GEASS: Neural causal feature selection for high-dimensional biological data
ICLR 2023(2023)
摘要
Identifying nonlinear causal relationships in high-dimensional biological data is an important task. However, current neural network based causality detection approaches for such data suffer from poor interpretability and cannot scale well to high dimensional regime. Here we present GEASS (Granger fEAture Selection of Spatiotemporal data), which identifies sparse Granger causality mechanisms of high dimensional spatiotemporal data by a single neural network. GEASS maximizes sparsity-regularized multi-dimensional transfer entropy with a theoretical guarantee of recovering features with spatial/temporal Granger causal relationships. The sparsity regularization is achieved by a novel combinatorial stochastic gate layer to select sparse non-overlapping feature subsets. We demonstrate the efficacy of GEASS in several synthetic datasets and real biological data from single-cell RNA sequencing and spatial transcriptomics.
更多查看译文
关键词
Granger causality,feature selection,neural networks,single-cell genomics,spatial transcriptomics
AI 理解论文
溯源树
样例
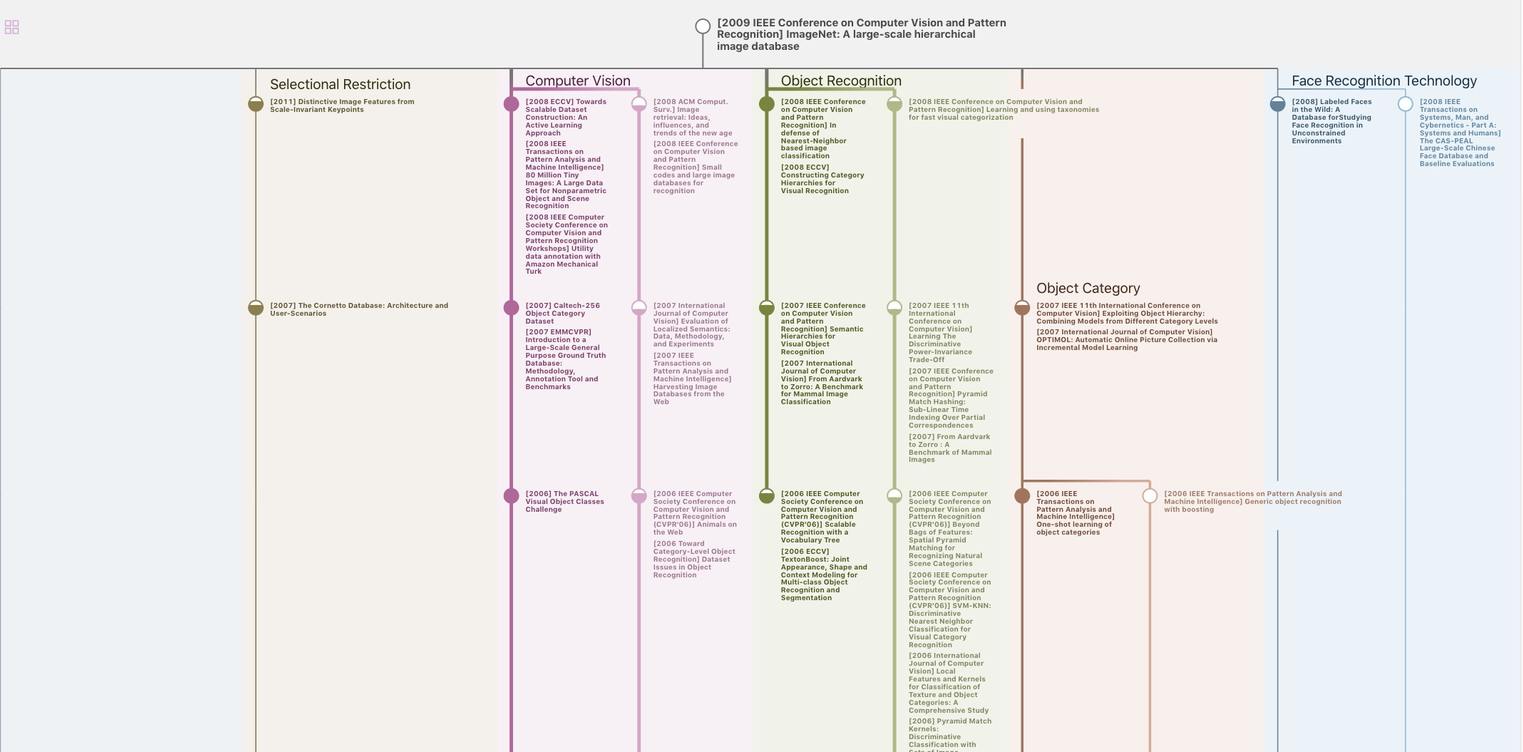
生成溯源树,研究论文发展脉络
Chat Paper
正在生成论文摘要