Reducing Blackwell and Average Optimality to Discounted MDPs via the Blackwell Discount Factor
CoRR(2023)
摘要
We introduce the Blackwell discount factor for Markov Decision Processes (MDPs). Classical objectives for MDPs include discounted, average, and Blackwell optimality. Many existing approaches to computing average-optimal policies solve for discounted optimal policies with a discount factor close to $1$, but they only work under strong or hard-to-verify assumptions such as ergodicity or weakly communicating MDPs. In this paper, we show that when the discount factor is larger than the Blackwell discount factor $\gamma_{\mathrm{bw}}$, all discounted optimal policies become Blackwell- and average-optimal, and we derive a general upper bound on $\gamma_{\mathrm{bw}}$. The upper bound on $\gamma_{\mathrm{bw}}$ provides the first reduction from average and Blackwell optimality to discounted optimality, without any assumptions, and new polynomial-time algorithms for average- and Blackwell-optimal policies. Our work brings new ideas from the study of polynomials and algebraic numbers to the analysis of MDPs. Our results also apply to robust MDPs, enabling the first algorithms to compute robust Blackwell-optimal policies.
更多查看译文
关键词
discounted mdps,average optimality,blackwell
AI 理解论文
溯源树
样例
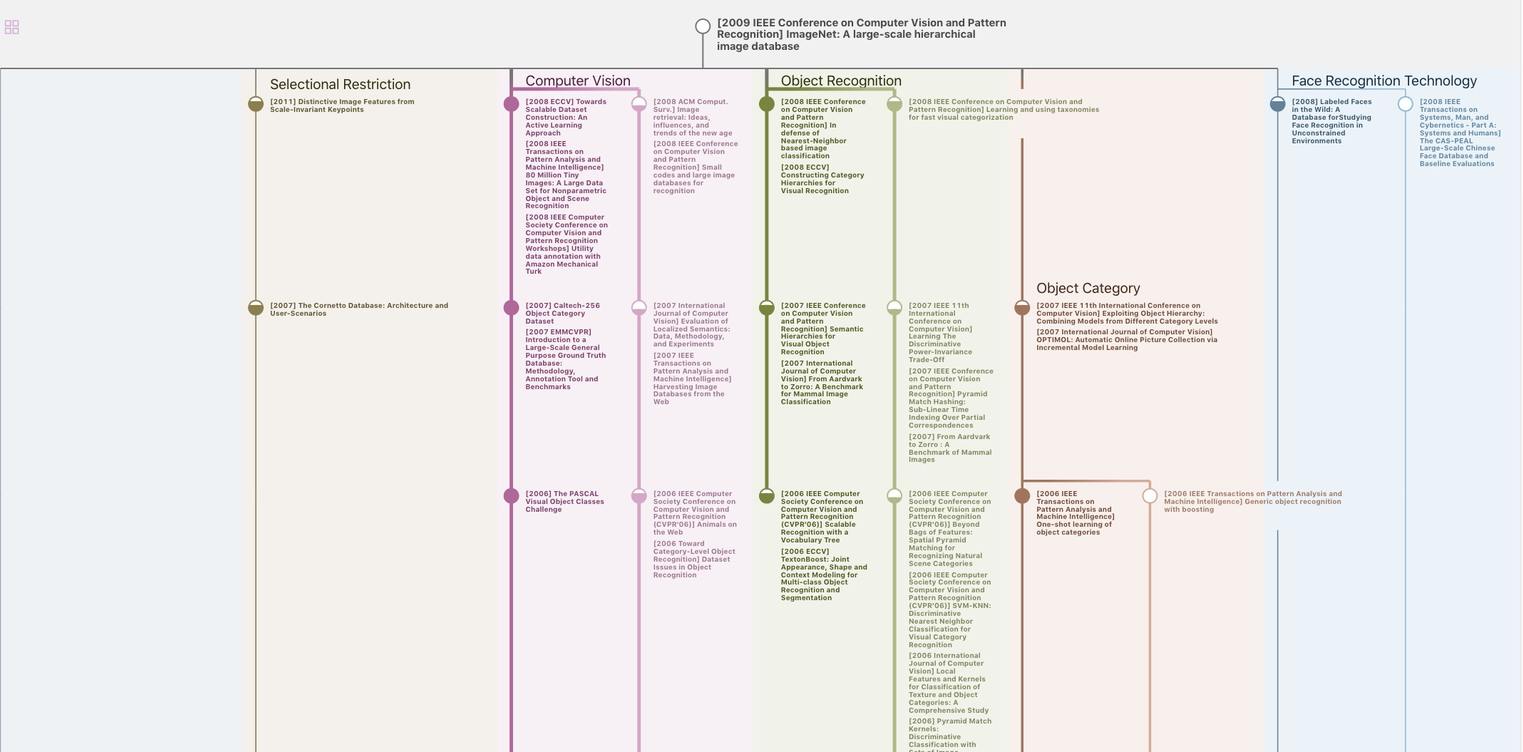
生成溯源树,研究论文发展脉络
Chat Paper
正在生成论文摘要