A Comprehensive Survey of Continual Learning: Theory, Method and Application.
arxiv(2024)
摘要
To cope with real-world dynamics, an intelligent system needs to incrementally acquire, update, accumulate, and exploit knowledge throughout its lifetime. This ability, known as continual learning, provides a foundation for AI systems to develop themselves adaptively. In a general sense, continual learning is explicitly limited by catastrophic forgetting, where learning a new task usually results in a dramatic performance drop of the old tasks. Beyond this, increasingly numerous advances have emerged in recent years that largely extend the understanding and application of continual learning. The growing and widespread interest in this direction demonstrates its realistic significance as well as complexity. In this work, we present a comprehensive survey of continual learning, seeking to bridge the basic settings, theoretical foundations, representative methods, and practical applications. Based on existing theoretical and empirical results, we summarize the general objectives of continual learning as ensuring a proper stability-plasticity trade-off and an adequate intra/inter-task generalizability in the context of resource efficiency. Then we provide a state-of-the-art and elaborated taxonomy, extensively analyzing how representative strategies address continual learning, and how they are adapted to particular challenges in various applications. Through an in-depth discussion of promising directions, we believe that such a holistic perspective can greatly facilitate subsequent exploration in this field and beyond.
更多查看译文
关键词
Continual learning,incremental learning,lifelong learning,catastrophic forgetting
AI 理解论文
溯源树
样例
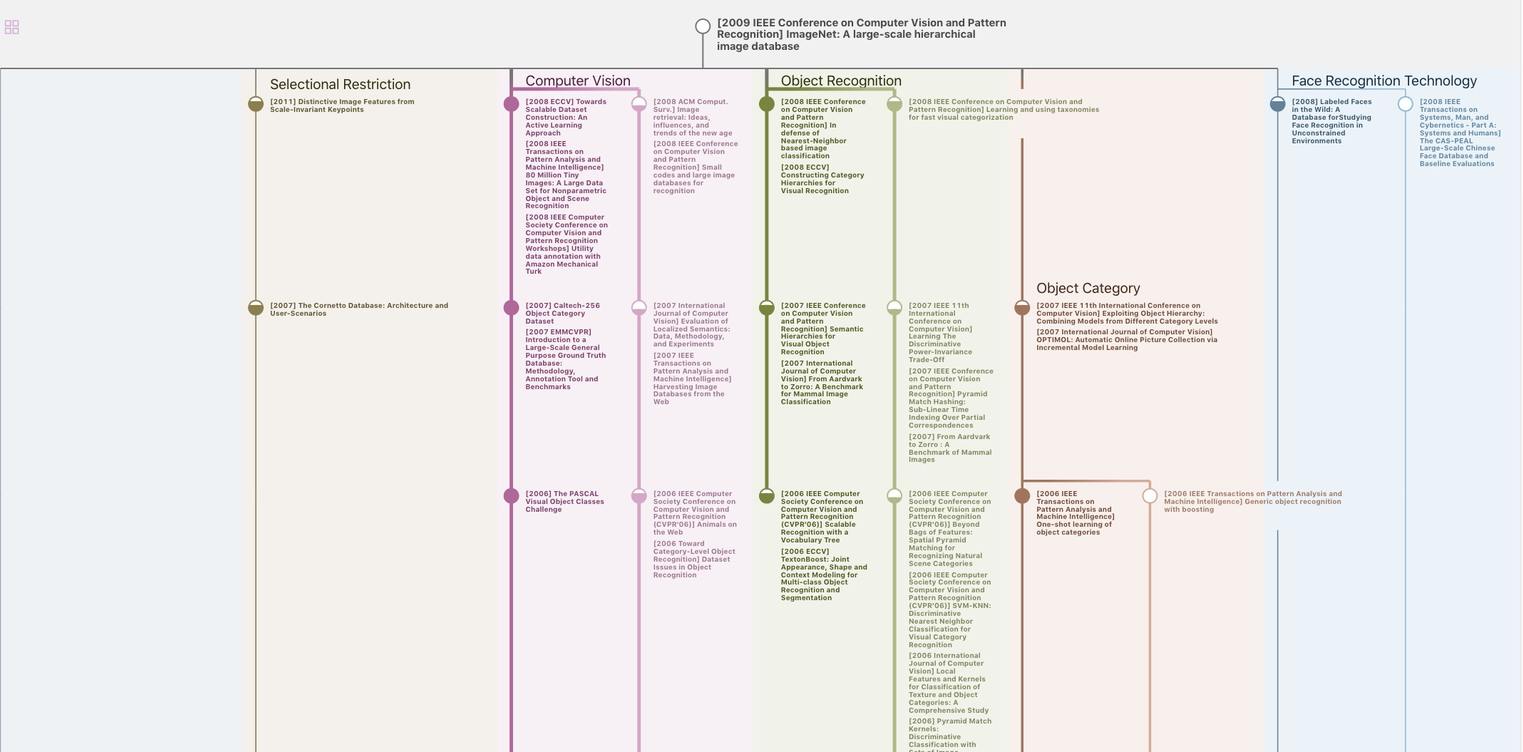
生成溯源树,研究论文发展脉络
Chat Paper
正在生成论文摘要