Toward a consistent performance evaluation for defect prediction models
arxiv(2023)
摘要
In defect prediction community, many defect prediction models have been proposed and indeed more new models are continuously being developed. However, there is no consensus on how to evaluate the performance of a newly proposed model. In this paper, we aim to propose MATTER, a fraMework towArd a consisTenT pErformance compaRison, which makes model performance directly comparable across different studies. We take three actions to build a consistent evaluation framework for defect prediction models. First, we propose a simple and easy-to-use unsupervised baseline model ONE (glObal baseliNe modEl) to provide "a single point of comparison". Second, we propose using the SQA-effort-aligned threshold setting to make a fair comparison. Third, we suggest reporting the evaluation results in a unified way and provide a set of core performance indicators for this purpose, thus enabling an across-study comparison to attain real progress. The experimental results show that MATTER can serve as an effective framework to support a consistent performance evaluation for defect prediction models and hence can help determine whether a newly proposed defect prediction model is practically useful for practitioners and inform the real progress in the road of defect prediction. Furthermore, when applying MATTER to evaluate the representative defect prediction models proposed in recent years, we find that most of them (if not all) are not superior to the simple baseline model ONE in terms of the SQA-effort awareness prediction performance. This reveals that the real progress in defect prediction has been overestimated. We hence recommend that, in future studies, when any new defect prediction model is proposed, MATTER should be used to evaluate its actual usefulness (on the same benchmark test data sets) to advance scientific progress in defect prediction.
更多查看译文
关键词
consistent performance evaluation,performance evaluation,prediction,models
AI 理解论文
溯源树
样例
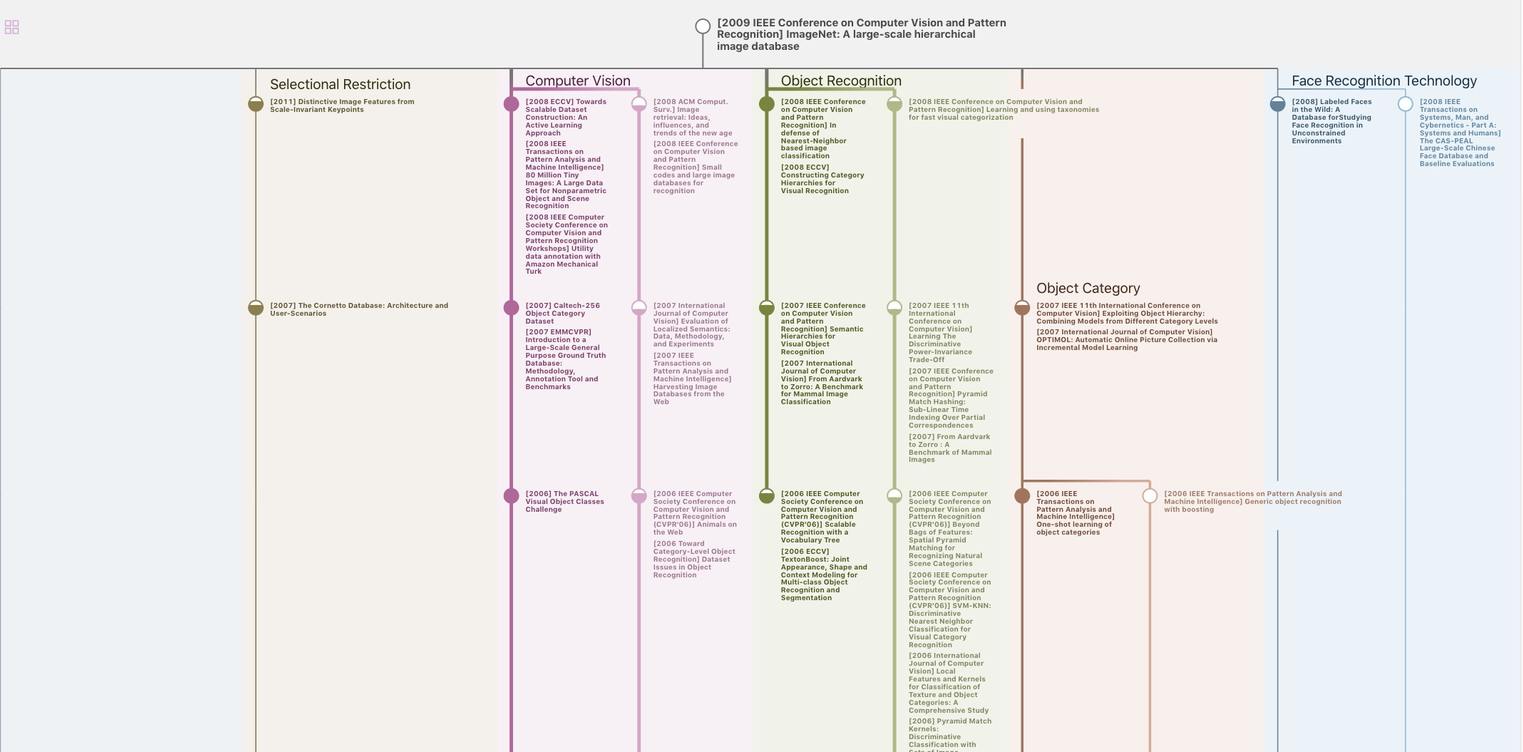
生成溯源树,研究论文发展脉络
Chat Paper
正在生成论文摘要