Efficient Learning of Rationalizable Equilibria in General-Sum Games
ICLR 2023(2023)
摘要
A natural goal in multi-agent learning is to learn \emph{rationalizable} behavior, where players learn to avoid any Iteratively Dominated Action (IDA). However, standard no-regret based equilibria-finding algorithms could take exponential samples to find such rationalizable strategies. In this paper, we first propose a simple yet sample-efficient algorithm for finding a rationalizable action profile in multi-player general-sum games under bandit feedback, which substantially improves over the results of Wu et al. We further develop algorithms with the first efficient guarantees for learning rationalizable Coarse Correlated Equilibria (CCE) and Correlated Equilibria (CE). Our algorithms incorporate several novel techniques to guarantee the elimination of IDA and no (swap-)regret simultaneously, including a correlated exploration scheme and adaptive learning rates, which may be of independent interest. We complement our results with a sample complexity lower bound showing the sharpness of our guarantees.
更多查看译文
关键词
Game Theory,Online Learning,Rationalizability
AI 理解论文
溯源树
样例
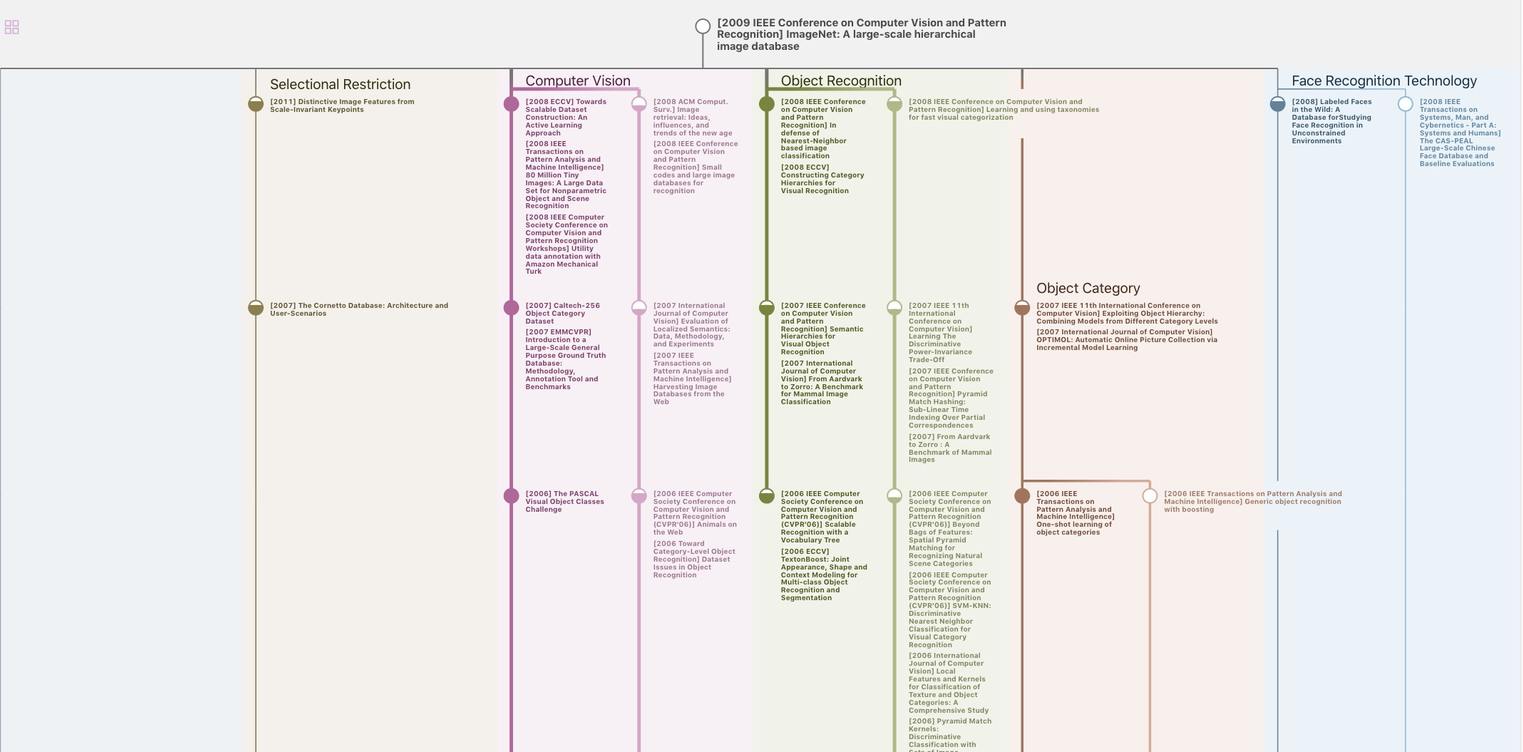
生成溯源树,研究论文发展脉络
Chat Paper
正在生成论文摘要