Debiasing Vision-Language Models via Biased Prompts
arxiv(2023)
摘要
Machine learning models have been shown to inherit biases from their training datasets, which can be particularly problematic for vision-language foundation models trained on uncurated datasets scraped from the internet. The biases can be amplified and propagated to downstream applications like zero-shot classifiers and text-to-image generative models. In this study, we propose a general approach for debiasing vision-language foundation models by projecting out biased directions in the text embedding. In particular, we show that debiasing only the text embedding with a calibrated projection matrix suffices to yield robust classifiers and fair generative models. The closed-form solution enables easy integration into large-scale pipelines, and empirical results demonstrate that our approach effectively reduces social bias and spurious correlation in both discriminative and generative vision-language models without the need for additional data or training.
更多查看译文
关键词
biased prompts,models,vision-language
AI 理解论文
溯源树
样例
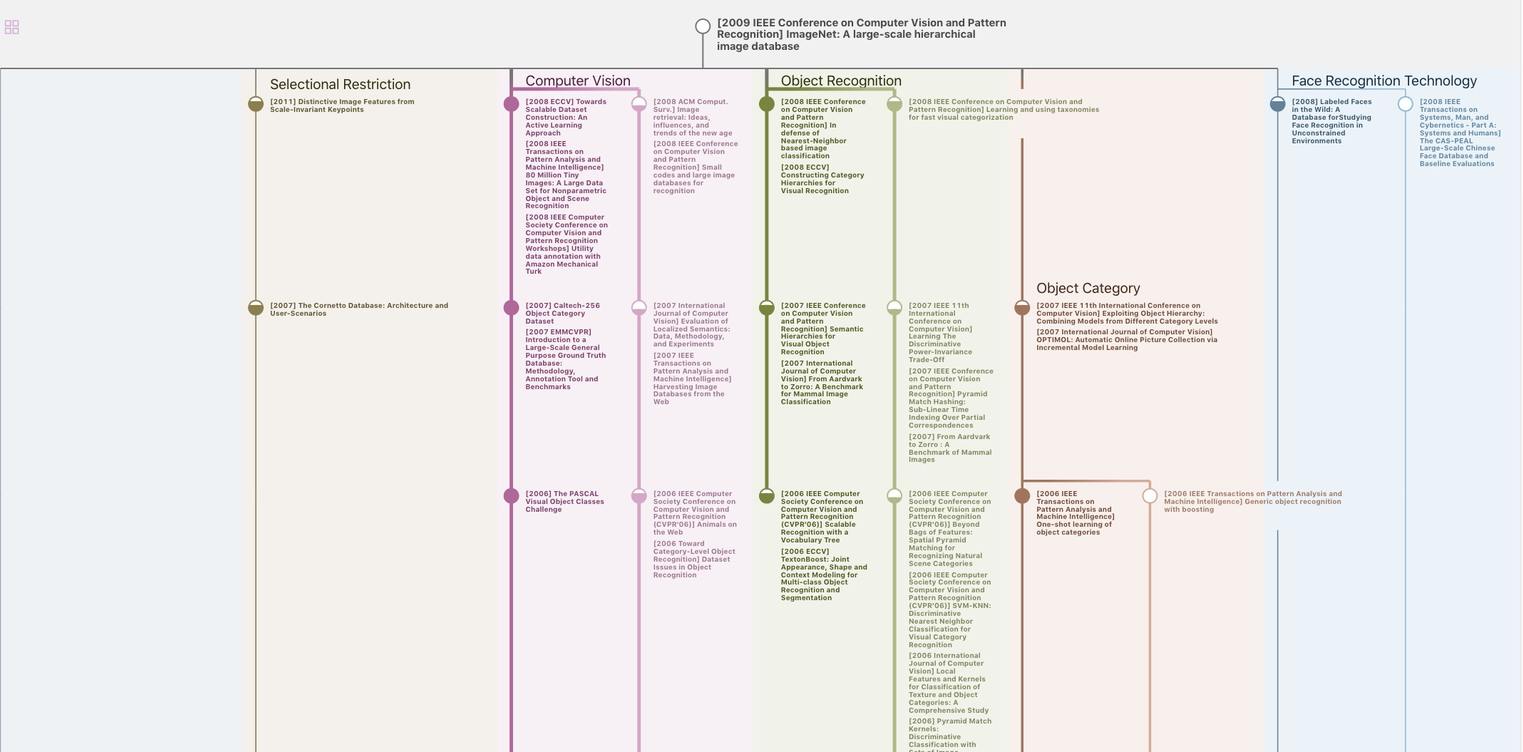
生成溯源树,研究论文发展脉络
Chat Paper
正在生成论文摘要