Discrete entropy-based health indicator and LSTM for the forecasting of bearing health
Journal of the Brazilian Society of Mechanical Sciences and Engineering(2023)
摘要
This work is dedicated to develop a novel discrete probabilistic entropy-based health indicator (HI) and long short-term memory (LSTM)-based method to forecast bearing health. The desired discrete probabilistic entropy measure is resulted from the proclaimed symmetric information discrimination measure between two discrete probability distributions. The proposed indicator is robust and unaffected by load and speed. The proposed HI is utilized to create the LSTM model, which can predict bearing health. Three different types of data sets are utilized to evaluate the proposed method. A comparison has also been made between the proposed method and the LSTM model with existing features. LSTM's performance has been compared to that of various time-series prediction models, including shallow artificial intelligence models such as ANN, bidirectional LSTM, and un-clipped LSTM. The comparison demonstrates that the proposed method outperforms other time-series prediction models.
更多查看译文
关键词
Bearing health, Deep learning, Forecasting, Health indicator, LSTM, Vibration
AI 理解论文
溯源树
样例
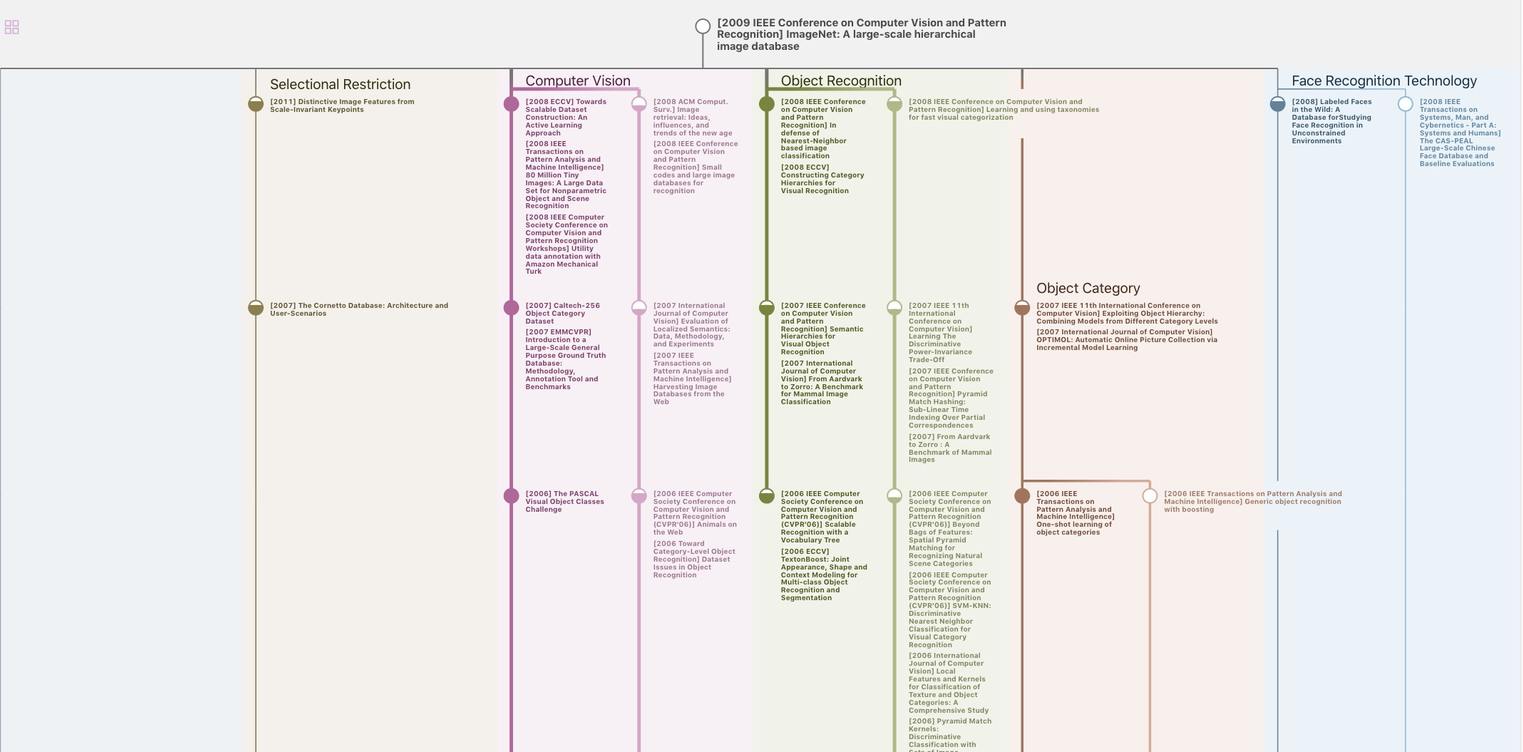
生成溯源树,研究论文发展脉络
Chat Paper
正在生成论文摘要