Parametrizing Product Shape Manifolds by Composite Networks
ICLR 2023(2023)
摘要
Parametrizations of data manifolds in shape spaces can be computed using the rich toolbox of Riemannian geometry. This, however, often comes with high computational costs, which raises the question if one can learn an efficient neural network approximation. We show that this is indeed possible for shape spaces with a special product structure, namely those smoothly approximable by a direct sum of low-dimensional manifolds. Our proposed architecture leverages this structure by separately learning approximations for the low-dimensional factors and a subsequent combination. After developing the approach as a general framework, we apply it to a shape space of triangular surfaces. Here, typical examples of data manifolds are given through datasets of articulated models and can be factorized, for example, by a Sparse Principal Geodesic Analysis (SPGA). We demonstrate the effectiveness of our proposed approach with experiments on synthetic data as well as manifolds extracted from data via SPGA.
更多查看译文
关键词
shape spaces,product manifolds,nonlinear statistics,low-dimensional data manifolds
AI 理解论文
溯源树
样例
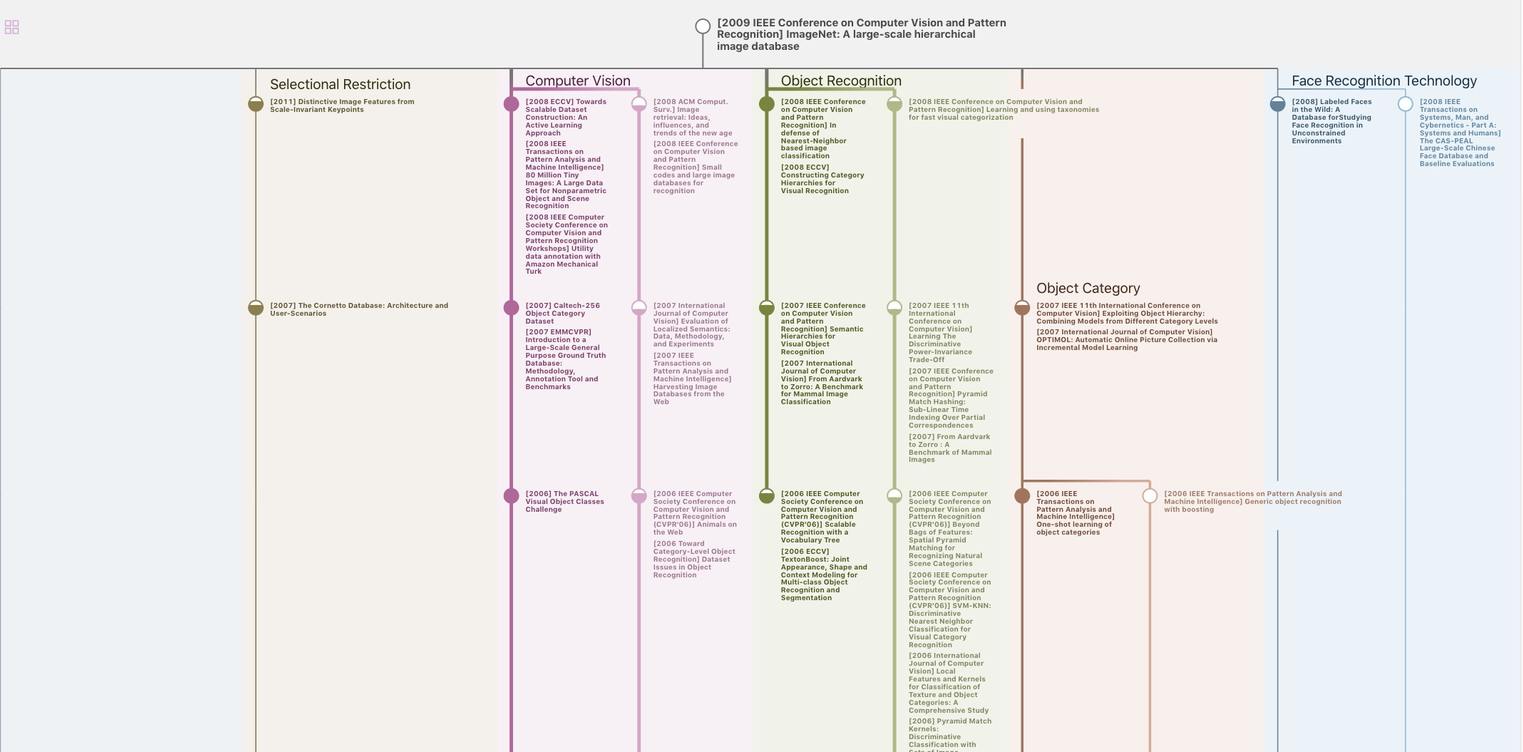
生成溯源树,研究论文发展脉络
Chat Paper
正在生成论文摘要