MedSkim: Denoised Health Risk Prediction via Skimming Medical Claims Data
2022 IEEE International Conference on Data Mining (ICDM)(2022)
摘要
Health risk prediction is a challenge task that aims to predict whether patients would suffer from a certain disease/condition in the near future based on their historical EHR data. Although existing approaches can achieve better performance, none of them can deal with the noise existing in the EHR data explicitly. In this paper, we hypothesize that automatically removing noise from EHR data should help the models further improve the performance. Correspondingly, we propose a novel model named MedSkim, which is able to automatically rule out irrelevant visits and codes by effectively skimming through the EHR data. In particular, the proposed model has a code selection module that can directly make a skipping decision to each individual diagnosis codes and then remove the target-irrelevant ones. A backward probing RNN (BPRNN) is designed to reversely process the EHR data and provide a coarse grained representation learning for visits. Besides, a forward skipping RNN (FSRNN) is proposed to read the EHR in a preceding way and dynamically select important visits and codes based on the results of previous two modules. Finally, the risk prediction module uses the output hidden states from FSRNN for generating the final representation to make predictions. Additionally, we also design an extra regularization term based on the skip rate of the model and combine it with standard cross entropy loss to train the model in an end-to-end setting. Experimental results show that MedSkim achieves the best performance on three real-world datasets compared with the state-of-the-art baselines in terms of PR-AUC, F1 and Cohen’s Kappa. Moreover, the ablation study and case study confirm that the proposed MedSkim is reasonable and effective for removing noise from EHR data
1
.
1
The source code of the proposed MedSkim is available at https://github.com/SH-Src/MedSkim
更多查看译文
关键词
Health Risk Prediction,Electronic Health Records,Denoising Algorithm
AI 理解论文
溯源树
样例
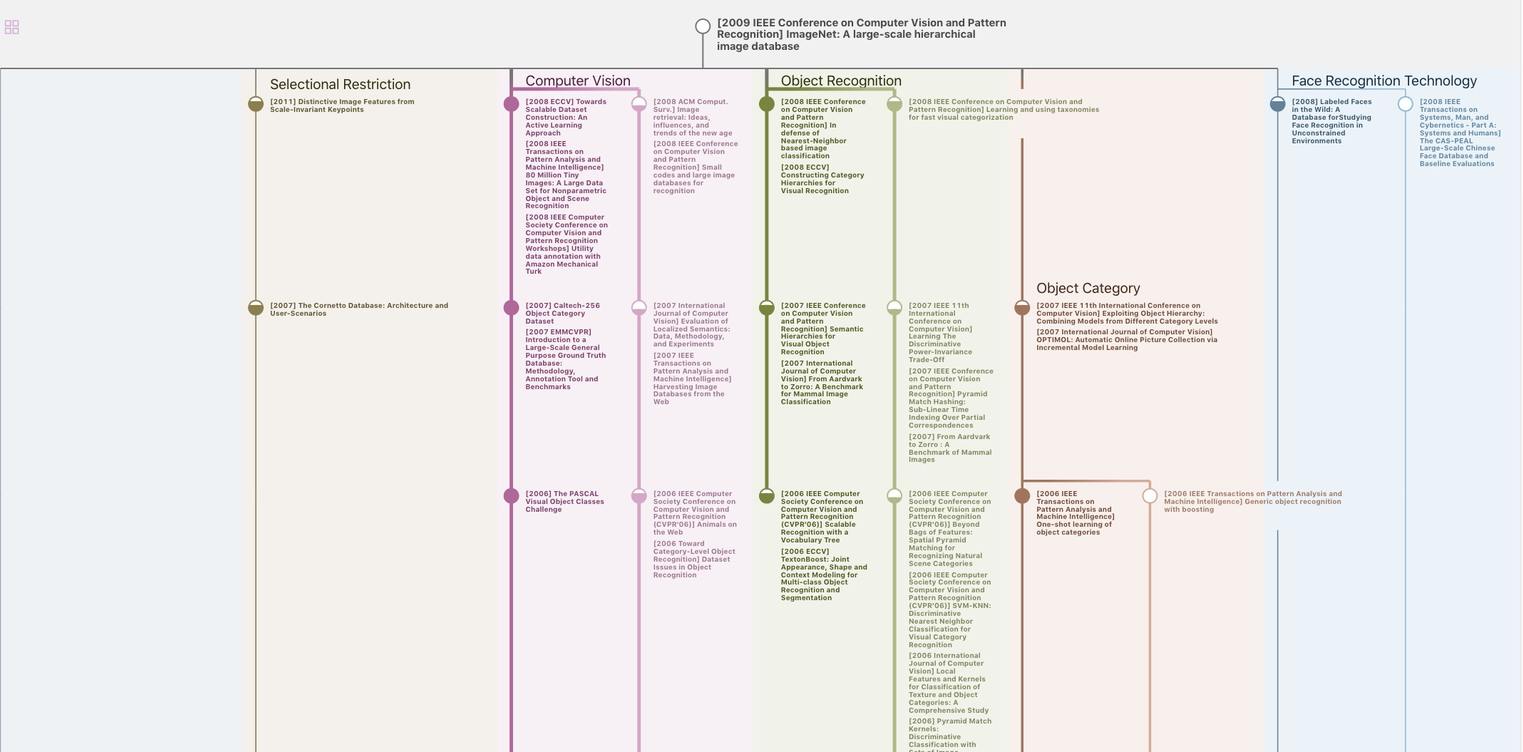
生成溯源树,研究论文发展脉络
Chat Paper
正在生成论文摘要