SLTUNET: A Simple Unified Model for Sign Language Translation
ICLR 2023(2023)
摘要
Despite recent successes with neural models for sign language translation (SLT), translation quality still lags behind spoken languages because of the data scarcity and modality gap between sign video and text. To address both problems, we investigate strategies for cross-modality representation sharing for SLT. We propose SLTUNET, a simple unified neural model designed to support multiple SLTrelated tasks jointly, such as sign-to-gloss, gloss-to-text and sign-to-text translation. Jointly modeling different tasks endows SLTUNET with the capability to explore the cross-task relatedness that could help narrow the modality gap. In addition, this allows us to leverage the knowledge from external resources, such as abundant parallel data used for spoken-language machine translation (MT). We show in experiments that SLTUNET achieves competitive and even state-of-the-art performance on PHOENIX-2014T and CSL-Daily when augmented with MT data and equipped with a set of optimization techniques. We further use the DGS Corpus for end-to-end SLT for the first time. It covers broader domains with a significantly larger vocabulary, which is more challenging and which we consider to allow for a more realistic assessment of the current state of SLT than the former two. Still, SLTUNET obtains improved results on the DGS Corpus. Code will be released.
更多查看译文
关键词
Unified Modeling,Multi-task Learning,Sign Language Translation,Cross-modality Learning
AI 理解论文
溯源树
样例
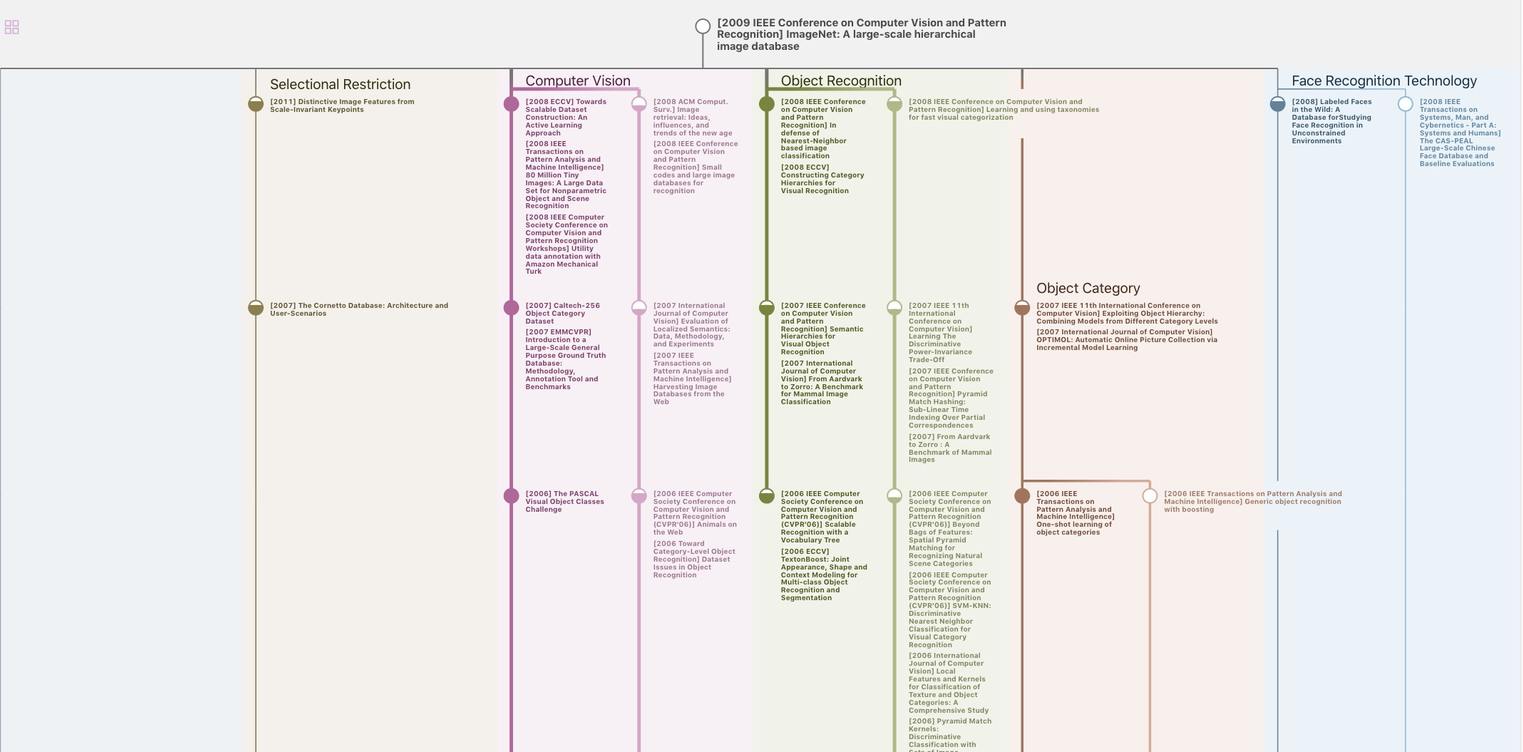
生成溯源树,研究论文发展脉络
Chat Paper
正在生成论文摘要