Scaffolding a Student to Instill Knowledge
ICLR 2023(2023)
摘要
We propose a novel knowledge distillation (KD) method to selectively instill teacher knowledge into a student model motivated by situations where the student's capacity is significantly smaller than that of the teachers. In vanilla KD, the teacher primarily sets a predictive target for the student to follow, and we posit that this target is overly optimistic due to the student's lack of capacity. We develop a novel scaffolding scheme where the teacher, in addition to setting a predictive target, also scaffolds the student's prediction by censoring hard-to-learn examples. Scaffolding utilizes the same information as the teacher's soft-max predictions as inputs, and in this sense, our proposal can be viewed as a natural variant of vanilla KD. We show on synthetic examples that censoring hard-examples leads to smoothening the student's loss landscape so that the student encounters fewer local minima. As a result, it has good generalization properties. Against vanilla KD, we achieve improved performance and are comparable to more intrusive techniques that leverage feature matching on benchmark datasets.
更多查看译文
关键词
knowledge distillation,tiny capacity student,large capacity teacher,budget constrained learning
AI 理解论文
溯源树
样例
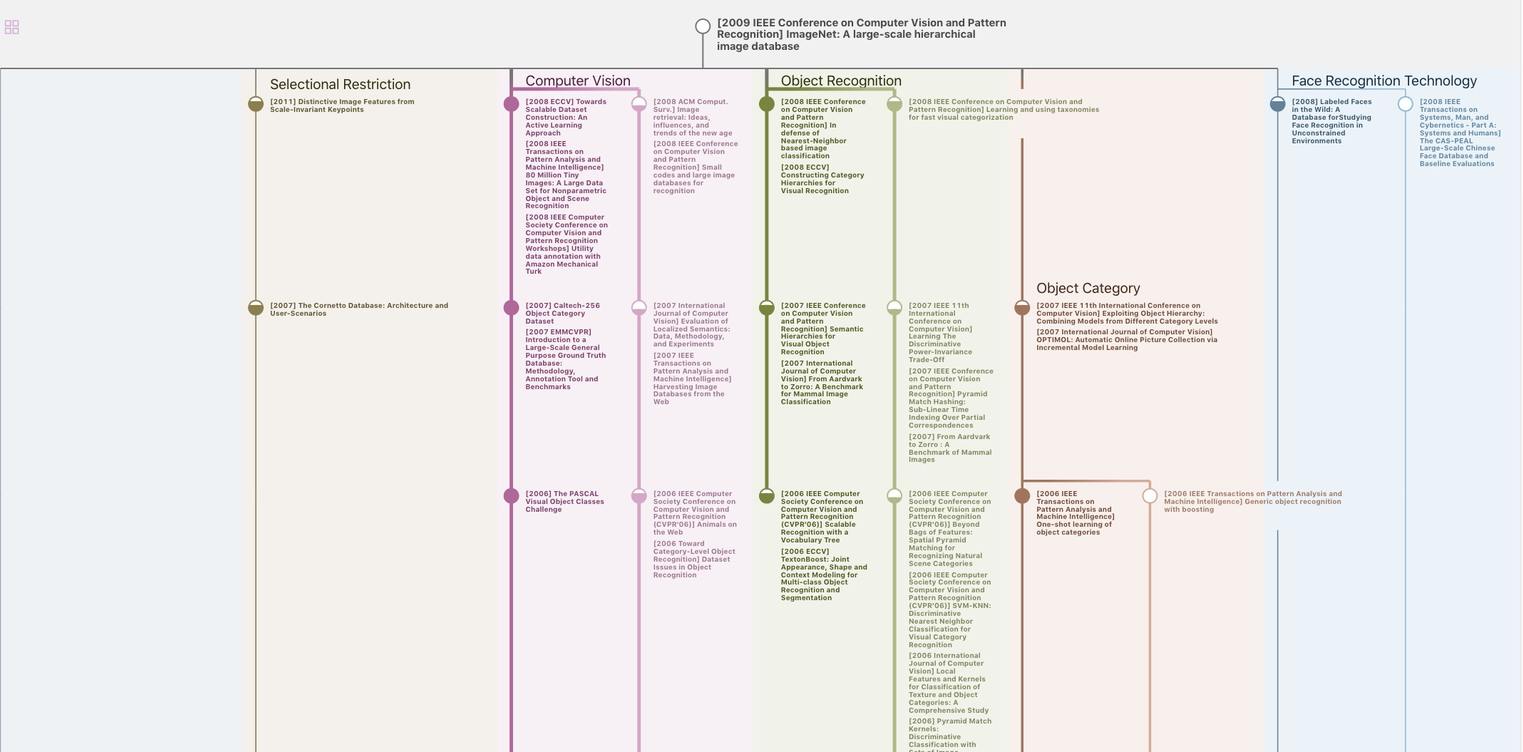
生成溯源树,研究论文发展脉络
Chat Paper
正在生成论文摘要