Computational Language Acquisition with Theory of Mind
ICLR 2023(2023)
摘要
Unlike current state-of-the-art language models, young children actively acquire language through interactions with their surrounding environment and caretakers. One mechanism that has been argued to be critical to language learning is the ability to infer the mental states of other agents in social environments, coined Theory of Mind (ToM) by Premack & Woodruff (1978). Drawing inspiration from the modern operationalized versions of ToM implemented in Rabinowitz et al. (2018) and Zhu et al. (2021), we build language-learning agents equipped with ToM, and measure its effects on the learning process.1 We model ToM by giving the speaker agent an internal listener model that is trained alongside the speaker and using this ToM model to rerank potential utterances. We also experiment with varying task difficulty, with the hypothesis that stronger environmental pressures will promote the development of more complex language. We find that speakers trained with a ToM listener component have higher accuracies than those trained without in our image referential game setting. We also find that increasing task difficulty in the training process results in more fluent, higher-quality utterances in evaluation. This suggests the utility of incorporating ToM, as well as other insights from child language acquisition, into computational models thereof.
更多查看译文
关键词
language acquisition,theory of mind,referential games,natural language processing
AI 理解论文
溯源树
样例
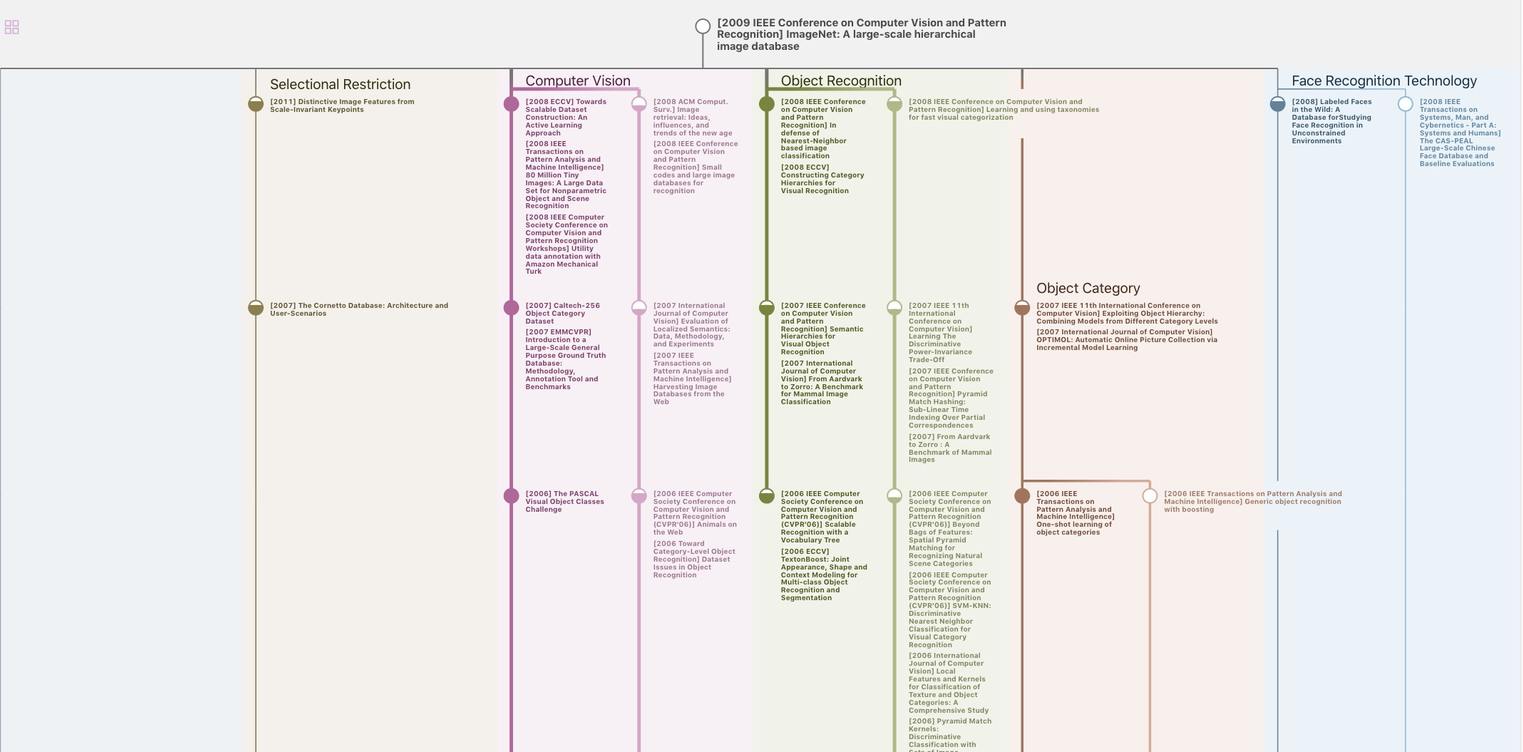
生成溯源树,研究论文发展脉络
Chat Paper
正在生成论文摘要