Accelerated First-Order Optimization under Nonlinear Constraints
arxiv(2023)
摘要
We exploit analogies between first-order algorithms for constrained optimization and non-smooth dynamical systems to design a new class of accelerated first-order algorithms for constrained optimization. Unlike Frank-Wolfe or projected gradients, these algorithms avoid optimization over the entire feasible set at each iteration. We prove convergence to stationary points even in a nonconvex setting and we derive rates for the convex setting. An important property of these algorithms is that constraints are expressed in terms of velocities instead of positions, which naturally leads to sparse, local and convex approximations of the feasible set (even if the feasible set is nonconvex). Thus, the complexity tends to grow mildly in the number of decision variables and in the number of constraints, which makes the algorithms suitable for machine learning applications. We apply our algorithms to a compressed sensing and a sparse regression problem, showing that we can treat nonconvex $\ell^p$ constraints ($p<1$) efficiently, while recovering state-of-the-art performance for $p=1$.
更多查看译文
关键词
nonlinear constraints,optimization,first-order
AI 理解论文
溯源树
样例
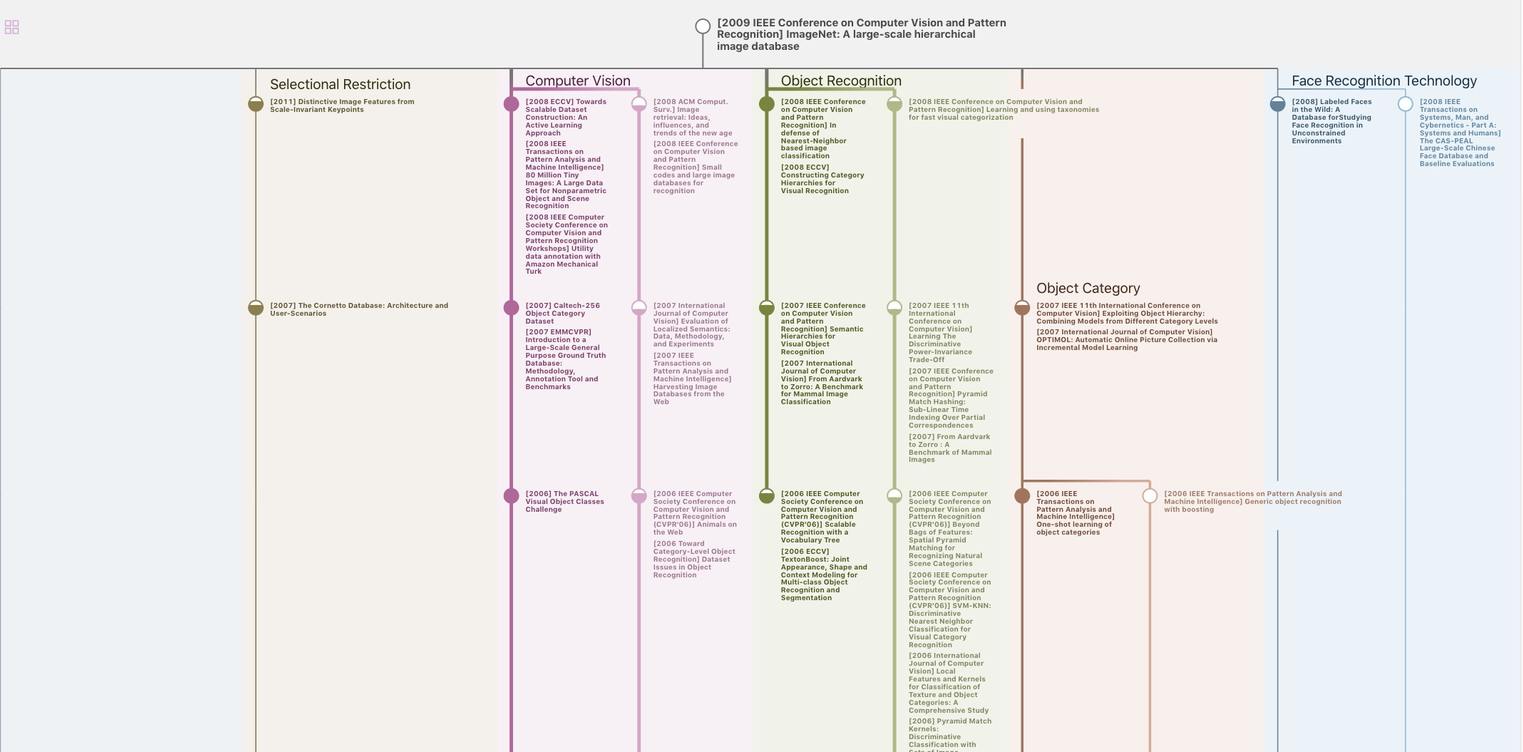
生成溯源树,研究论文发展脉络
Chat Paper
正在生成论文摘要