On the Edge of Benign Overfitting: Label Noise and Overparameterization Level
ICLR 2023(2023)
摘要
Studies on benign overfitting provide insights for the success of overparameterized deep learning models. In this work, we examine whether overfitting is truly benign in real-world classification tasks. We start with the observation that a ResNet model overfits benignly on Cifar10 but not benignly on ImageNet. To understand why benign overfitting fails in the ImageNet experiment, we theoretically analyze benign overfitting under a more restrictive setup where the number of parameters is not significantly larger than the number of data points. Under this mild overparameterization setup, our analysis identifies a phase change: unlike in the previous heavy overparameterization settings, benign overfitting can now fail in the presence of label noise. Our analysis explains our empirical observations, and is validated by a set of control experiments with ResNets. Our work highlights the importance of understanding implicit bias in underfitting regimes as a future direction.
更多查看译文
关键词
generalization,benign overfitting,mild overparameterization,implicit bias
AI 理解论文
溯源树
样例
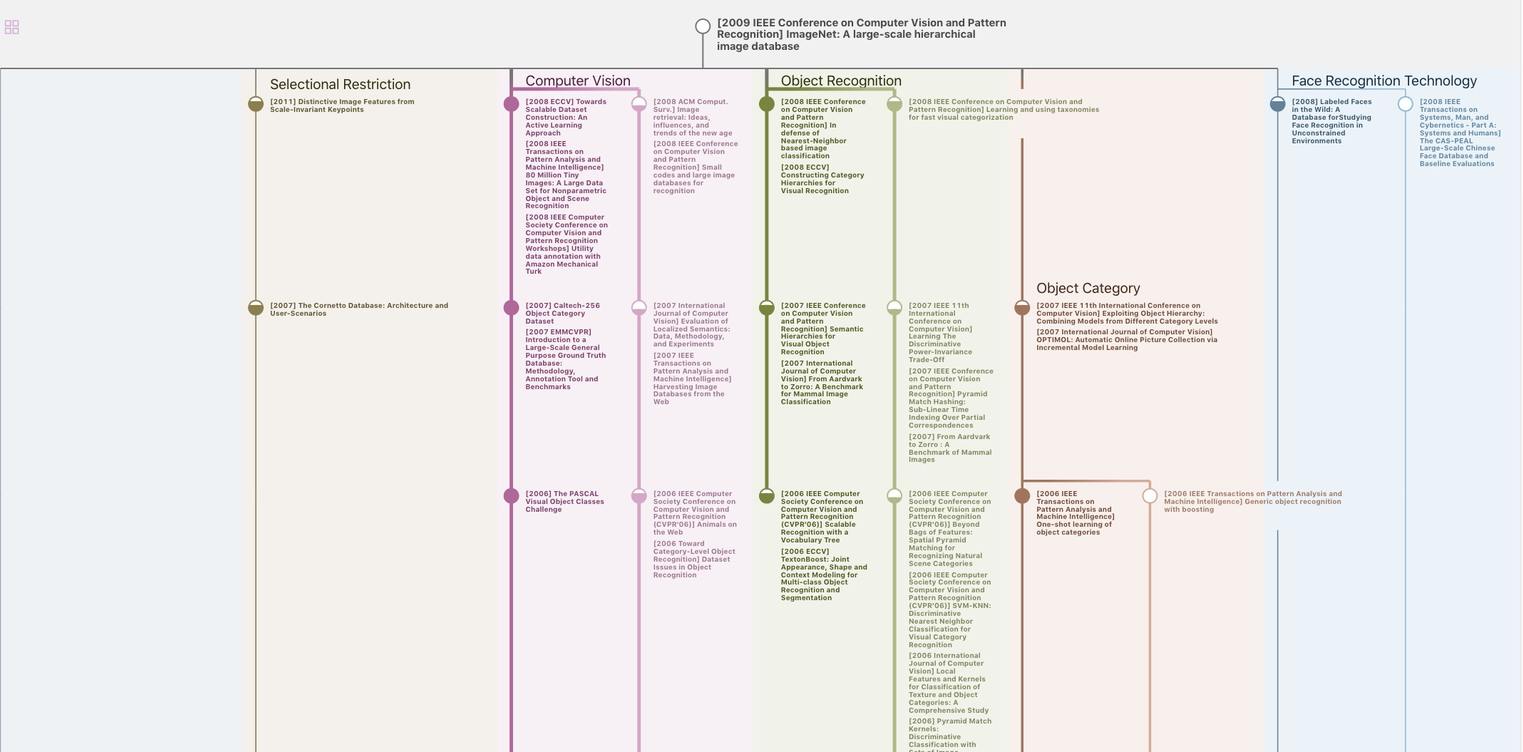
生成溯源树,研究论文发展脉络
Chat Paper
正在生成论文摘要