Empirical Study of Pre-training a Backbone for 3D Human Pose and Shape Estimation
ICLR 2023(2023)
摘要
We empirically study unexplored, yet must-know baselines of pre-training a backbone for 3D human pose and shape estimation (3DHPSE). Recently, a few self-supervised representation learning (SSL) methods have been reported to outperform the ImageNet classification pre-training for vision tasks such as object detection. However, its effects on 3DHPSE are open to question, whose target is fixed to a single class, the human. In this regard, we inspect the effectiveness of SSL on 3DHPSE and investigate two other pre-training approaches that have received relatively less attention. They are 2D annotation-based pre-training and synthetic data pre-training. Similar to the motivation of SSL to benefit from unlabeled data, they have potential advantages to exploit data with less data collection cost compared with real 3D data. SSL methods underperform the conventional ImageNet classification pre-training on multiple 3DHPSE benchmarks by 7.7% on average. In contrast, despite a much less amount of pre-training data, the 2D annotation-based pre-training improves accuracy on all benchmarks and shows faster convergence during fine-tuning. In the semi-supervised setting, the improvement increases up to 8.2%, while SSL decreases accuracy by 10.7%, and synthetic data pre-training shows 0.2% decreased accuracy compared with the classification pre-training. Our observations would make the community carefully think about the current SSL-based pre-training trend for 3DHPSE and diversify research on pre-training approaches.
更多查看译文
关键词
pre-training,3D human pose and shape estimation,self-supervised representation learning
AI 理解论文
溯源树
样例
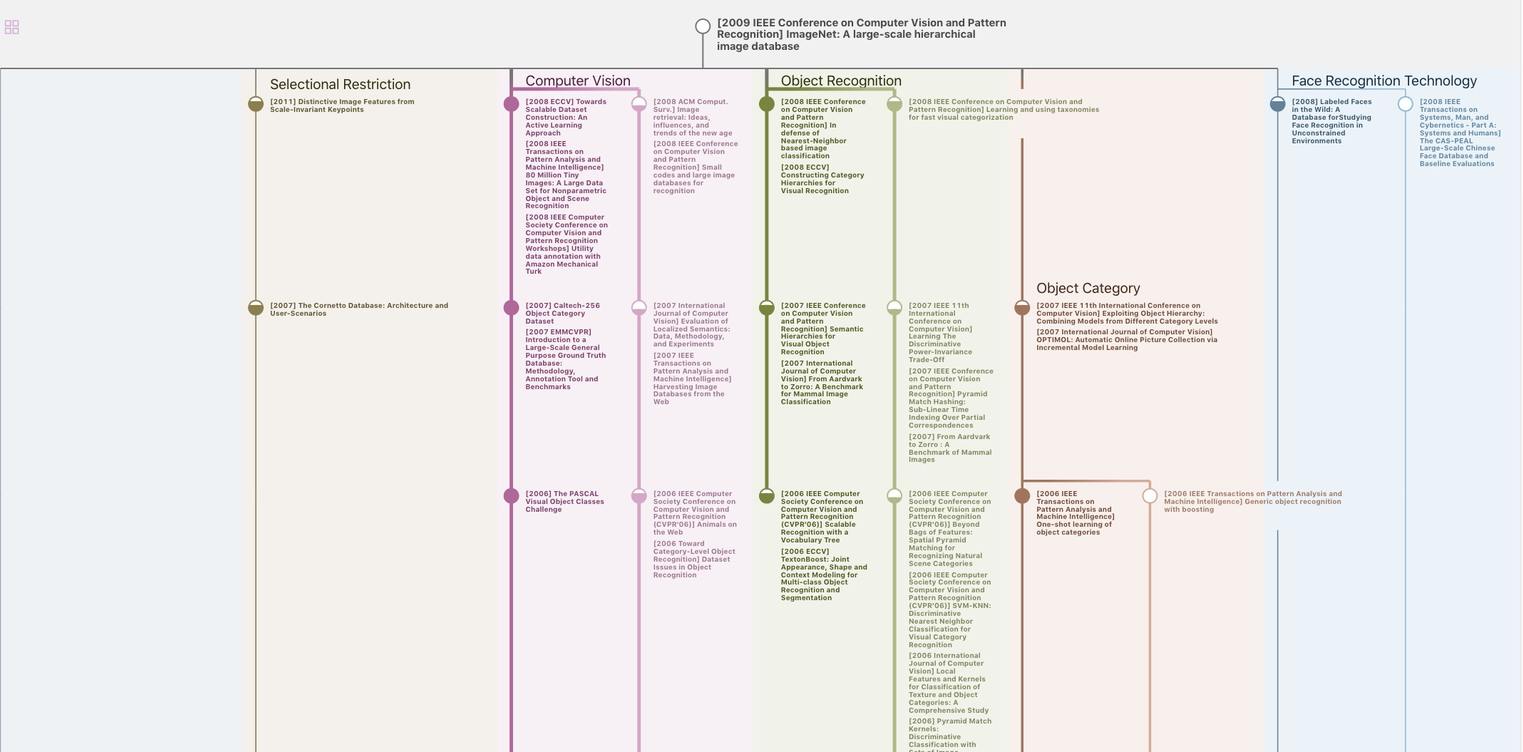
生成溯源树,研究论文发展脉络
Chat Paper
正在生成论文摘要