Beam Selection for Energy-Efficient mmWave Network Using Advantage Actor Critic Learning
ICC 2023 - IEEE INTERNATIONAL CONFERENCE ON COMMUNICATIONS(2023)
摘要
The growing adoption of mmWave frequency bands to realize the full potential of 5G, turns beamforming into a key enabler for current and next-generation wireless technologies. Many mmWave networks rely on beam selection with Grid-of-Beams (GoB) approach to handle user-beam association. In beam selection with GoB, users select the appropriate beam from a set of pre-defined beams and the overhead during the beam selection process is a common challenge in this area. In this paper, we propose an Advantage Actor Critic (A2C) learning-based framework to improve the GoB and the beam selection process, as well as optimize transmission power in a mmWave network. The proposed beam selection technique allows performance improvement while considering transmission power improves Energy Efficiency (EE) and ensures the coverage is maintained in the network. We further investigate how the proposed algorithm can be deployed in a Service Management and Orchestration (SMO) platform. Our simulations show that A2C-based joint optimization of beam selection and transmission power is more effective than using Equally Spaced Beams (ESB) and fixed power strategy, or optimization of beam selection and transmission power disjointly. Compared to the ESB and fixed transmission power strategy, the proposed approach achieves more than twice the average EE in the scenarios under test and is closer to the maximum theoretical EE.
更多查看译文
关键词
beamforming,beam selection,energy efficiency,reinforcement learning,advantage actor critic
AI 理解论文
溯源树
样例
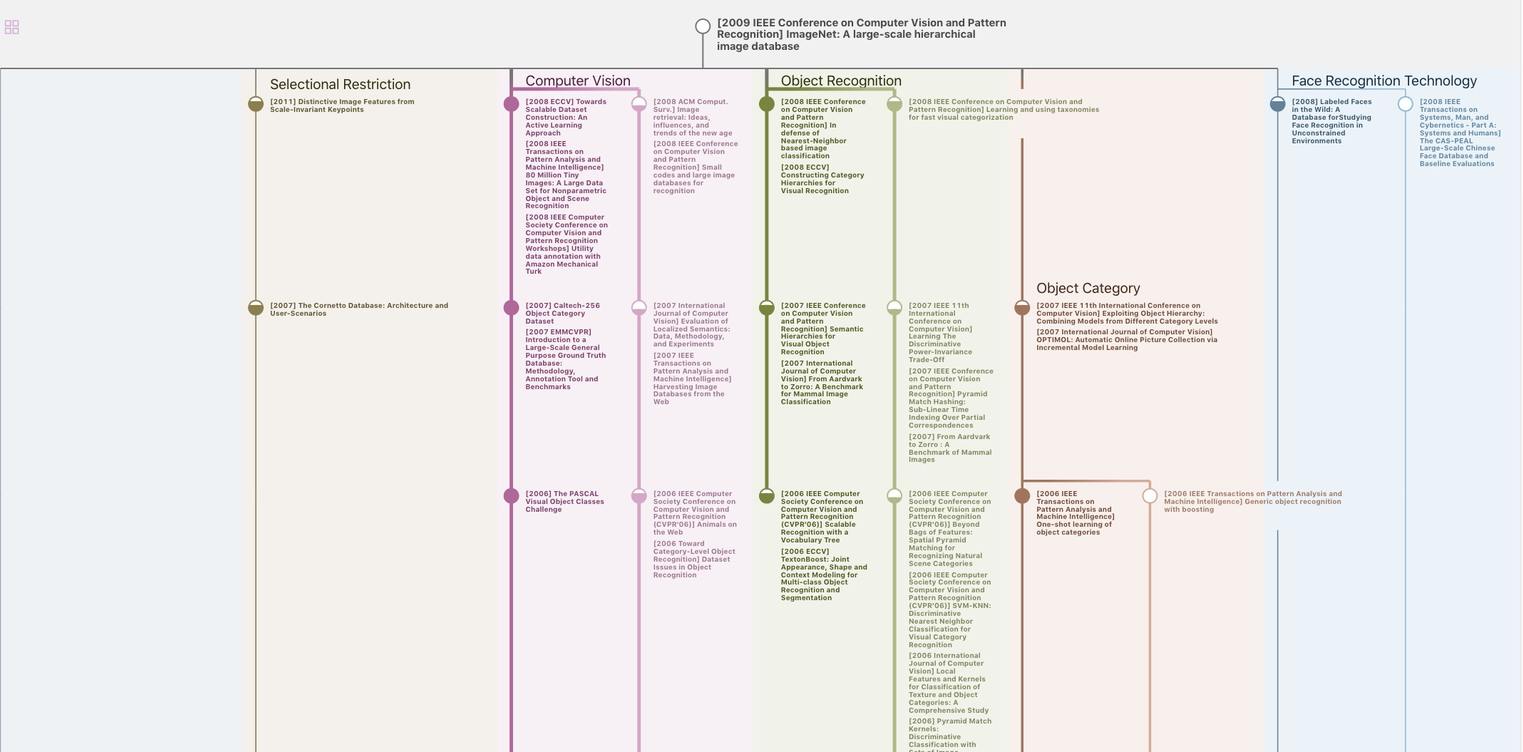
生成溯源树,研究论文发展脉络
Chat Paper
正在生成论文摘要