Assessing atypical brain functional connectivity development: An approach based on generative adversarial networks.
Frontiers in neuroscience(2022)
摘要
Generative Adversarial Networks (GANs) are promising analytical tools in machine learning applications. Characterizing atypical neurodevelopmental processes might be useful in establishing diagnostic and prognostic biomarkers of psychiatric disorders. In this article, we investigate the potential of GANs models combined with functional connectivity (FC) measures to build a predictive neurotypicality score 3-years after scanning. We used a ROI-to-ROI analysis of resting-state functional magnetic resonance imaging (fMRI) data from a community-based cohort of children and adolescents (377 neurotypical and 126 atypical participants). Models were trained on data from neurotypical participants, capturing their sample variability of FC. The discriminator subnetwork of each GAN model discriminated between the learned neurotypical functional connectivity pattern and atypical or unrelated patterns. Discriminator models were combined in ensembles, improving discrimination performance. Explanations for the model's predictions are provided using the LIME (Local Interpretable Model-Agnostic) algorithm and local hubs are identified in light of these explanations. Our findings suggest this approach is a promising strategy to build potential biomarkers based on functional connectivity.
更多查看译文
关键词
GANs,biomarker,children,functional connectivity,machine learning (ML),neural networks,neurodevelopment
AI 理解论文
溯源树
样例
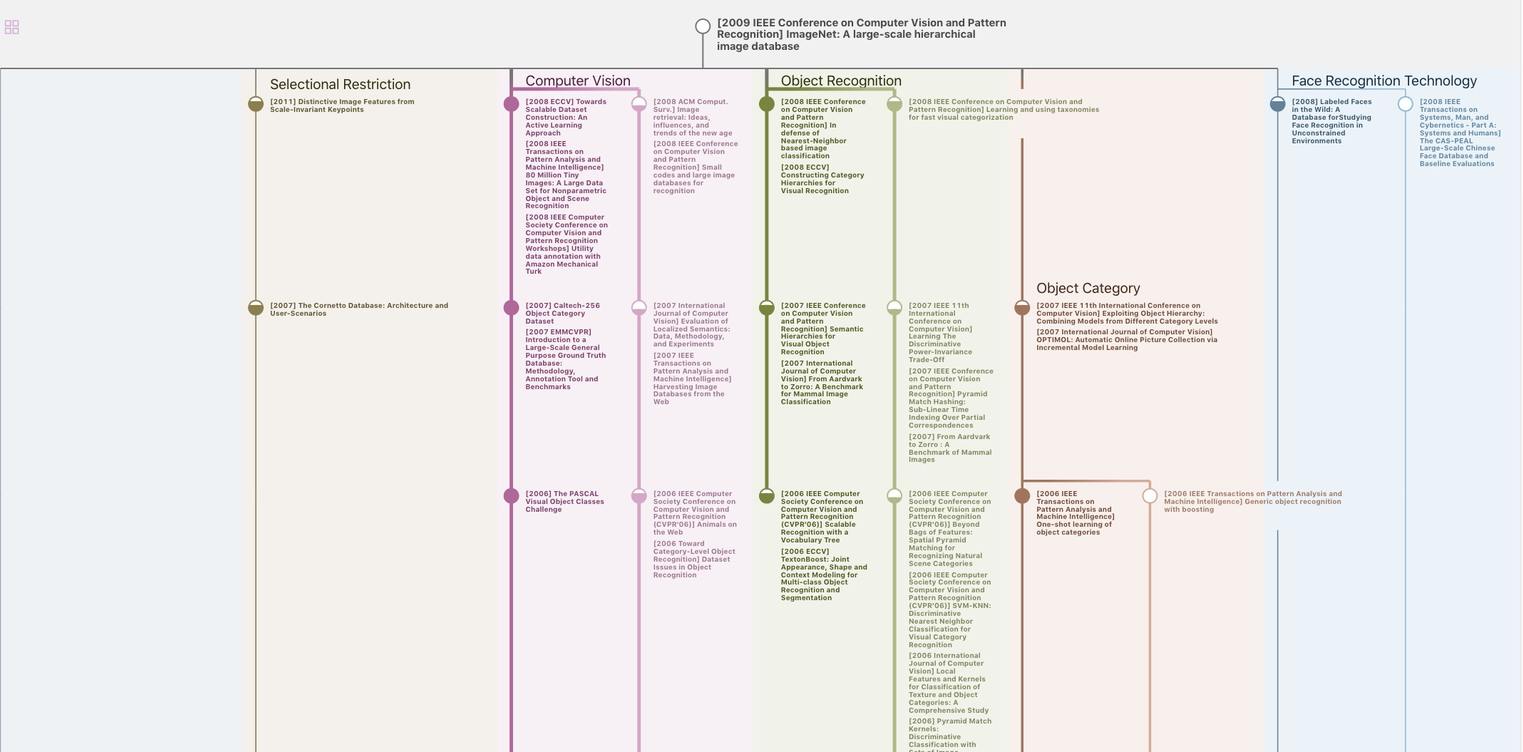
生成溯源树,研究论文发展脉络
Chat Paper
正在生成论文摘要