Learning Continuous Normalizing Flows For Faster Convergence To Target Distribution via Ascent Regularizations
ICLR 2023(2023)
摘要
Normalizing flows (NFs) have been shown to be advantageous in modeling complex distributions and improving sampling efficiency for unbiased sampling. In this work, we propose a new class of continuous NFs, ascent continuous normalizing flows (ACNFs), that makes a base distribution converge faster to a target distribution. Although solving such a flow is non-trivial and barely possible, we propose a practical implementation to learn flexibly parametric ACNFs via ascent regularization and apply in two learning cases: maximum likelihood learning for density estimation and minimizing reverse KL divergence for unbiased sampling and variational inference. The learned ACNFs demonstrate faster convergence towards the target distributions, therefore, achieving better density estimations, unbiased sampling and variational approximation at lower computational cost. Furthermore, the flows show to stabilize themselves to mitigate performance deterioration and are less sensitive to the choice of training flow length $T$ .
更多查看译文
关键词
normalizing flows,gradient flows,density estimation,unbiased sampling,variational inference
AI 理解论文
溯源树
样例
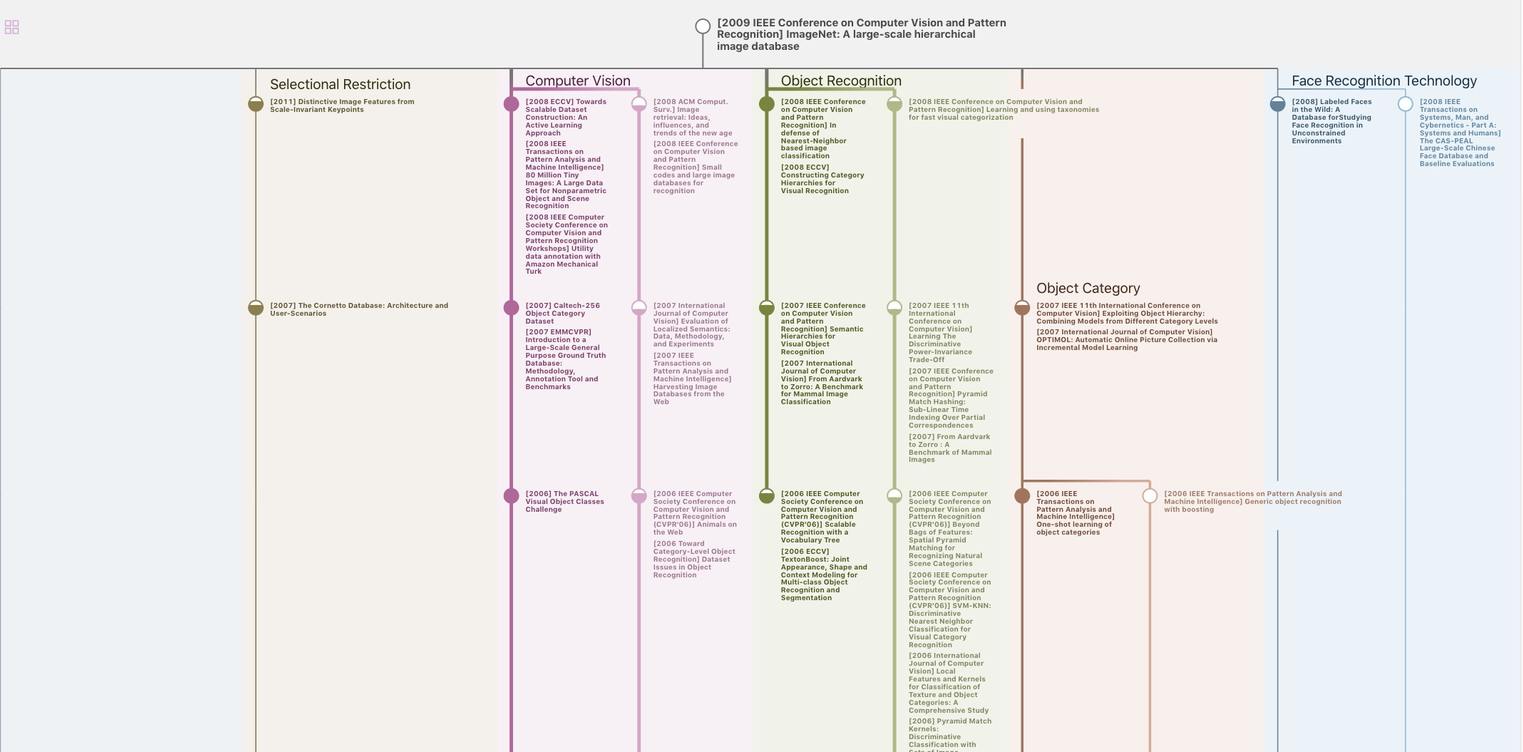
生成溯源树,研究论文发展脉络
Chat Paper
正在生成论文摘要