Holistic Graph-based Motion Prediction
2023 IEEE INTERNATIONAL CONFERENCE ON ROBOTICS AND AUTOMATION, ICRA(2023)
摘要
Motion prediction for automated vehicles in complex environments is a difficult task that is to be mastered when automated vehicles are to be used in arbitrary situations. Many factors influence the future motion of traffic participants starting with traffic rules and reaching from the interaction between each other to personal habits of human drivers. Therefore, we present a novel approach for a graph-based prediction based on a heterogeneous holistic graph representation that combines temporal information, properties and relations between traffic participants as well as relations with static elements such as the road network. The information is encoded through different types of nodes and edges that both are enriched with arbitrary features. We evaluated the approach on the INTERACTION and the Argoverse dataset and conducted an informative ablation study to demonstrate the benefit of different types of information for the motion prediction quality.
更多查看译文
关键词
arbitrary features,arbitrary situations,Argoverse dataset,automated vehicles,complex environments,future motion,graph-based prediction,heterogeneous holistic graph representation,holistic graph-based motion prediction,human drivers,informative ablation study,motion prediction quality,personal habits,temporal information,traffic participants,traffic rules
AI 理解论文
溯源树
样例
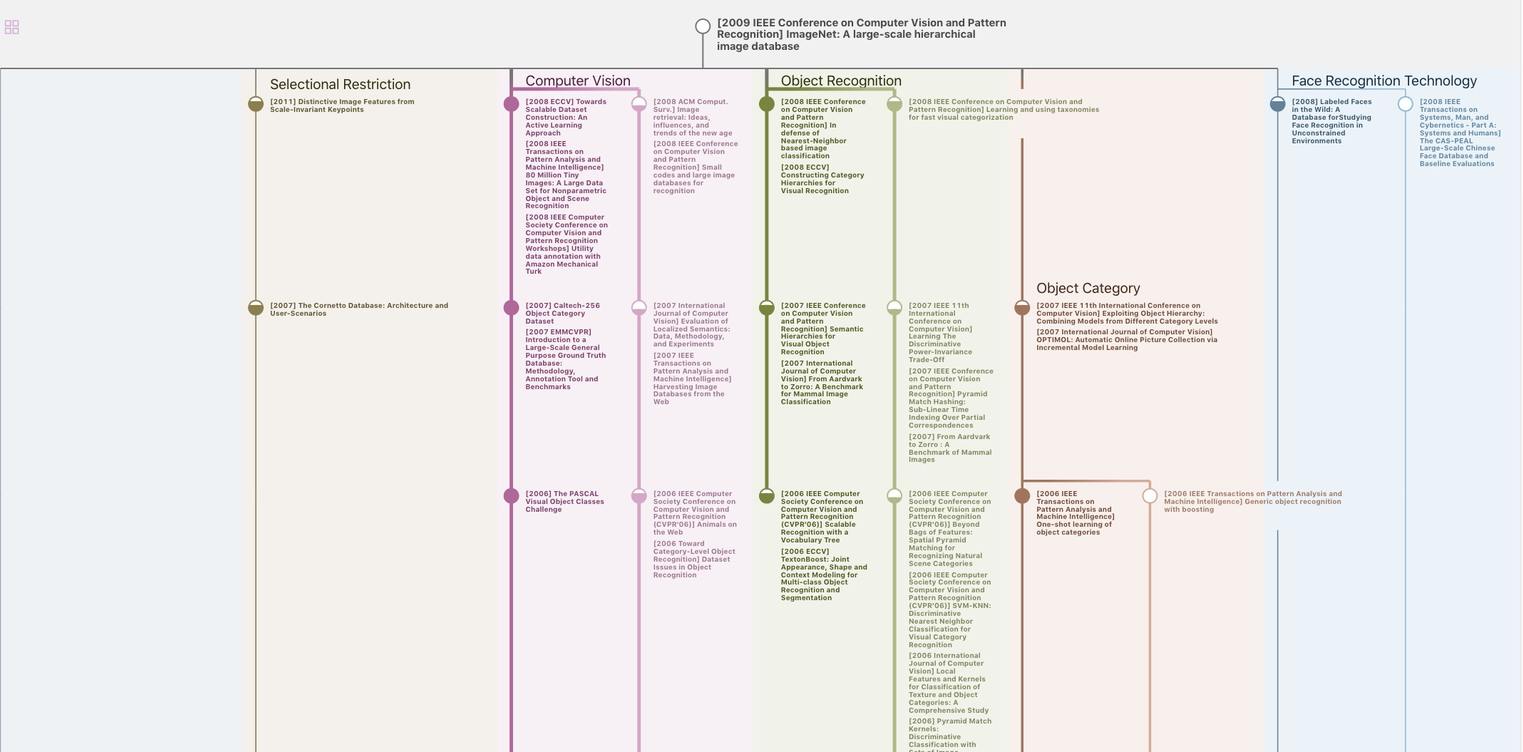
生成溯源树,研究论文发展脉络
Chat Paper
正在生成论文摘要