Average Sensitivity of Decision Tree Learning
ICLR 2023(2023)
摘要
A decision tree is a fundamental model used in data mining and machine learning. In practice, the training data used to construct a decision tree may change over time or contain noise. A drastic change in the learned tree structure owing to such data perturbation is unfavorable in practice. For example, in data mining, a change in the tree implies that the extracted knowledge can be unstable, which raises the question of whether the extracted knowledge is truly reliable or is only a noisy artifact. To alleviate this issue, we design decision tree learning algorithms that are stable against insignificant perturbations in the training data. Specifically, we adopt the notion of average sensitivity as a stability measure, and design an algorithm with low average sensitivity that outputs a decision tree whose accuracy is nearly equal to the optimal decision tree. The experimental results on real-world datasets demonstrate that the proposed algorithm achieves a low average sensitivity with an insignificant decrease in accuracy.
更多查看译文
关键词
decision tree,average sensitivity,trustworthy machine learning
AI 理解论文
溯源树
样例
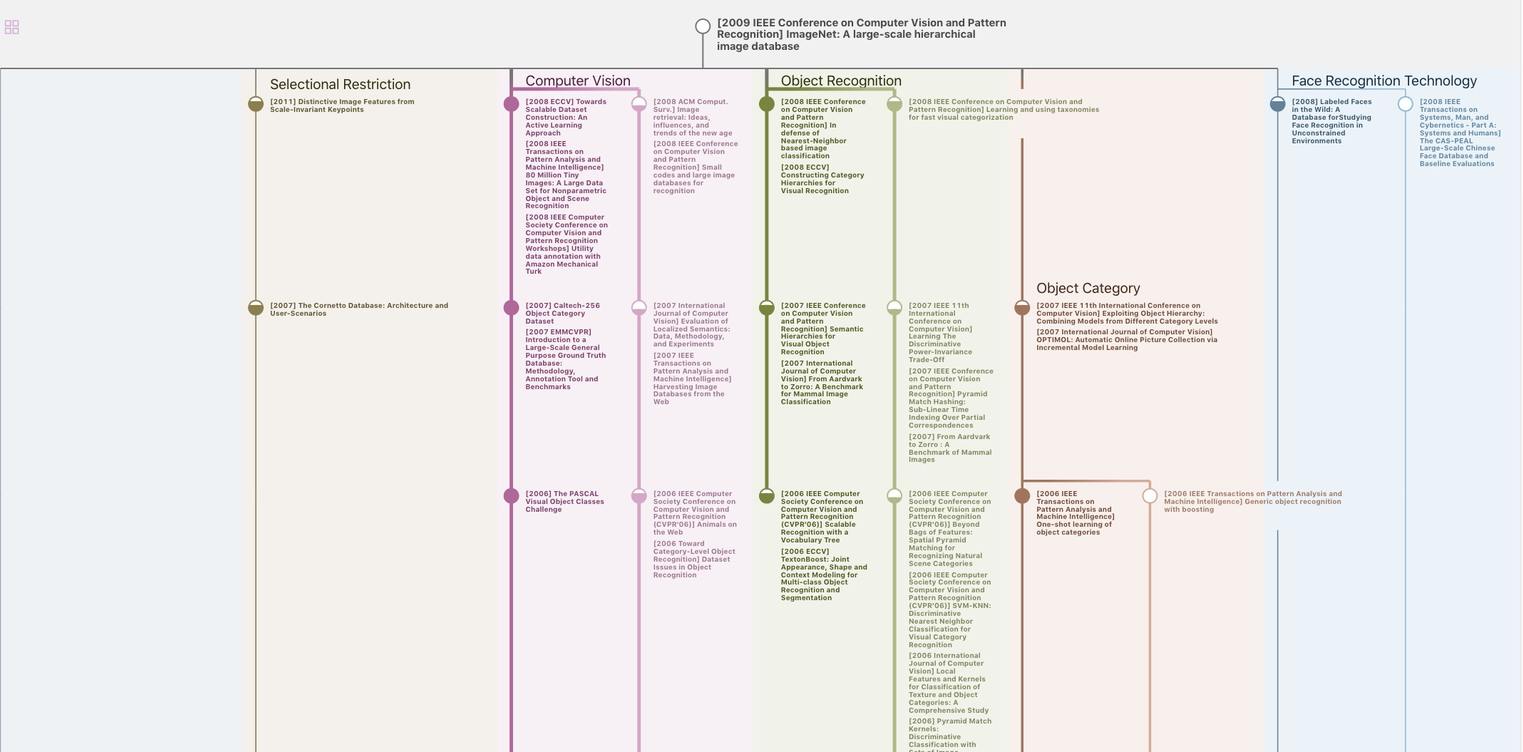
生成溯源树,研究论文发展脉络
Chat Paper
正在生成论文摘要