Lossless Adaptation of Pretrained Vision Models For Robotic Manipulation
ICLR 2023(2023)
摘要
Recent works have shown that large models pretrained on common visual learning tasks can provide useful representations for a wide range of specialized perception problems, as well as a variety of robotic manipulation tasks. While prior work on robotic manipulation has predominantly used frozen pretrained features, we demonstrate that in robotics, unlike in other domains, this approach can fail to reach optimal performance, and that fine-tuning of the full model can lead to significantly better results. Unfortunately, fine-tuning disrupts the pretrained visual representation, and causes representational drift towards the fine-tuned task thus leading to a loss of the versatility of the original model. We introduce a method for lossless adaptation to address this shortcoming of classical fine-tuning. We demonstrate that appropriate placement of our parameter efficient adapters can significantly reduce the performance gap between frozen pretrained representations and full end-to-end fine-tuning without changes to the original representation and thus preserving original capabilities of the pretrained model. We perform a comprehensive investigation across three major model architectures (ViTs, NFNets, and ResNets), supervised (ImageNet-1K classification) and self-supervised pretrained weights (CLIP, BYOL, Visual MAE) in three manipulation task domains and 35 individual tasks, and demonstrate that our claims are strongly validated in various settings.
更多查看译文
关键词
pretrained vision models,adaptation
AI 理解论文
溯源树
样例
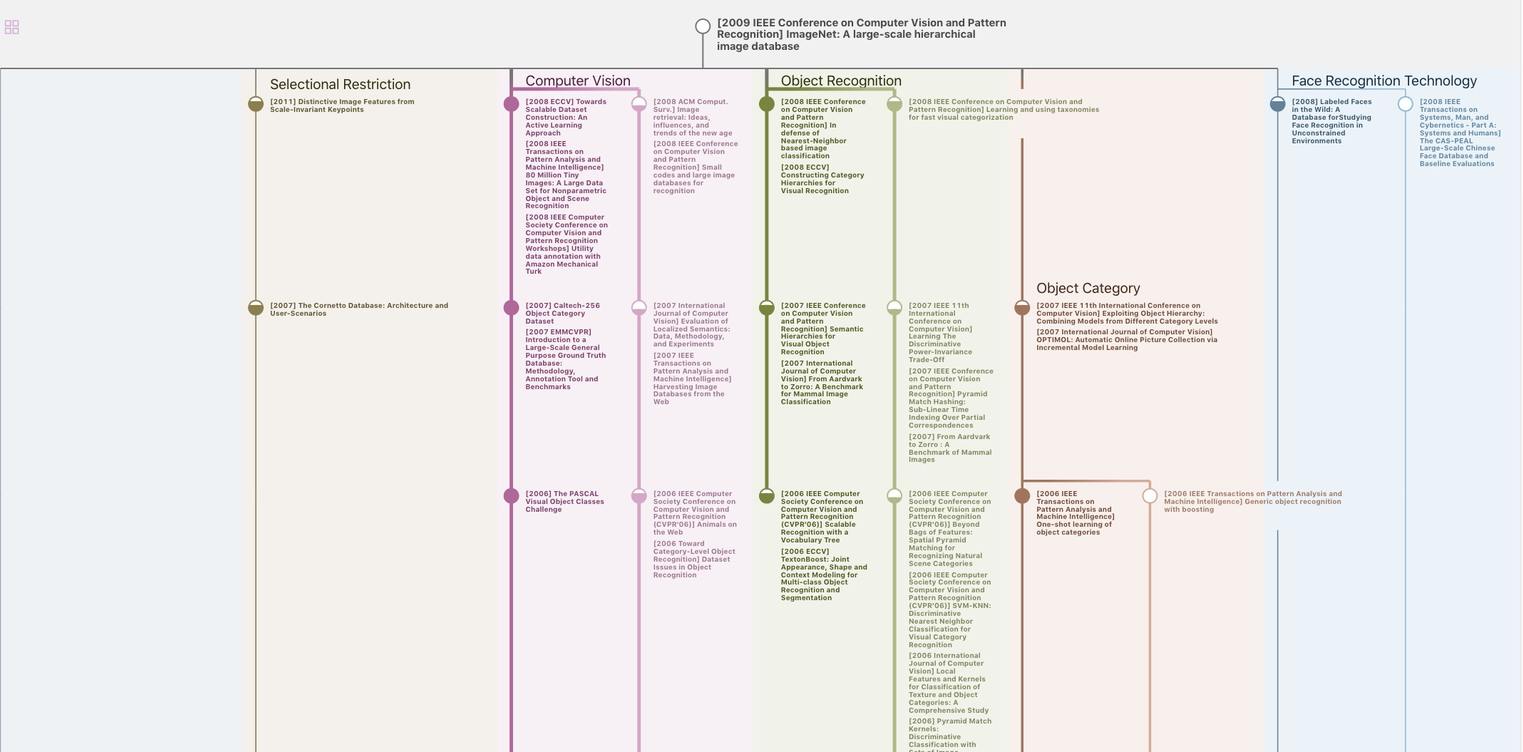
生成溯源树,研究论文发展脉络
Chat Paper
正在生成论文摘要