SceneDreamer: Unbounded 3D Scene Generation From 2D Image Collections
IEEE TRANSACTIONS ON PATTERN ANALYSIS AND MACHINE INTELLIGENCE(2023)
摘要
In this work, we present SceneDreamer, an unconditional generative model for unbounded 3D scenes, which synthesizes large-scale 3D landscapes from random noise. Our framework is learned from in-the-wild 2D image collections only, without any 3D annotations. At the core of SceneDreamer is a principled learning paradigm comprising: 1) an efficient yet expressive 3D scene representation, 2) a generative scene parameterization, and 3) an effective renderer that can leverage the knowledge from 2D images. Our approach begins with an efficient bird's-eye-view (BEV) representation generated from simplex noise, which includes a height field for surface elevation and a semantic field for detailed scene semantics. This BEV scene representation enables: 1) representing a 3D scene with quadratic complexity, 2) disentangled geometry and semantics, and 3) efficient training. Moreover, we propose a novel generative neural hash grid to parameterize the latent space based on 3D positions and scene semantics, aiming to encode generalizable features across various scenes. Lastly, a neural volumetric renderer, learned from 2D image collections through adversarial training, is employed to produce photorealistic images. Extensive experiments demonstrate the effectiveness of SceneDreamer and superiority over state-of-the-art methods in generating vivid yet diverse unbounded 3D worlds.
更多查看译文
关键词
Three-dimensional displays,Solid modeling,Semantics,Cameras,Training,Rendering (computer graphics),Geometry,3D generative model,GAN,neural rendering,unbounded scene generation
AI 理解论文
溯源树
样例
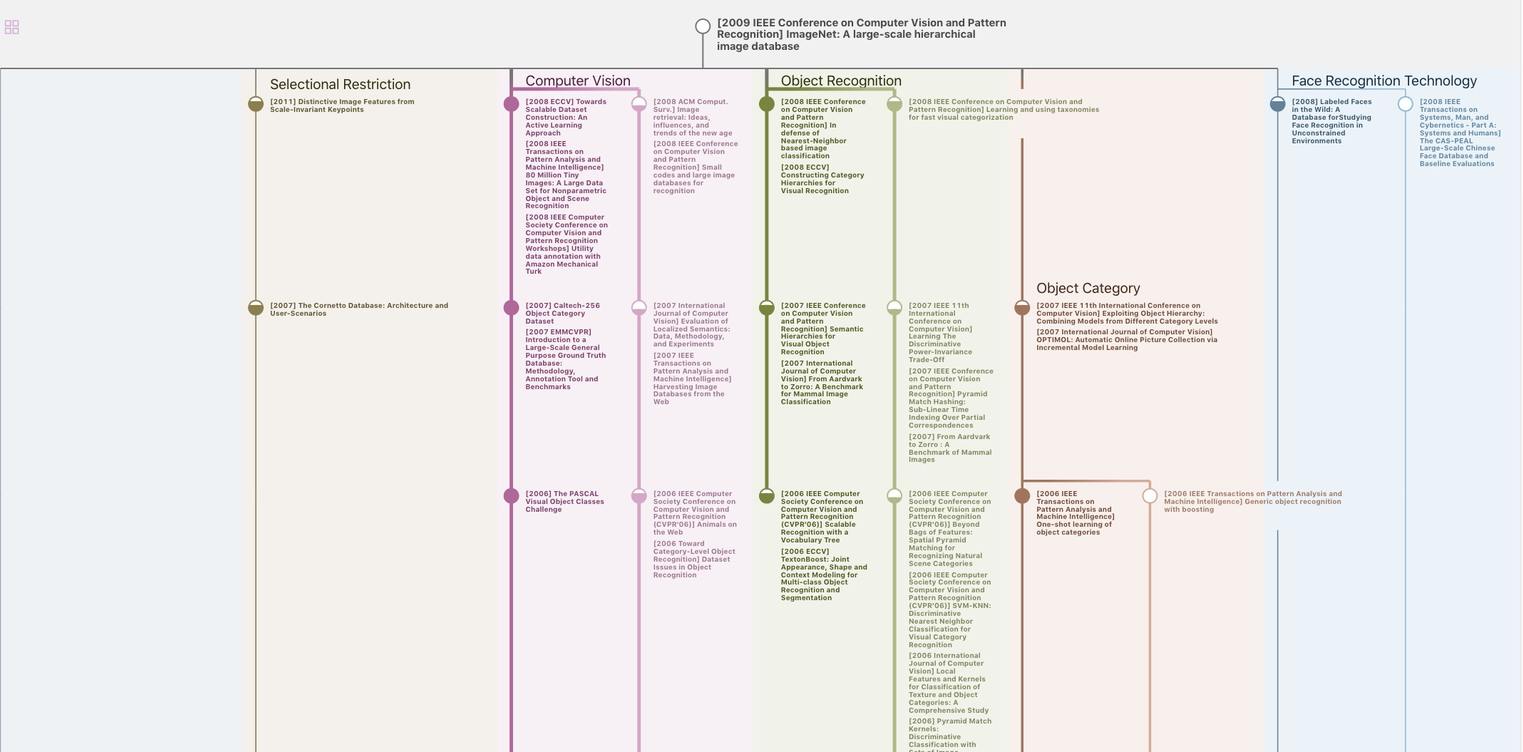
生成溯源树,研究论文发展脉络
Chat Paper
正在生成论文摘要