Robust Attention for Contextual Biased Visual Recognition
ICLR 2023(2023)
摘要
Visual attention does not always capture the essential object representation desired for robust predictions. Attention modules tend to underline not only the target object but also the common co-occurring context that the module thinks helpful in the training. The problem is rooted in the confounding effect of the context leading to incorrect causalities between objects and predictions, which is further exacerbated by visual attention. In this paper, to learn causal object features robust for contextual bias, we propose a novel attention module named Interventional Dual Attention (IDA) for visual recognition. Specifically, IDA adopts two attention layers with multiple sampling intervention, which protects the attention against the confounder context. Note that our method is model-agnostic and thus can be implemented on various backbones. Extensive experiments show our model obtains significant improvements in classification and detection with lower computation. In particular, we achieve the state-of-the-art results in multi-label classification on MS-COCO and PASCAL-VOC. The codes will be publicly available.
更多查看译文
关键词
Causal Inference,Object Recognition,Attention Mechanism,Confounding Context,Interventional Dual Attention
AI 理解论文
溯源树
样例
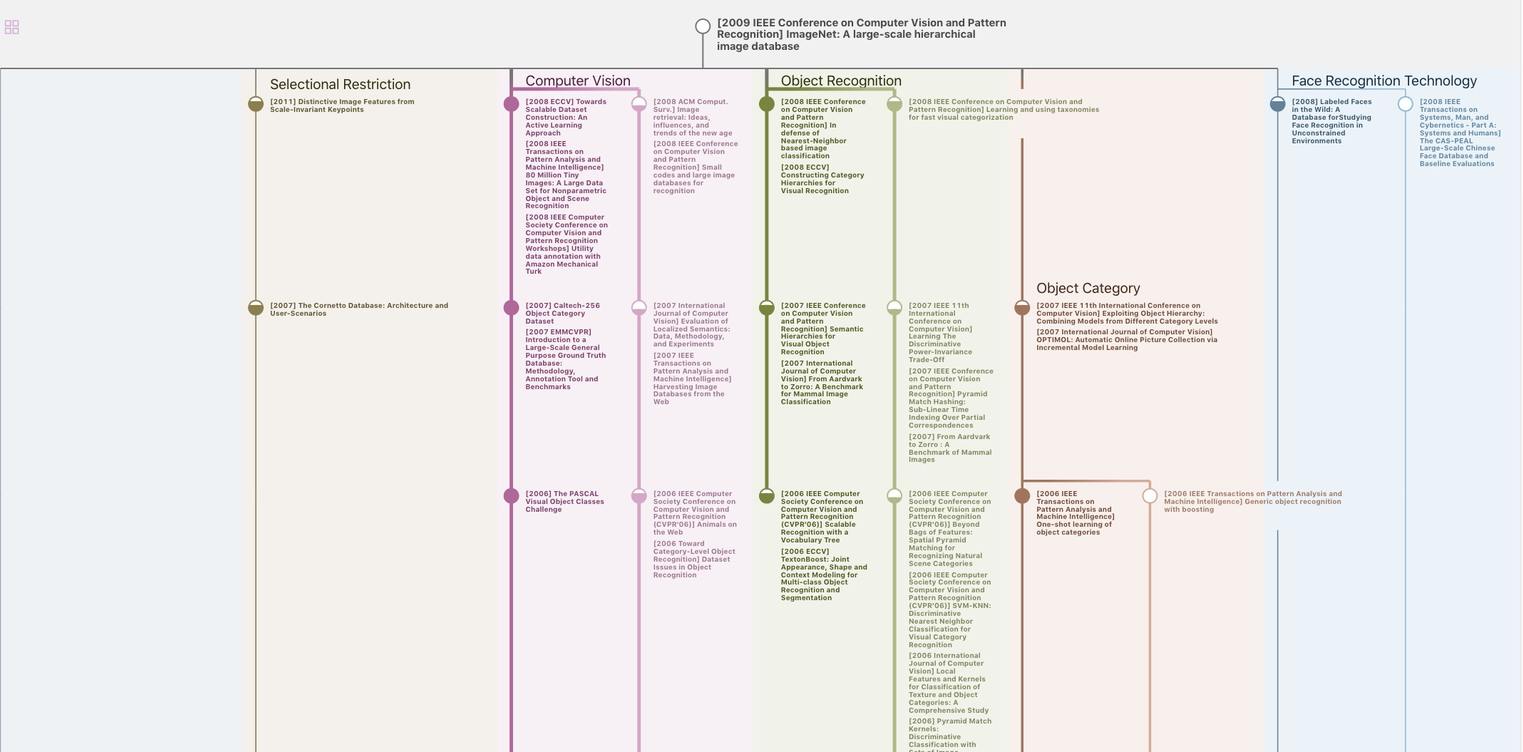
生成溯源树,研究论文发展脉络
Chat Paper
正在生成论文摘要