Pushing the Accuracy-Fairness Tradeoff Frontier with Introspective Self-play
ICLR 2023(2023)
摘要
Improving the accuracy-fairness frontier of deep neural network (DNN) models is an important problem. Uncertainty-based active learning (AL) can potentially improve the frontier by preferentially sampling underrepresented subgroups to create a more balanced training dataset. However, the quality of uncertainty estimates from modern DNNs tend to degrade in the presence of spurious correlations and dataset bias, compromising the effectiveness of AL for sampling tail groups. In this work, we propose $Introspective Self-play$ (ISP), a simple approach to improve the uncertainty estimation of a deep neural network under dataset bias, by adding an auxiliary $introspection$ task requiring a model to predict the bias for each data point in addition to the label. We show that ISP provably improves the bias-awareness of the model representation and the resulting uncertainty estimates. On two real-world tabular and language tasks,ISP serves as a simple “plug-in” for AL model training, consistently improving both the tail-group sampling rate and the final accuracy-fairness trade-off frontier of popular AL methods.
更多查看译文
关键词
Uncertainty Quantification,Spurious Correlation,Active Learning
AI 理解论文
溯源树
样例
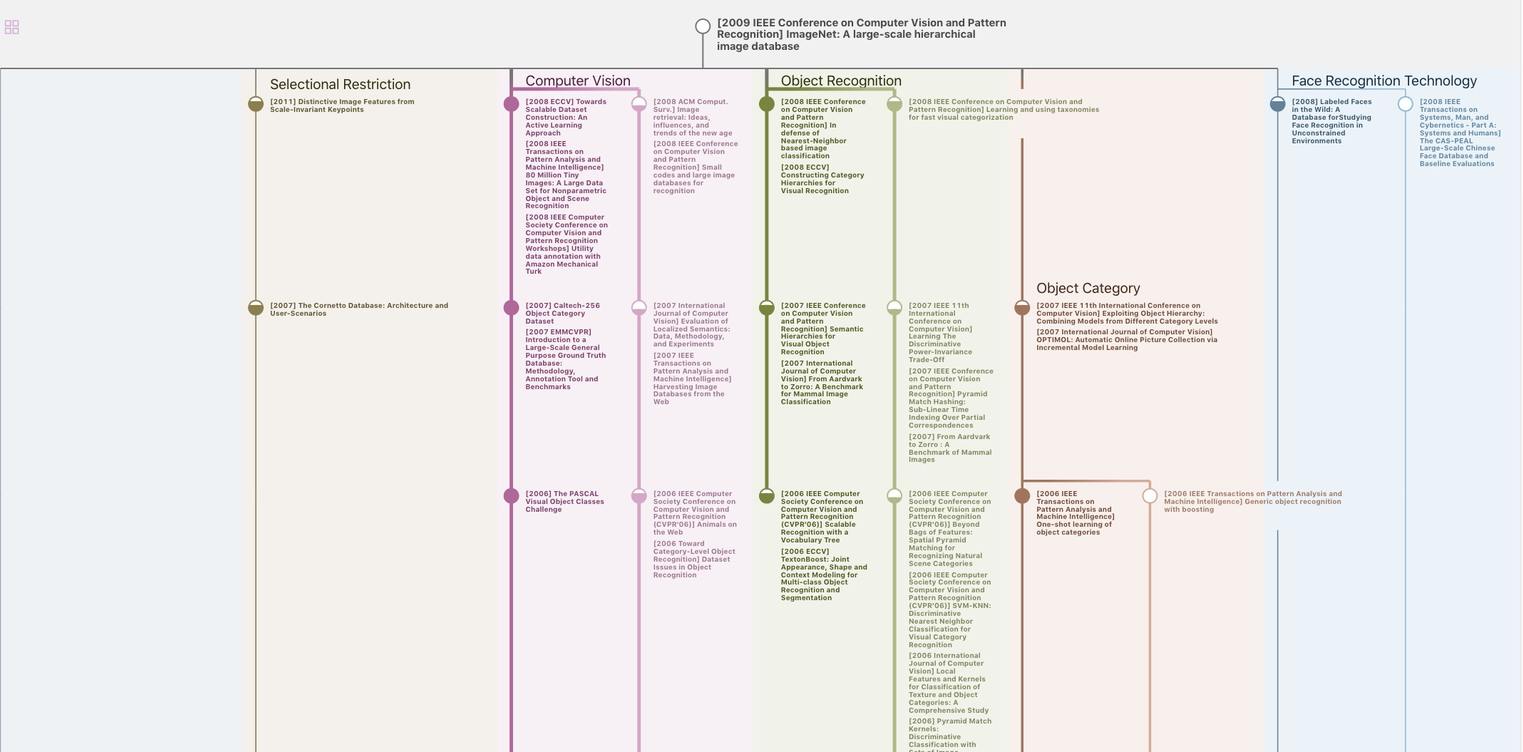
生成溯源树,研究论文发展脉络
Chat Paper
正在生成论文摘要