Continual Unsupervised Disentangling of Self-Organizing Representations
ICLR 2023(2023)
摘要
Limited progress has been made in continual unsupervised learning of representations, especially in reusing, expanding, and continually disentangling learned semantic factors across data environments. We argue that this is because existing approaches treat continually-arrived data independently, without considering how they are related based on the underlying semantic factors. We address this by a new generative model describing a topologically-connected mixture of spike-and-slab distributions in the latent space, learned end-to-end in a continual fashion via principled variational inference. The learned mixture is able to automatically discover the active semantic factors underlying each data environment and to accumulate their relational structure based on that. This distilled knowledge of different data environments can further be used for generative replay and guiding continual disentangling of new semantic factors. We tested the presented method on a split version of 3DShapes to provide the first quantitative disentanglement evaluation of continually learned representations, and further demonstrated its ability to continually disentangle new representations in benchmark datasets.
更多查看译文
关键词
continual disentanglment,generative model,VAE,SOM
AI 理解论文
溯源树
样例
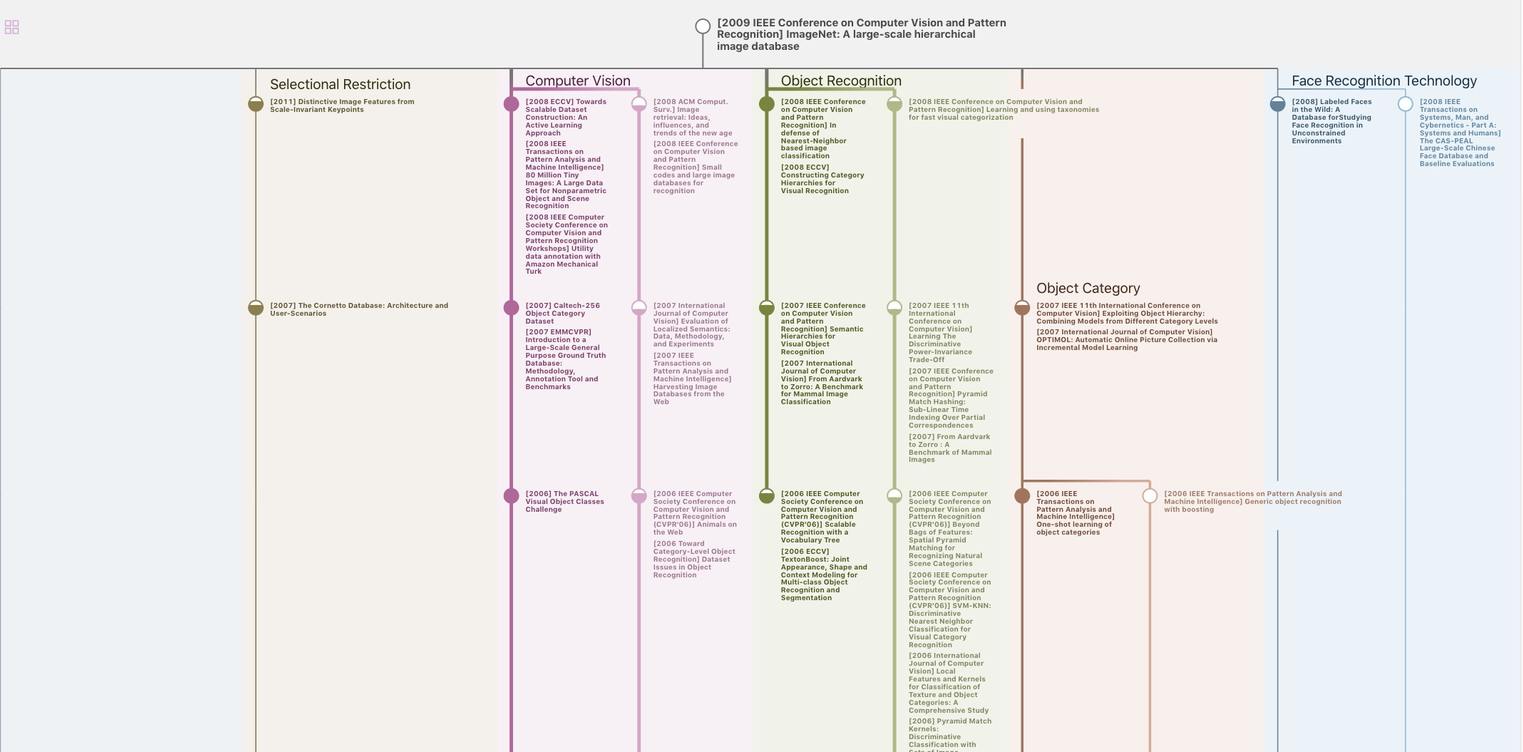
生成溯源树,研究论文发展脉络
Chat Paper
正在生成论文摘要