Projective Proximal Gradient Descent for Nonconvex Nonsmooth Optimization: Fast Convergence Without Kurdyka-Lojasiewicz (KL) Property
ICLR 2023(2023)
摘要
Nonconvex and nonsmooth optimization problems are important and challenging for statistics and machine learning. In this paper, we propose Projected Proximal Gradient Descent (PPGD) which solves a class of nonconvex and nonsmooth optimization problems, where the nonconvexity nd nonsmoothness come from a nonsmooth regularization term which is nonconvex but piecewise convex. In contrast with existing convergence analysis of accelerated PGD methods for nonconvex and nonsmooth problems based on the Kurdyka-Lojasiewicz (KL) property, we provide a new theoretical analysis showing that PPGD achieves optimal convergence rate on a class of nonconvex and nonsmooth problems under mild assumptions, which is the Nesterov's optimal convergence rate of first-order methods on smooth and convex objective function with Lipschitz continuous gradient. Experimental results demonstrate the effectiveness of the
PPGD.
更多查看译文
关键词
Nonconvex Nonsmooth Optimization,Projective Proximal Gradient Descent,Kurdyka-Lojasiewicz Property,Optimal Convergence Rate.
AI 理解论文
溯源树
样例
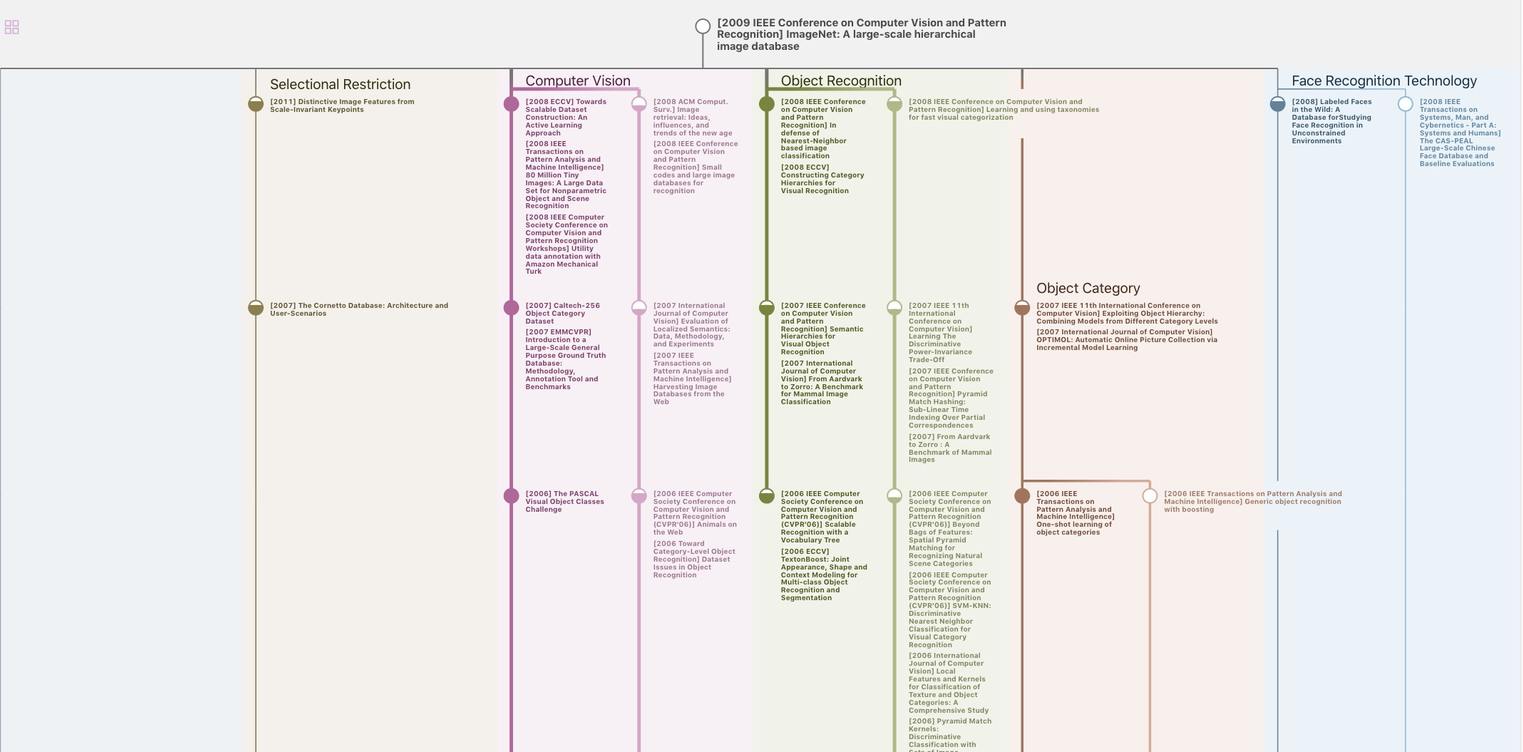
生成溯源树,研究论文发展脉络
Chat Paper
正在生成论文摘要