On Explaining Neural Network Robustness with Activation Path
ICLR 2023(2023)
摘要
Despite their verified performance, neural networks are prone to be misled by maliciously designed adversarial examples. This work investigates the robustness of neural networks from the activation pattern perspective. We find that despite the complex structure of the deep neural network, most of the neurons provide locally stable contributions to the output, while the minority, which we refer to as float neurons, can greatly affect the prediction. We decompose the computational graph of the neural network into the fixed path and float path and investigate their role in generating adversarial examples. Based on our analysis, we categorize the vulnerable examples into Lipschitz vulnerability and float neuron vulnerability. We show that the boost of robust accuracy from randomized smoothing is the result of correcting the latter. We then propose an SC-RFP (smoothed classifier with repressed float path) to further reduce the instability of the float neurons and show that our result can provide a higher certified radius as well as accuracy.
更多查看译文
关键词
Randomized Smoothing,Robustness,Neural Network
AI 理解论文
溯源树
样例
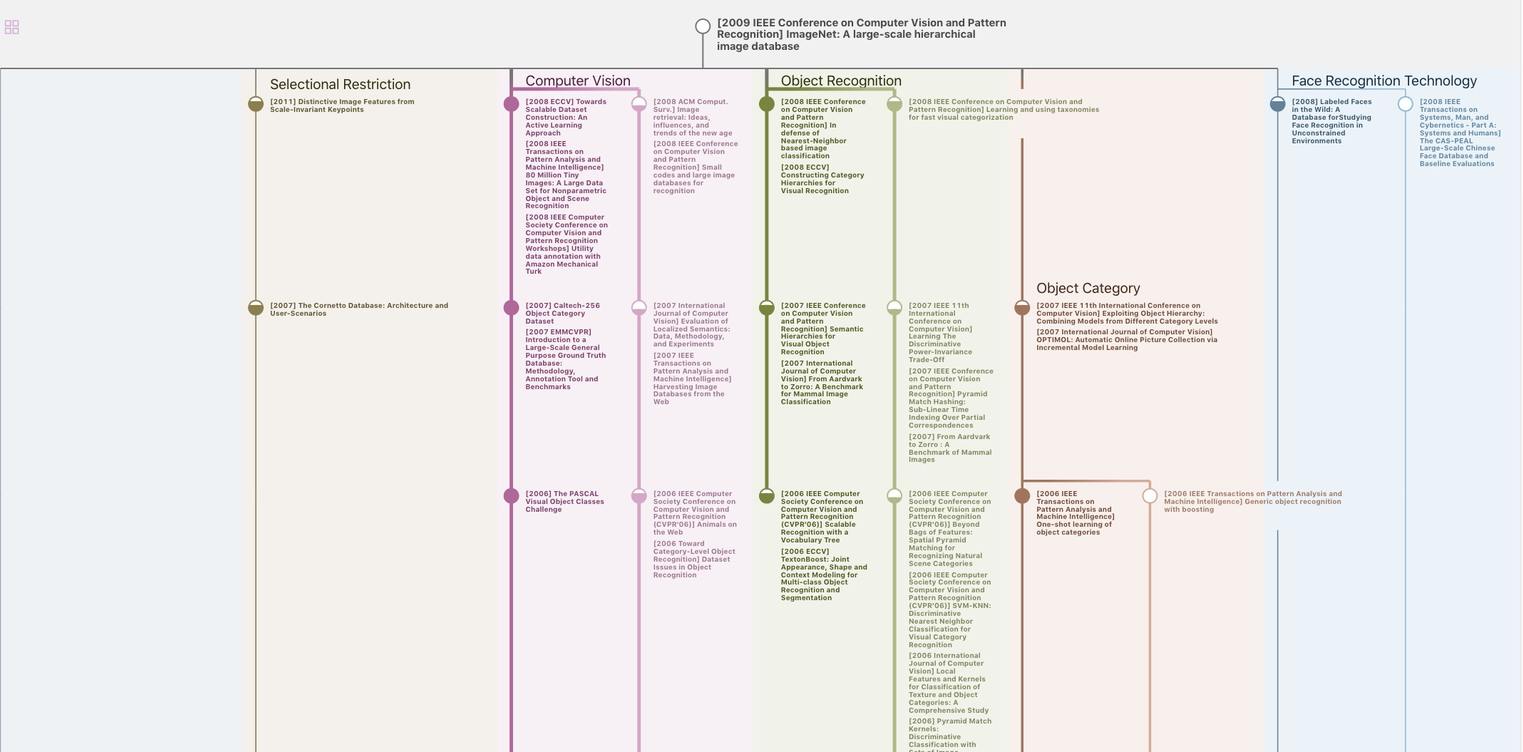
生成溯源树,研究论文发展脉络
Chat Paper
正在生成论文摘要