Evaluation of generated synthetic OCT images in deep‐learning models for glaucoma detection
Acta Ophthalmologica(2022)
摘要
Abstract Purpose: To evaluate the use of synthetically generated OCT images for the development of deep‐learning models in glaucoma detection. Methods: Progressively Grown Generative Adversarial Network (PGGAN) models for glaucoma and healthy eyes were developed with data from 862 Asian glaucoma eyes and 990 Asian normal eyes to generate synthetic circumpapillary OCT images. Glaucoma detection deep‐learning models were trained using 1200, 10 000, 60 000 or 200 000 of the generated images, equally split between glaucoma and normal. Detection performance was evaluated on real images from an Asian dataset of 140 eyes from 112 subjects and a Caucasian dataset of 300 eyes from 160 subjects, with half of each being glaucoma. Results were compared with a glaucoma detection model trained with real images from 600 glaucoma and 600 healthy eyes, and with global retinal nerve fibre layer (RNFL) measurements using Area Under the Curve (AUC) analysis. Results: Glaucoma detection performance improved with increasing synthetic dataset size, from an AUC of 0.945 [95% CI: 0.917–0.974] and 0.856 [95% CI: 0.819–0.889] on the Asian and Caucasian test data respectively when 1200 synthetic images were used, to AUCs of 0.969 [95% CI: 0.949–0.987] and 0.897 [95% CI: 0.867–0.927] when 200 000 synthetic images were used. The model trained on 200 000 synthetic images was significantly better ( p < 0.05) than the model trained on real images on the Caucasian test data (0.838 [95% CI: 0.800–0.874]) but not on the Asian test data (0.962 [95% CI: 0.939–0.985]). Both detection models showed significantly better AUCs than that of global RNFL for both Asian (0.920 [95% CI: 0.884–0.957]) and Caucasian (0.833 [95% CI: 0.793–0.869]) test datasets. Conclusions: Synthetically circumpapillary OCT images used to train deep‐learning glaucoma detection models showed similar performance to a model trained with real data, and could potentially be used to facilitate sharing of imaging data while addressing privacy considerations.
更多查看译文
关键词
glaucoma detection,deep‐learning deep‐learning models,deep‐learning deep‐learning,images
AI 理解论文
溯源树
样例
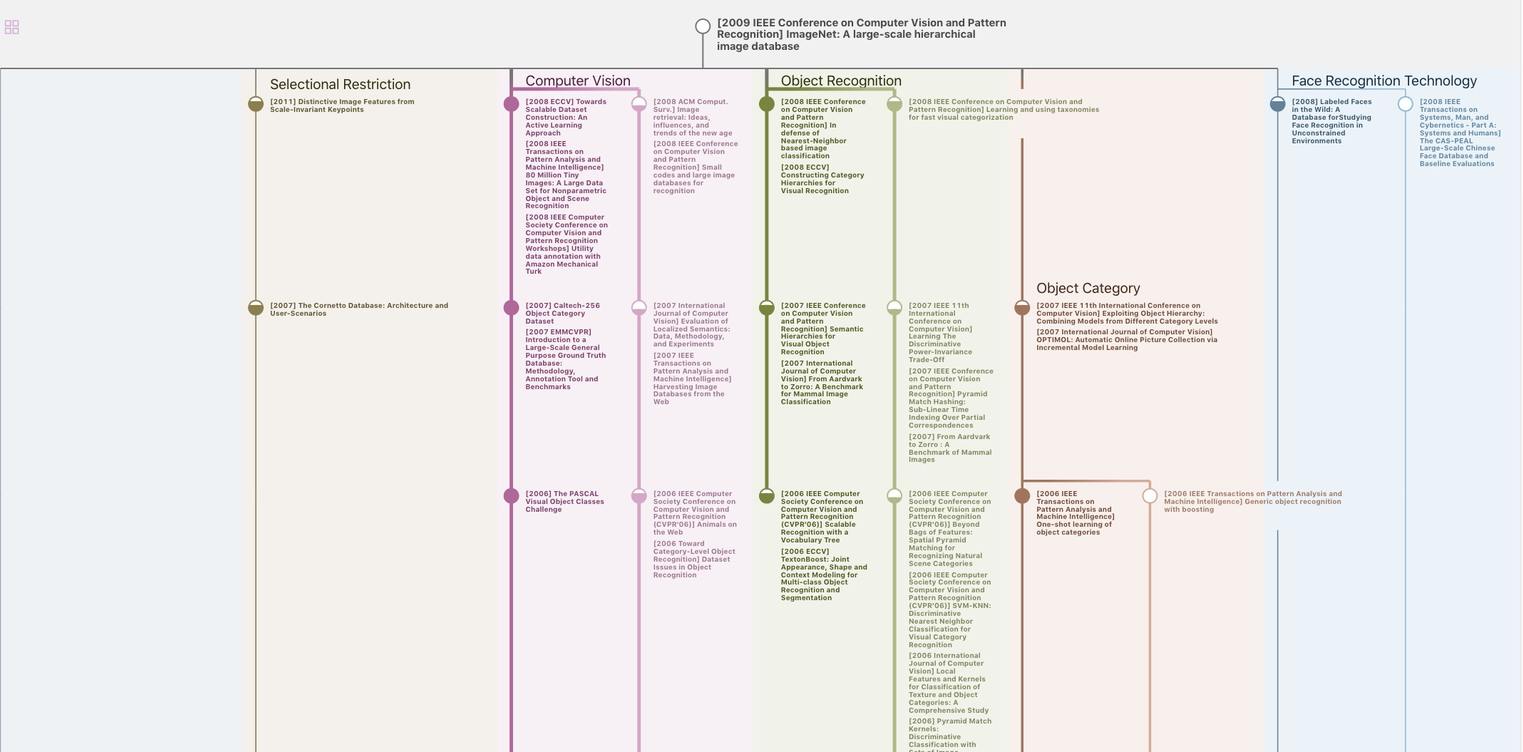
生成溯源树,研究论文发展脉络
Chat Paper
正在生成论文摘要