RGI: robust GAN-inversion for mask-free image inpainting and unsupervised pixel-wise anomaly detection
ICLR 2023(2023)
摘要
Generative adversarial networks (GANs), trained on a large-scale image dataset, can be a good approximator of the natural image manifold. GAN-inversion, using a pre-trained generator as a deep generative prior, is a promising tool for image restoration under corruptions. However, the performance of GAN-inversion can be limited by a lack of robustness to unknown gross corruptions, i.e., the restored image might easily deviate from the ground truth. In this paper, we propose a Robust GAN-inversion (RGI) method with a provable robustness guarantee to achieve image restoration under unknown \textit{gross} corruptions, where a small fraction of pixels are completely corrupted. Under mild assumptions, we show that the restored image and the identified corrupted region mask converge asymptotically to the ground truth. Moreover, we extend RGI to Relaxed-RGI (R-RGI) for generator fine-tuning to mitigate the gap between the GAN learned manifold and the true image manifold while avoiding trivial overfitting to the corrupted input image, which further improves the image restoration and corrupted region mask identification performance. The proposed RGI/R-RGI method unifies two important applications with state-of-the-art (SOTA) performance: (i) mask-free semantic inpainting, where the corruptions are unknown missing regions, the restored background can be used to restore the missing content. (ii) unsupervised pixel-wise anomaly detection, where the corruptions are unknown anomalous regions, the retrieved mask can be used as the anomalous region’s segmentation mask.
更多查看译文
关键词
Robust GAN-inversion,Mask-free Semantic Inpainting,Unsupervised Pixel-wise Anomaly Detection
AI 理解论文
溯源树
样例
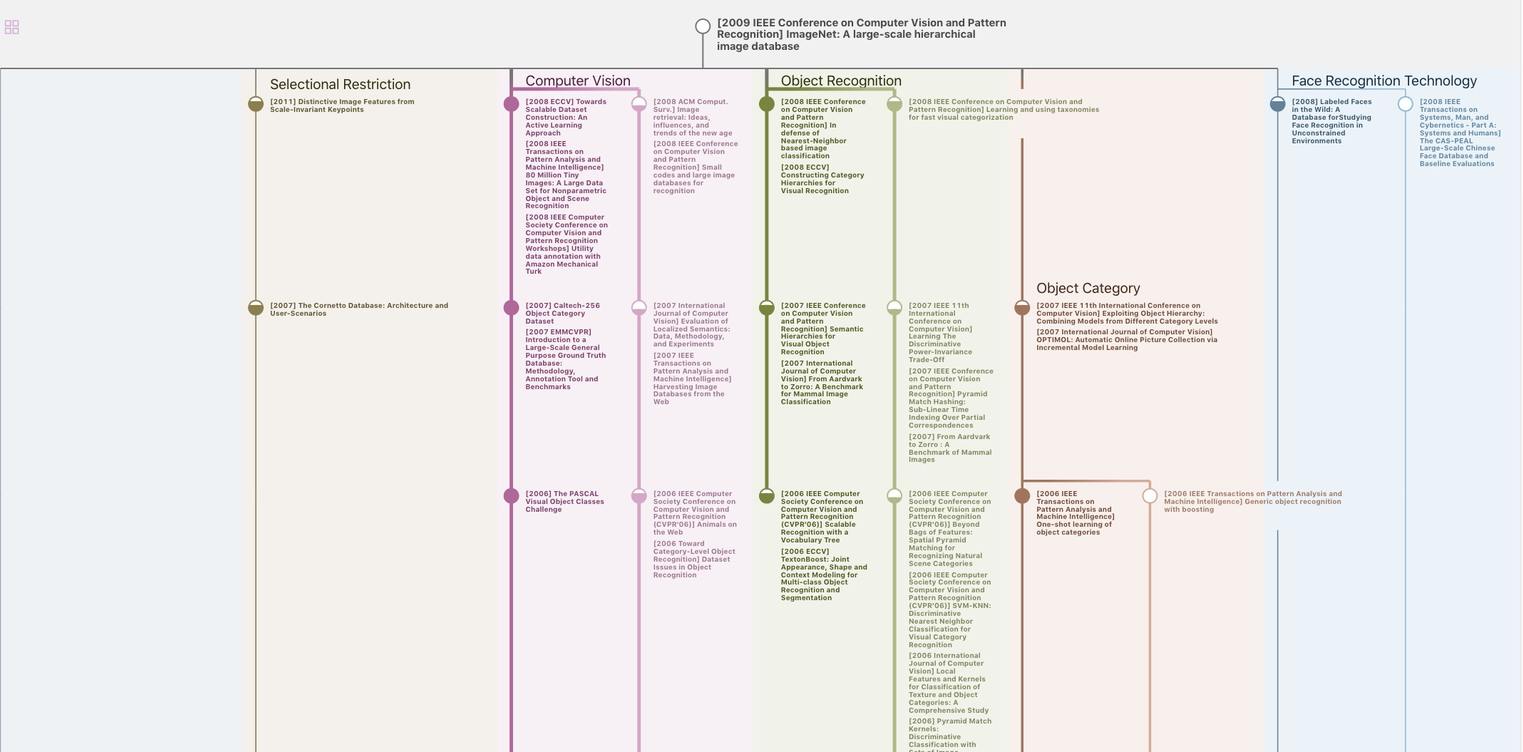
生成溯源树,研究论文发展脉络
Chat Paper
正在生成论文摘要