Image to Sphere: Learning Equivariant Features for Efficient Pose Prediction
ICLR 2023(2023)
摘要
Predicting the pose of objects from a single image is an important but difficult computer vision problem. Methods that predict a single point estimate do not predict the pose of objects with symmetries well and cannot represent uncertainty. Alternatively, some works predict a distribution over orientations in $\mathrm{SO}(3)$. However, training such models can be computation- and sample-inefficient. Instead, we propose a novel mapping of features from the image domain to the 3D rotation manifold. Our method then leverages $\mathrm{SO}(3)$ equivariant layers, which are more sample efficient, and outputs a distribution over rotations that can be sampled at arbitrary resolution. We demonstrate the effectiveness of our method at object orientation prediction, and achieve state-of-the-art performance on the popular PASCAL3D+ dataset. Moreover, we show that our method can model complex object symmetries, without any modifications to the parameters or loss function.
更多查看译文
关键词
equivariance,sample efficiency,pose detection,symmetry,SO(3)
AI 理解论文
溯源树
样例
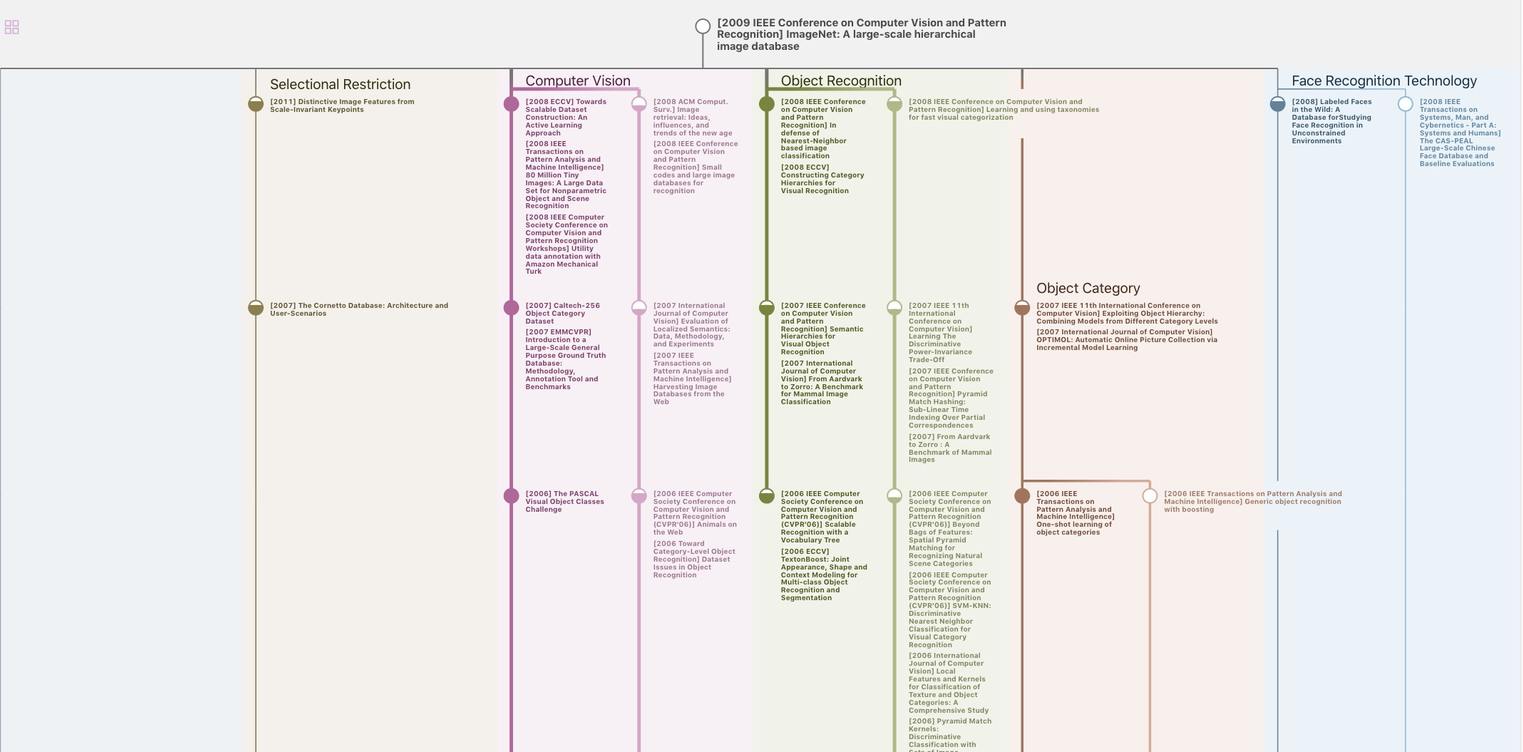
生成溯源树,研究论文发展脉络
Chat Paper
正在生成论文摘要