A general Markov decision process formalism for action-state entropy-regularized reward maximization
arxiv(2023)
摘要
Previous work has separately addressed different forms of action, state and action-state entropy regularization, pure exploration and space occupation. These problems have become extremely relevant for regularization, generalization, speeding up learning and providing robust solutions at unprecedented levels. However, solutions of those problems are hectic, ranging from convex and non-convex optimization, and unconstrained optimization to constrained optimization. Here we provide a general dual function formalism that transforms the constrained optimization problem into an unconstrained convex one for any mixture of action and state entropies. The cases with pure action entropy and pure state entropy are understood as limits of the mixture.
更多查看译文
关键词
markov,decision,action-state,entropy-regularized
AI 理解论文
溯源树
样例
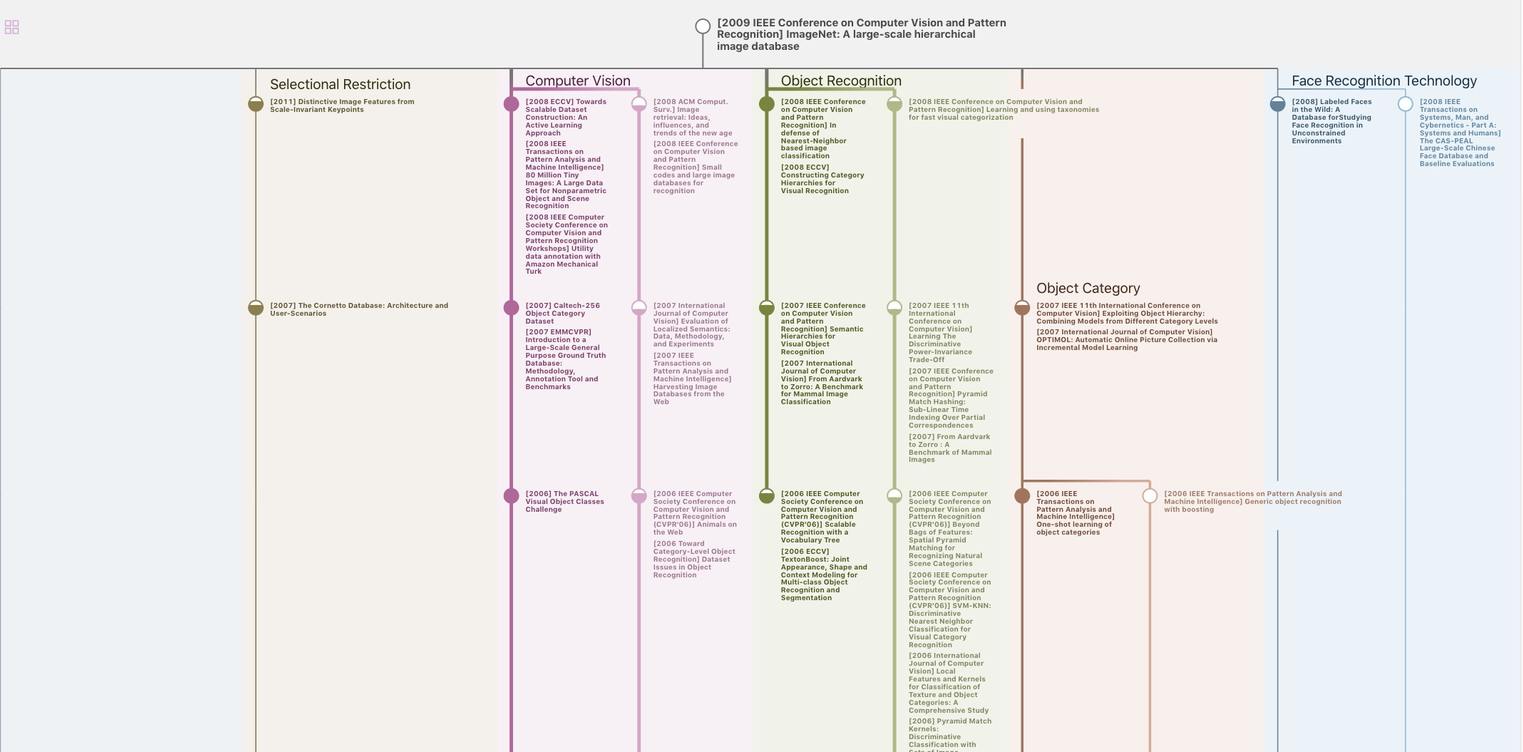
生成溯源树,研究论文发展脉络
Chat Paper
正在生成论文摘要