Energy-Based Survival Models for Predictive Maintenance
arxiv(2023)
摘要
Predictive maintenance is an effective tool for reducing maintenance costs. Its effectiveness relies heavily on the ability to predict the future state of health of the system, and for this survival models have shown to be very useful. Due to the complex behavior of system degradation, data-driven methods are often preferred, and neural network-based methods have been shown to perform particularly very well. Many neural network-based methods have been proposed and successfully applied to many problems. However, most models rely on assumptions that often are quite restrictive and there is an interest to find more expressive models. Energy-based models are promising candidates for this due to their successful use in other applications, which include natural language processing and computer vision. The focus of this work is therefore to investigate how energy-based models can be used for survival modeling and predictive maintenance. A key step in using energy-based models for survival modeling is the introduction of right-censored data, which, based on a maximum likelihood approach, is shown to be a straightforward process. Another important part of the model is the evaluation of the integral used to normalize the modeled probability density function, and it is shown how this can be done efficiently. The energy-based survival model is evaluated using both simulated data and experimental data in the form of starter battery failures from a fleet of vehicles, and its performance is found to be highly competitive compared to existing models. Code available at https://github.com/oholmer/PySaRe.
更多查看译文
关键词
survival models,maintenance,energy-based
AI 理解论文
溯源树
样例
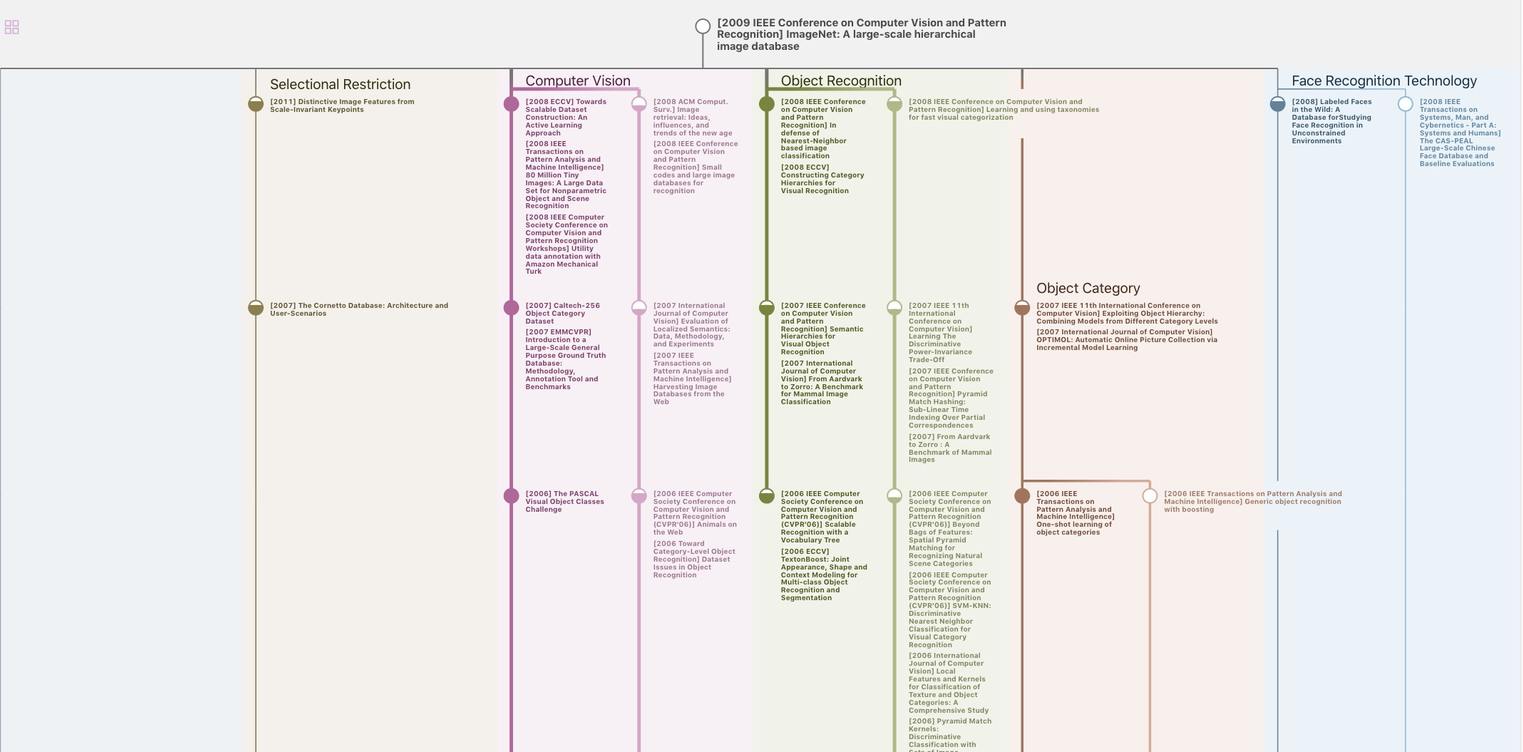
生成溯源树,研究论文发展脉络
Chat Paper
正在生成论文摘要