Topological data analysis of thoracic radiographic images shows improved radiomics-based lung tumor histology prediction
Patterns(2023)
摘要
Topological data analysis provides tools to capture wide-scale structural shape information in data. Its main method, persistent homology, has found successful applications to various machine-learning problems. Despite its recent gain in popularity, much of its potential for medical image analysis remains undiscovered. We explore the prominent learning problems on thoracic radiographic images of lung tumors for which persistent homology improves radiomic-based learning. It turns out that our topological features well capture complementary information important for benign versus malignant and adenocarcinoma versus squamous cell carcinoma tumor prediction while contributing less consistently to small cell versus non-small cell—an interesting result in its own right. Furthermore, while radiomic features are better for predicting malignancy scores assigned by expert radiologists through visual inspection, we find that topological features are better for predicting more accurate histology assessed through long-term radiology review, biopsy, surgical resection, progression, or response.
更多查看译文
关键词
topological data analysis,radiology,histology,computed tomography,computer vision,supervised learning,feature engineering
AI 理解论文
溯源树
样例
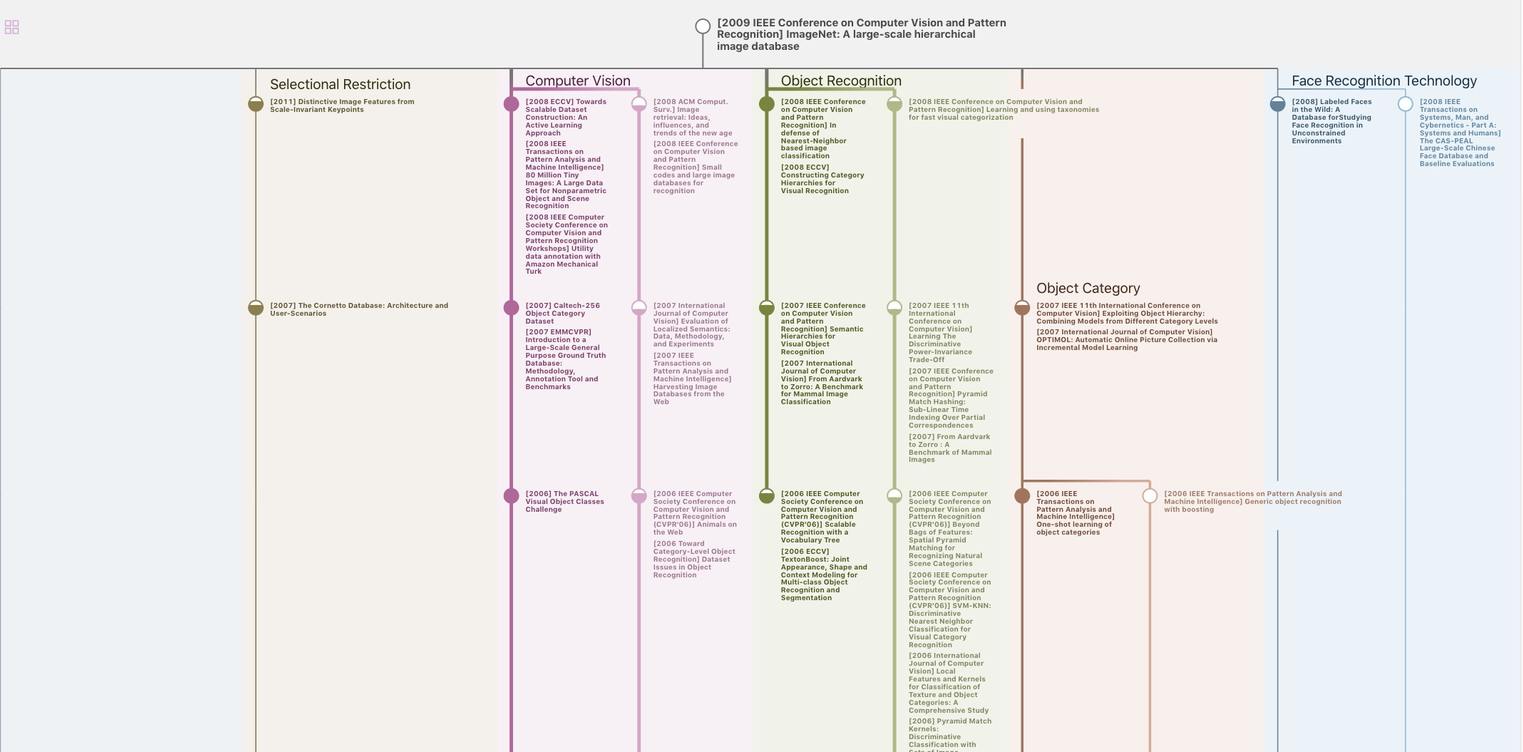
生成溯源树,研究论文发展脉络
Chat Paper
正在生成论文摘要