Transformers Meet Directed Graphs
arxiv(2023)
摘要
Transformers were originally proposed as a sequence-to-sequence model for text but have become vital for a wide range of modalities, including images, audio, video, and undirected graphs. However, transformers for directed graphs are a surprisingly underexplored topic, despite their applicability to ubiquitous domains including source code and logic circuits. In this work, we propose two direction- and structure-aware positional encodings for directed graphs: (1) the eigenvectors of the Magnetic Laplacian - a direction-aware generalization of the combinatorial Laplacian; (2) directional random walk encodings. Empirically, we show that the extra directionality information is useful in various downstream tasks, including correctness testing of sorting networks and source code understanding. Together with a data-flow-centric graph construction, our model outperforms the prior state of the art on the Open Graph Benchmark Code2 relatively by 14.7%.
更多查看译文
关键词
graphs
AI 理解论文
溯源树
样例
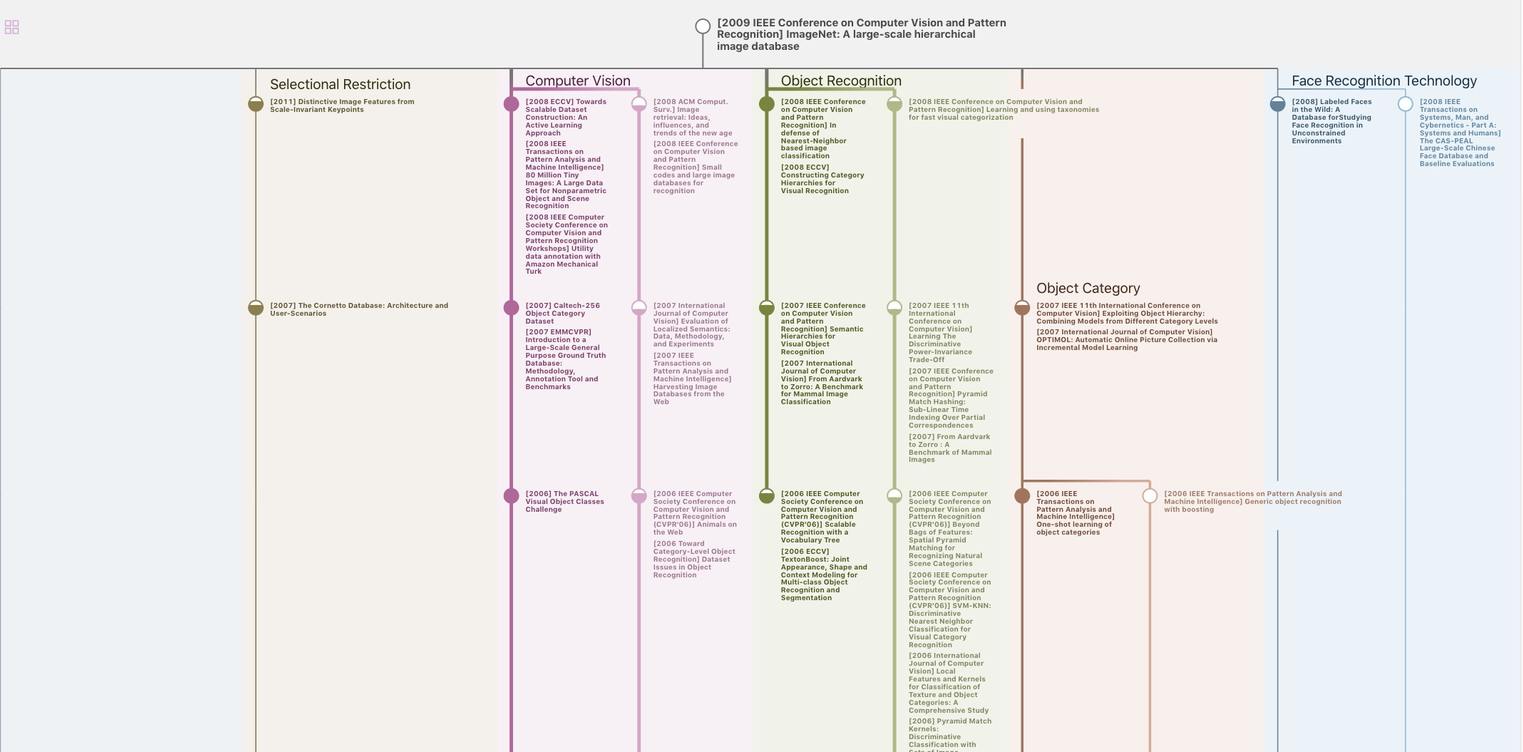
生成溯源树,研究论文发展脉络
Chat Paper
正在生成论文摘要