Sharper Bounds for Uniformly Stable Algorithms with Stationary Mixing Process
ICLR 2023(2023)
摘要
Generalization analysis of learning algorithms often builds on a critical assumption that training examples are independently and identically distributed, which is often violated in practical problems such as time series prediction. In this paper, we use algorithmic stability to study the generalization performance of learning algorithms with $\psi$-mixing data, where the dependency between observations weakens over time. We show uniformly stable algorithms guarantee high-probability generalization bounds of the order $O(1/\sqrt{n})$ (within a logarithmic factor), where $n$ is the sample size. We apply our general result to specific algorithms including regularization schemes, stochastic gradient descent and localized iterative regularization, and develop excess population risk bounds for learning with $\psi$-mixing data. Our analysis builds on a novel moment bound for weakly-dependent random variables on a $\varphi$-mixing sequence and a novel error decomposition of generalization error.
更多查看译文
关键词
Algorithmic Stability,Non-I.I.D. Learning,Generalization Error,Learning Theory
AI 理解论文
溯源树
样例
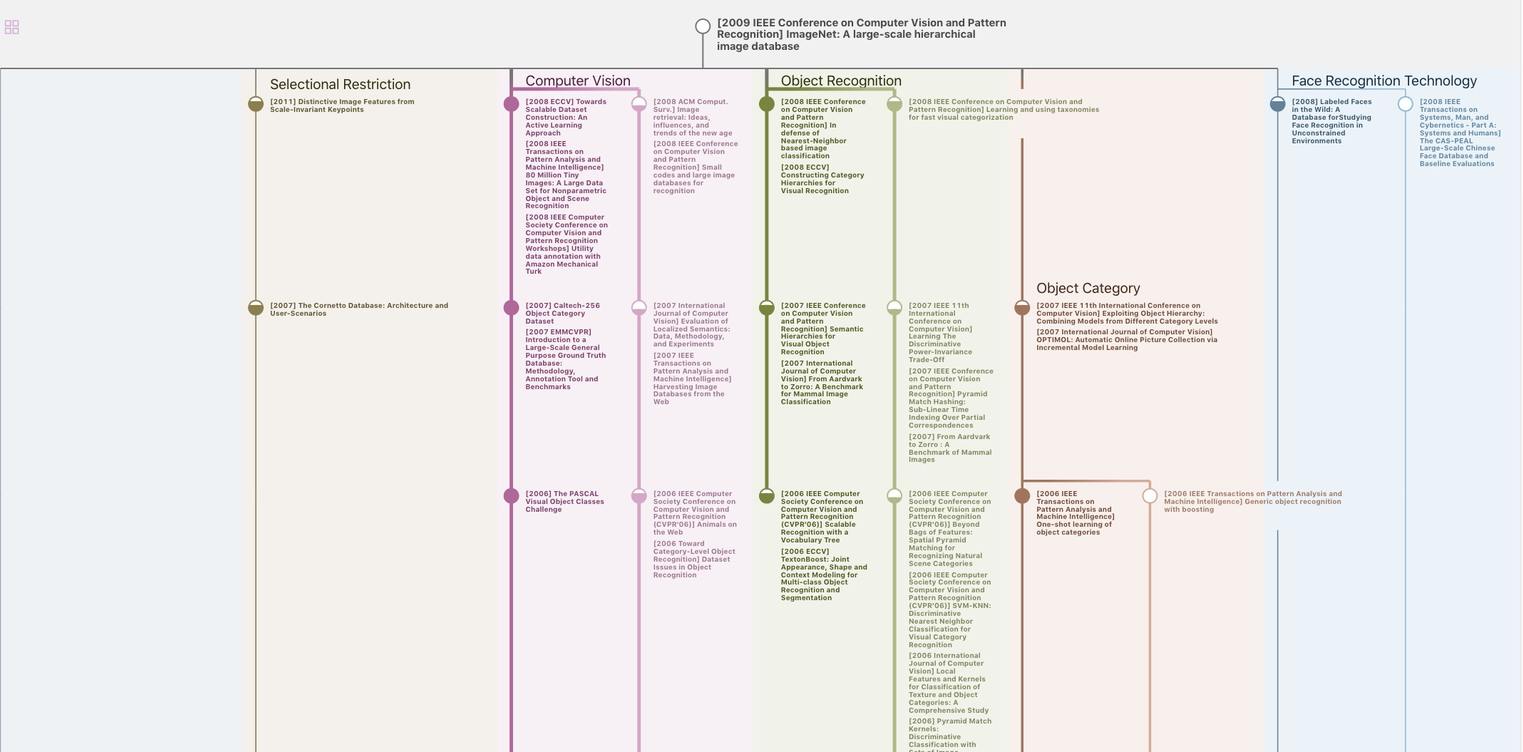
生成溯源树,研究论文发展脉络
Chat Paper
正在生成论文摘要