DINO as a von Mises-Fisher mixture model
ICLR 2023(2023)
摘要
Self-distillation methods using Siamese networks are popular for self-supervised pre-training. DINO is one such method based on a cross-entropy loss between $K$-dimensional probability vectors, obtained by applying a softmax function to the dot product between representations and learnt prototypes. Given the fact that the learned representations are $L^2$-normalized, we show that DINO can be interpreted as a mixture model of von Mises-Fisher components. With this interpretation, DINO assumes equal precision for all components when the prototypes are also $L^2$-normalized. Using this insight we propose DINO-vMF, that adds appropriate normalization constants when computing the cluster assignment probabilities. Unlike DINO, DINO-vMF is stable also for the larger ViT-Base model with unnormalized prototypes. We show that the added flexibility of the mixture model is beneficial in terms of better image representations. The DINO-vMF pre-trained model consistently performs better than DINO on a range of downstream tasks.
更多查看译文
关键词
self-supervised learning,vision transformers,mixture models
AI 理解论文
溯源树
样例
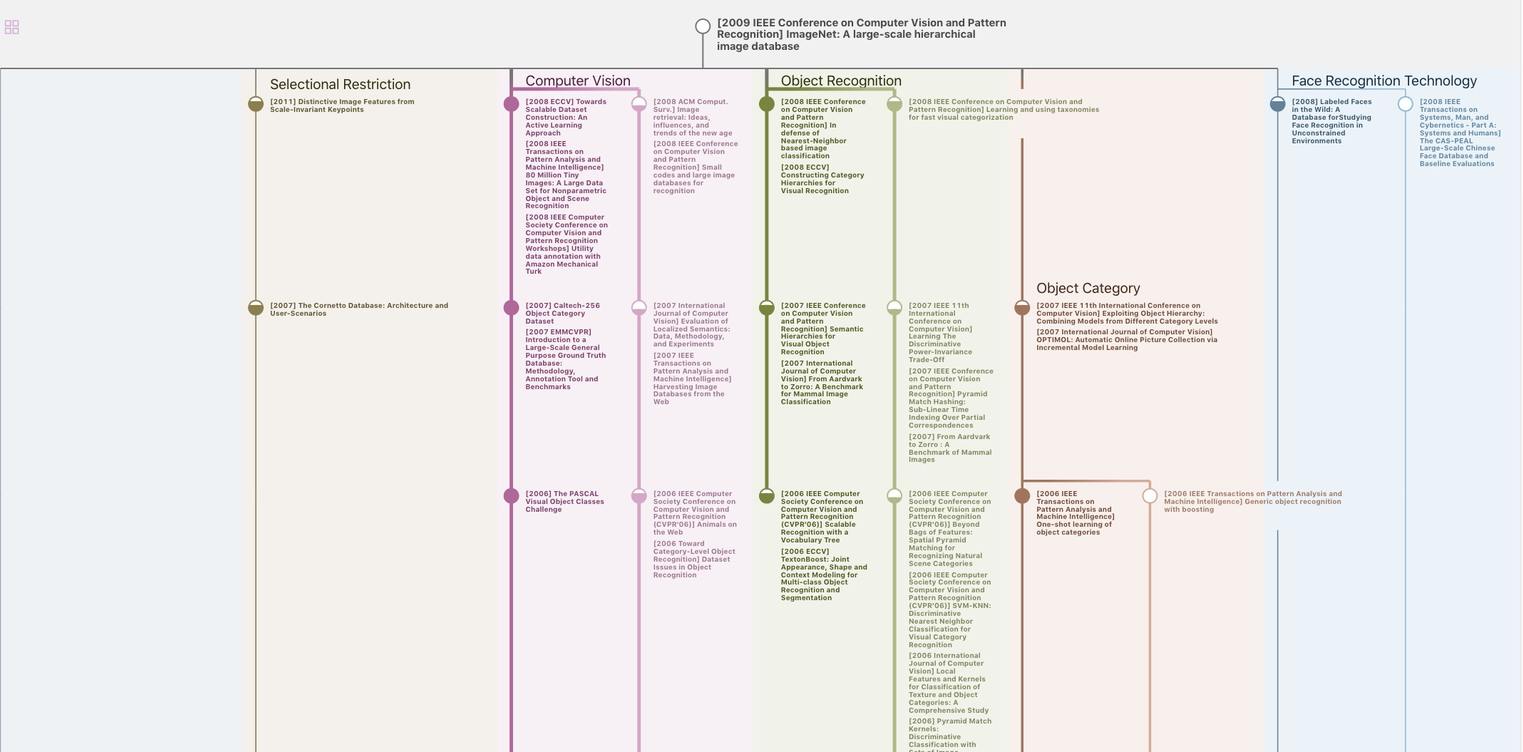
生成溯源树,研究论文发展脉络
Chat Paper
正在生成论文摘要