An enhanced surrogate-assisted differential evolution for constrained optimization problems
Soft Computing(2023)
摘要
The application of evolutionary algorithms (EAs) to complex engineering optimization problems may present difficulties as they require many evaluations of the objective functions by computationally expensive simulation procedures. To deal with this issue, surrogate models have been employed to replace those expensive simulations. In this work, a surrogate-assisted evolutionary optimization procedure is proposed. The procedure combines the differential evolution method with a k -nearest neighbors ( k -NN) similarity-based surrogate model. In this approach, the database that stores the solutions evaluated by the exact model, which are used to approximate new solutions, is managed according to a merit scheme. Constraints are handled by a rank-based technique that builds multiple separate queues based on the values of the objective function and the violation of each constraint. Also, to avoid premature convergence of the method, a strategy that triggers a random reinitialization of the population is considered. The performance of the proposed method is assessed by numerical experiments using 24 constrained benchmark functions and 5 mechanical engineering problems. The results show that the method achieves optimal solutions with a remarkably reduction in the number of function evaluations compared to the literature.
更多查看译文
关键词
Constrained optimization problems,Evolutionary algorithms,Surrogate models,Constraint-handling techniques
AI 理解论文
溯源树
样例
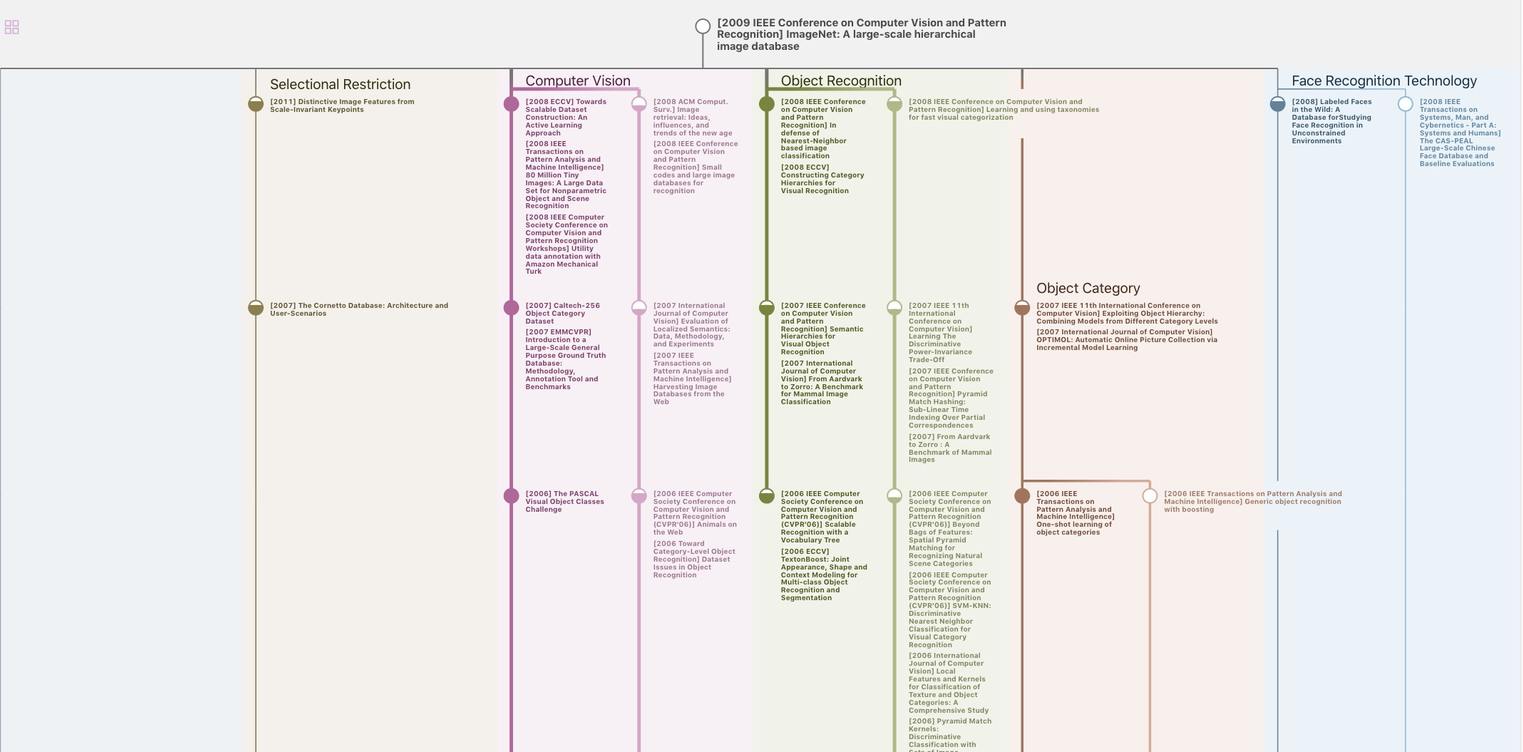
生成溯源树,研究论文发展脉络
Chat Paper
正在生成论文摘要