MAESTRO: Open-Ended Environment Design for Multi-Agent Reinforcement Learning
ICLR 2023(2023)
摘要
Open-ended learning methods that automatically generate a curriculum of increasingly challenging tasks serve as a promising avenue toward generally capable reinforcement learning (RL) agents. Existing methods adapt curricula independently over either environment parameters (in single-agent settings) or co-player policies (in multi-agent settings). However, the strengths and weaknesses of co-players can manifest themselves differently depending on environmental features. It is thus crucial to consider the dependency between the environment and co-player when shaping a curriculum in multi-agent domains. In this work, we use this insight and extend Unsupervised Environment Design (UED) to multi-agent environments. We then introduce Multi-Agent Environment-Space Response Oracles (MAESTRO), the first multi-agent UED approach for two-player zero-sum settings. MAESTRO efficiently produces adversarial, joint curricula over both environment parameters and co-player policies and attains minimax-regret guarantees at Nash equilibrium. Our experiments show that MAESTRO outperforms a number of strong baselines on competitive two-player environments, spanning discrete and continuous control.
更多查看译文
关键词
reinforcement learning,multi-agent learning,unsupervised environment design
AI 理解论文
溯源树
样例
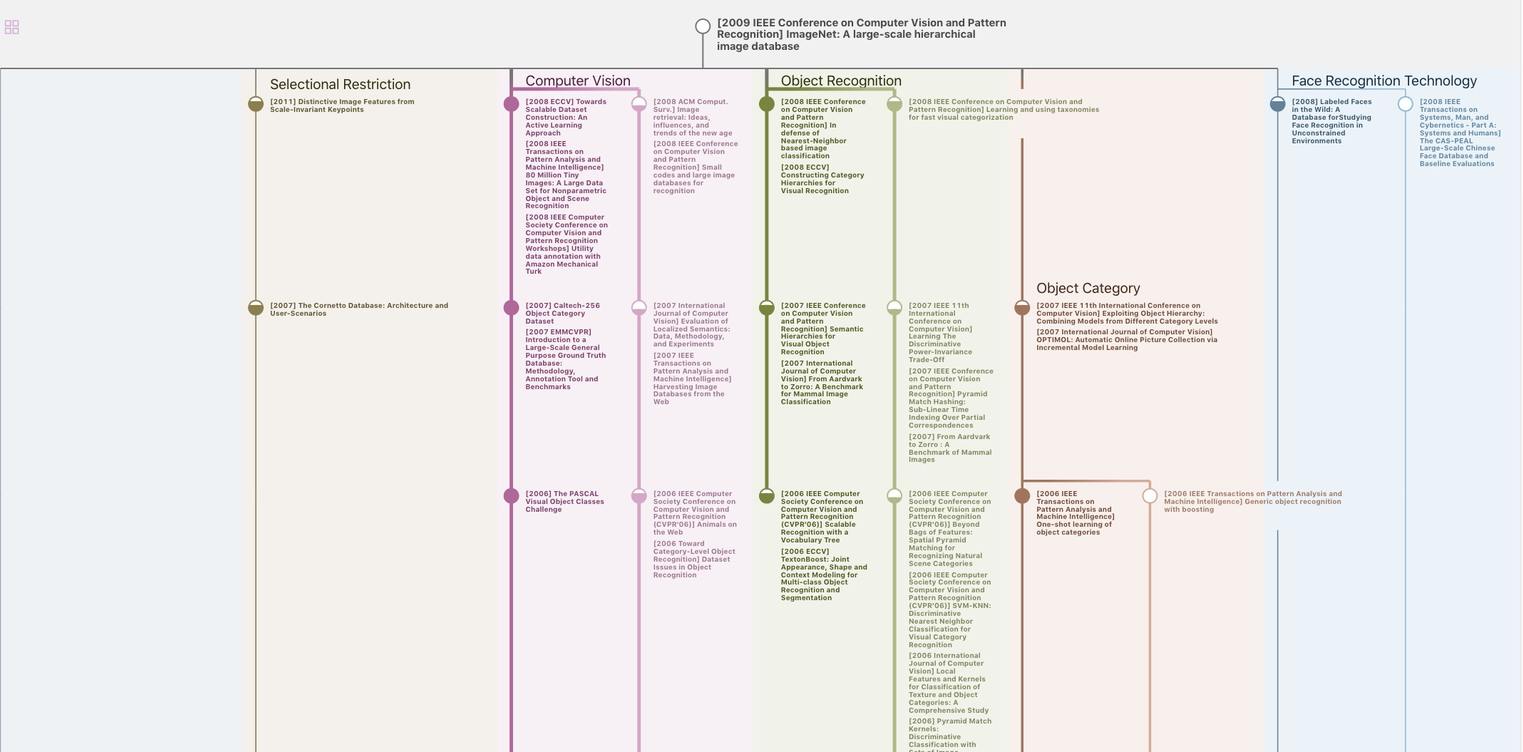
生成溯源树,研究论文发展脉络
Chat Paper
正在生成论文摘要