Enhancing Meta Learning via Multi-Objective Soft Improvement Functions
ICLR 2023(2023)
摘要
Meta-learning tries to leverage information from similar learning tasks. In the commonly-used bilevel optimization formulation, the shared parameter is learned in the outer loop by minimizing the average loss over all tasks. However, the converged solution may be comprised in that it only focuses on optimizing on a small subset of tasks. To alleviate this problem, we consider meta-learning as a multi-objective optimization (MOO) problem, in which each task is an objective. However, existing MOO solvers need to access all the objectives’ gradients in each iteration, and cannot scale to the huge number of tasks in typical meta-learning settings. To alleviate this problem, we propose a scalable gradient-based solver with the use of mini-batch. We provide theoretical guarantees on the Pareto optimality or Pareto stationarity of the converged solution. Empirical studies on various machine learning settings demonstrate that the proposed method is efficient, and achieves better performance than the baselines, particularly on improving the performance of the poorly-performing tasks and thus alleviating the compromising phenomenon.
更多查看译文
关键词
Meta Learning,Multi-Objective Optimization
AI 理解论文
溯源树
样例
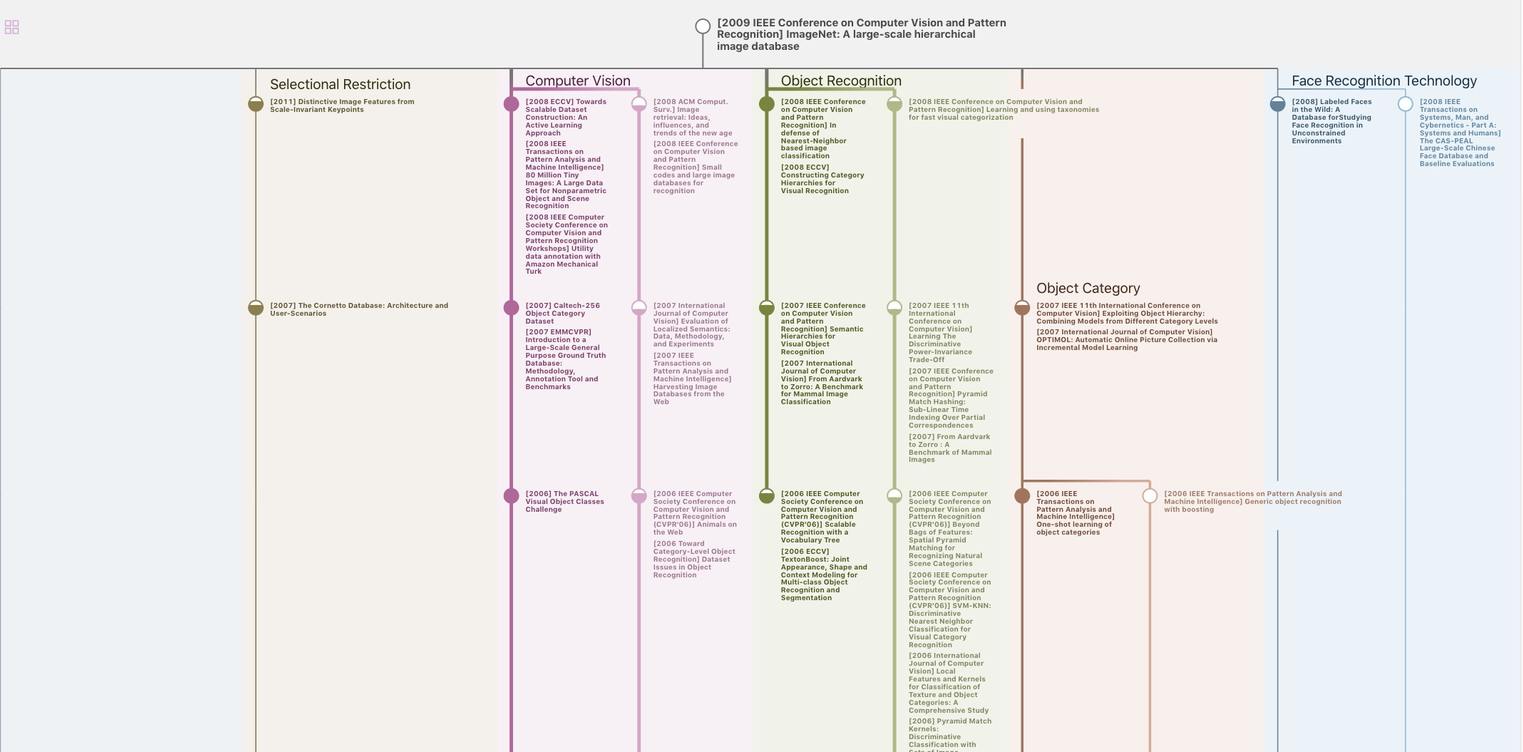
生成溯源树,研究论文发展脉络
Chat Paper
正在生成论文摘要