Causal lifting and link prediction
arxiv(2023)
摘要
Existing causal models for link prediction assume an underlying set of inherent node factors-an innate characteristic defined at the node's birth-that governs the causal evolution of links in the graph. In some causal tasks, however, link formation is path-dependent: the outcome of link interventions depends on existing links. Unfortunately, these existing causal methods are not designed for path-dependent link formation, as the cascading functional dependencies between links (arising from path dependence) are either unidentifiable or require an impractical number of control variables. To overcome this, we develop the first causal model capable of dealing with path dependencies in link prediction. In this work, we introduce the concept of causal lifting, an invariance in causal models of independent interest that, on graphs, allows the identification of causal link prediction queries using limited interventional data. Further, we show how structural pairwise embeddings exhibit lower bias and correctly represent the task's causal structure, as opposed to existing node embeddings, e.g. graph neural network node embeddings and matrix factorization. Finally, we validate our theoretical findings on three scenarios for causal link prediction tasks: knowledge base completion, covariance matrix estimation and consumer-product recommendations.
更多查看译文
关键词
causal inference,link prediction,graph embeddings,causal representation learning,geometric deep learning
AI 理解论文
溯源树
样例
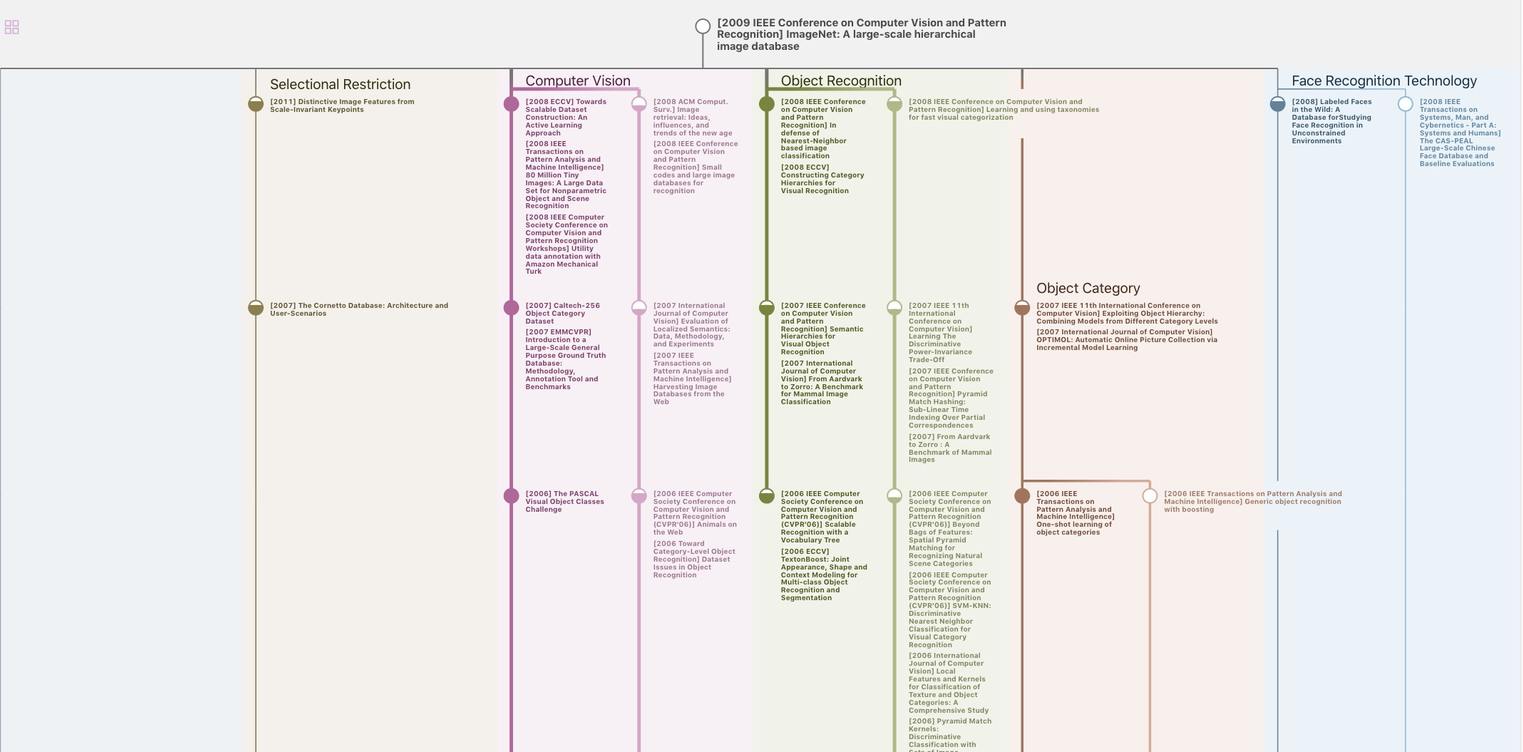
生成溯源树,研究论文发展脉络
Chat Paper
正在生成论文摘要