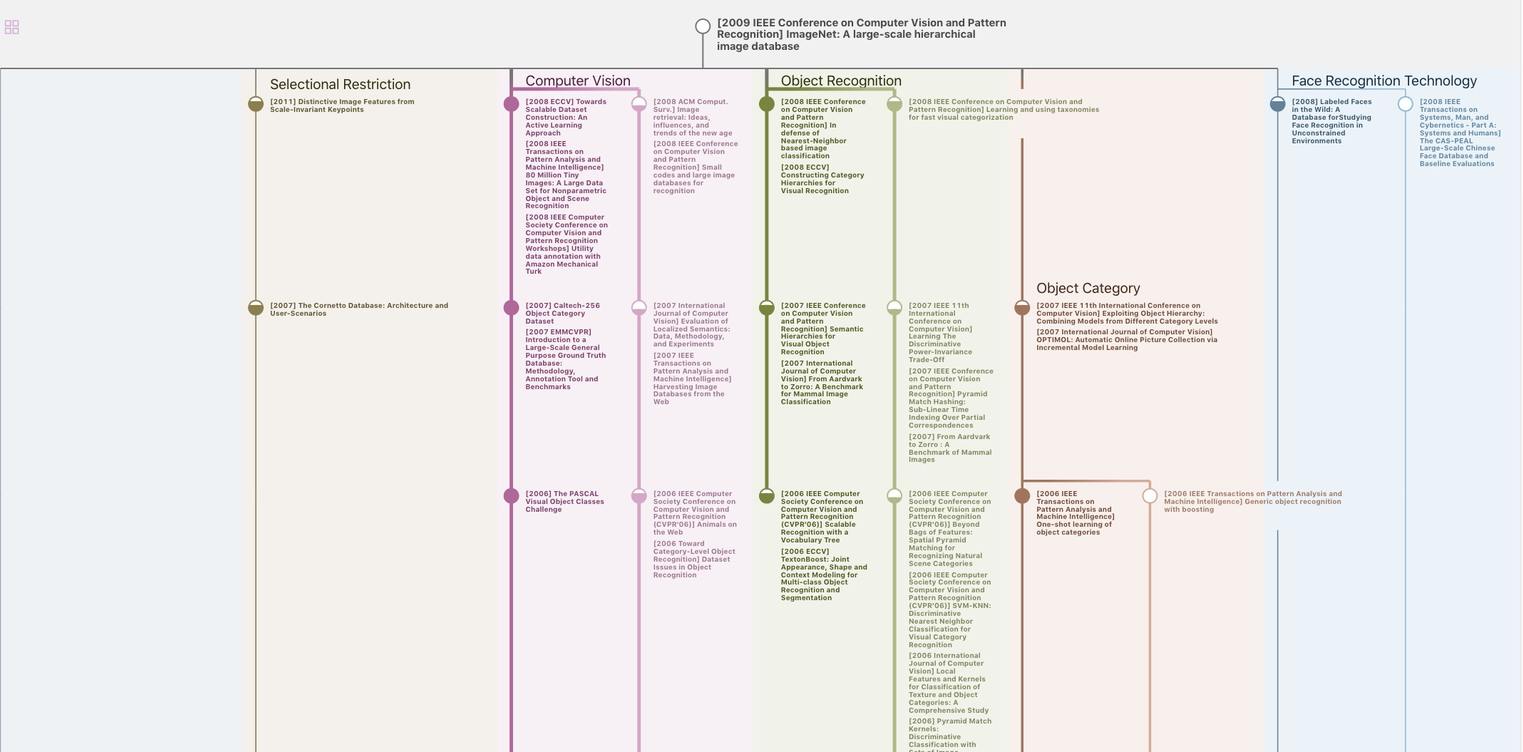
生成溯源树,研究论文发展脉络
Chat Paper
正在生成论文摘要