Behavior Proximal Policy Optimization
ICLR 2023(2023)
摘要
Offline reinforcement learning (RL) is a challenging setting where existing off-policy actor-critic methods perform poorly due to the overestimation of out-of-distribution actions. Thus, various additional augmentations are proposed to keep the learned policy close to the offline dataset (or behavior policy). In this work, starting from the analysis of offline monotonic policy improvement, we get a surprising finding that some online on-policy algorithms are naturally able to solve offline RL. Specifically, the inherent conservatism of these on-policy algorithms is exactly what the offline RL method needs to accomplish the closeness. Based on this, we design an algorithm called Behavior Proximal Policy Optimization (BPPO), which successfully solves offline RL without any extra constraint or regularization introduced. Extensive experiments on the D4RL benchmark indicate this extremely succinct method outperforms state-of-the-art offline RL algorithms.
更多查看译文
关键词
Offline Reinforcement Learning,Monotonic Policy Improvement
AI 理解论文
溯源树
样例
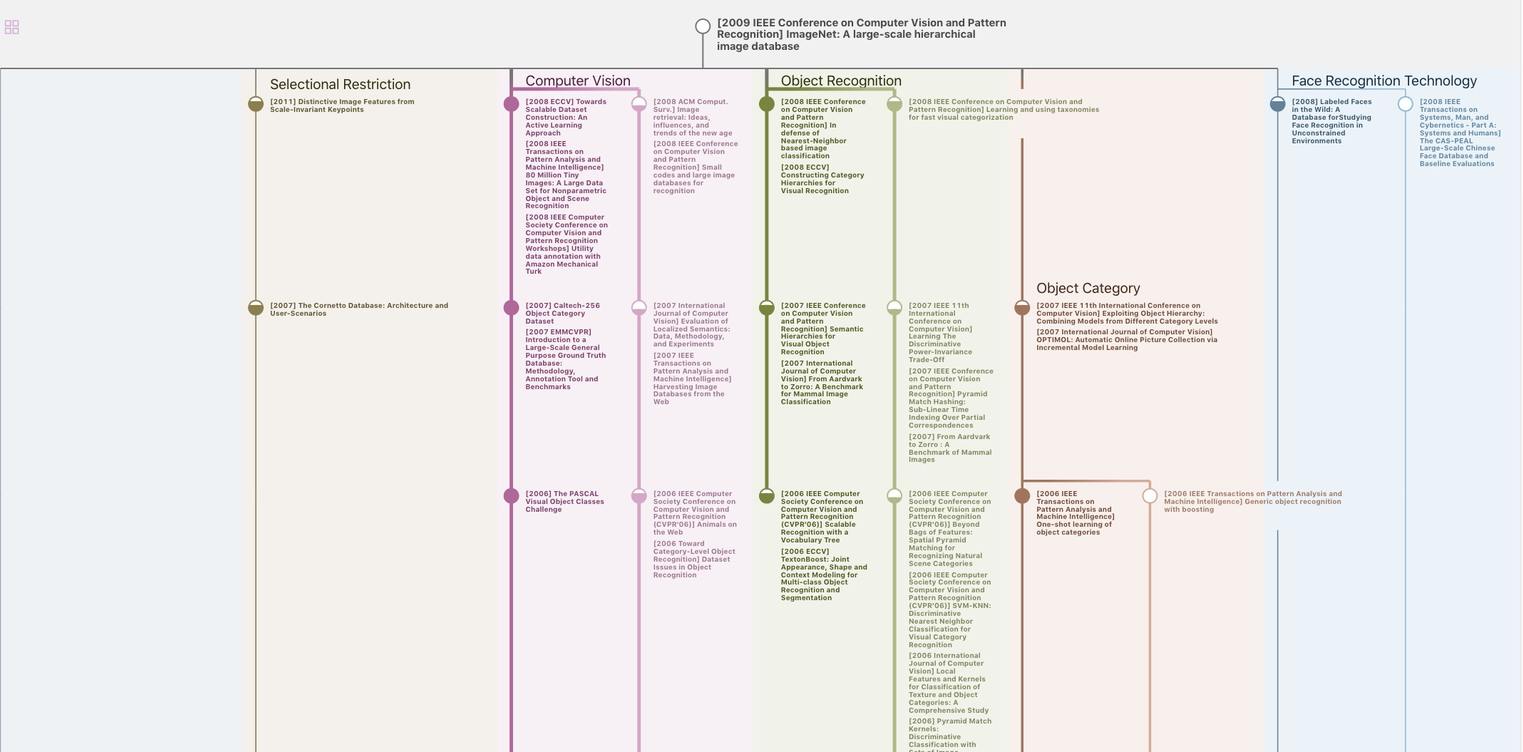
生成溯源树,研究论文发展脉络
Chat Paper
正在生成论文摘要