HFML: heterogeneous hierarchical federated mutual learning on non-IID data
Annals of Operations Research(2023)
摘要
Non-independent and identical distribution (Non-IID) data and model heterogeneity pose a great challenge for federated learning in cloud-based and edge-based systems. They are easy to lead to inconsistency of gradient updates during the training stage and mismatch of gradient dimensions during the aggregation stage, resulting in the degradation of the global model performance and the consumption of a lot of training time. To solve these problems, this paper proposes a Heterogeneous Hierarchical Federated Mutual Learning (HFML) method in an edge-based system. We design a model assignment mechanism in which clients and edge servers individually fork global models of different structures, and the untrained local models learn mutually with the edge models in deep mutual learning. We use partial periodic aggregation to approximate global aggregation to achieve fast convergence. Our experiments show that HFML obtains state-of-the-art performance than three approaches on common datasets like CIFAR-10/100. Our method improves accuracy up to 2.9% and reduces training time by 30% under homogeneous and heterogeneous models.
更多查看译文
关键词
Federated learning,Non-independent and identical distribution (Non-IID),Heterogeneous models,Deep mutual learning
AI 理解论文
溯源树
样例
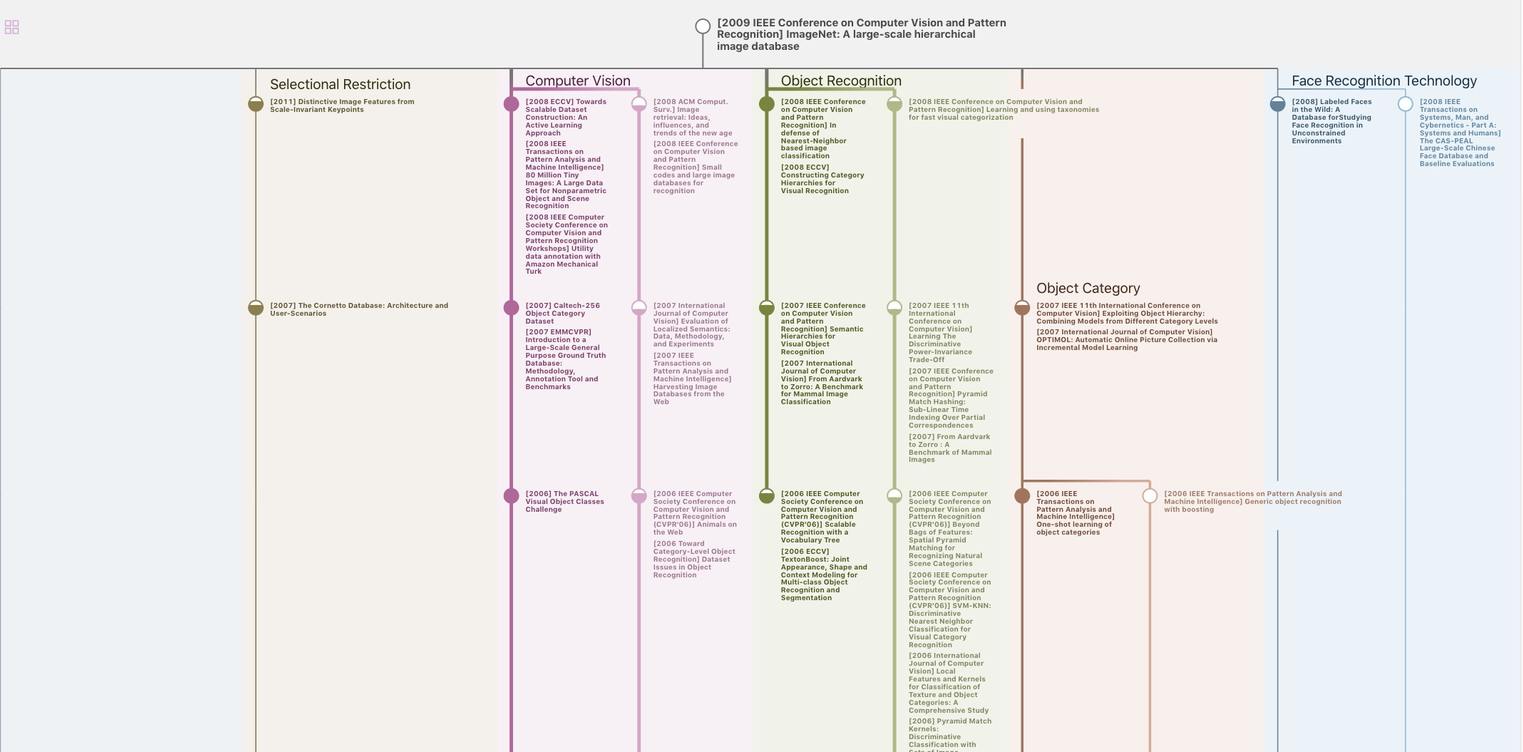
生成溯源树,研究论文发展脉络
Chat Paper
正在生成论文摘要