Learned Index with Dynamic $\epsilon$
ICLR 2023(2023)
摘要
Index structure is a fundamental component in database and facilitates broad data retrieval applications. Recent learned index methods show superior performance by learning hidden yet useful data distribution with the help of machine learning, and provide a guarantee that the prediction error is no more than a pre-defined $\epsilon$. However, existing learned index methods adopt a fixed $\epsilon$ for all the learned segments, neglecting the diverse characteristics of different data localities. In this paper, we propose a mathematically-grounded learned index framework with dynamic $\epsilon$, which is efficient and pluggable to existing learned index methods. We theoretically analyze prediction error bounds that link $\epsilon$ with data characteristics for an illustrative learned index method. Under the guidance of the derived bounds, we learn how to vary $\epsilon$ and improve the index performance with a better space-time trade-off. Experiments with real-world datasets and several state-of-the-art methods demonstrate the efficiency, effectiveness and usability of the proposed framework.
更多查看译文
关键词
Learned Index,Dynamic $\epsilon$
AI 理解论文
溯源树
样例
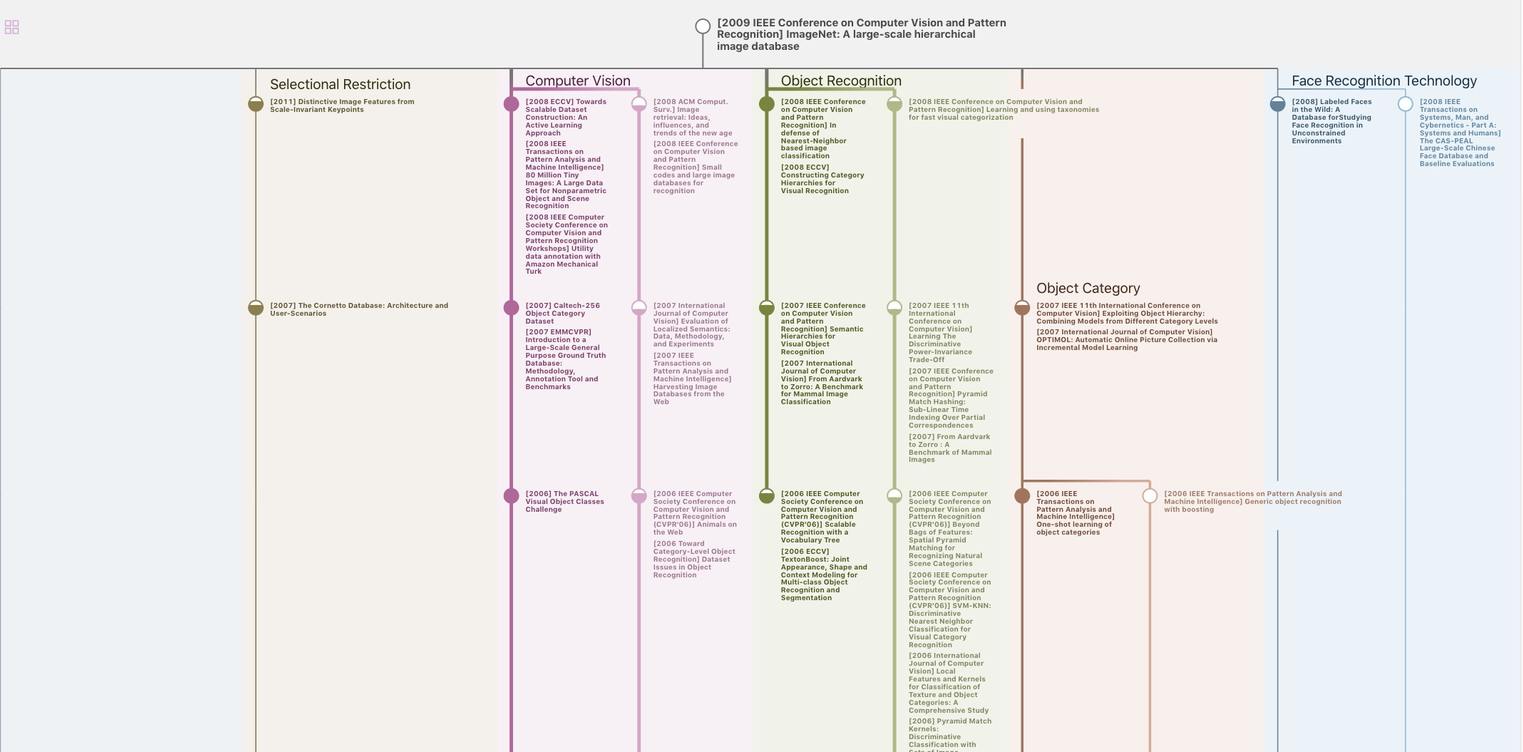
生成溯源树,研究论文发展脉络
Chat Paper
正在生成论文摘要