Sharp Variance-Dependent Bounds in Reinforcement Learning: Best of Both Worlds in Stochastic and Deterministic Environments
arxiv(2023)
摘要
We study variance-dependent regret bounds for Markov decision processes (MDPs). Algorithms with variance-dependent regret guarantees can automatically exploit environments with low variance (e.g., enjoying constant regret on deterministic MDPs). The existing algorithms are either variance-independent or suboptimal. We first propose two new environment norms to characterize the fine-grained variance properties of the environment. For model-based methods, we design a variant of the MVP algorithm (Zhang et al., 2021a) and use new analysis techniques show to this algorithm enjoys variance-dependent bounds with respect to our proposed norms. In particular, this bound is simultaneously minimax optimal for both stochastic and deterministic MDPs, the first result of its kind. We further initiate the study on model-free algorithms with variance-dependent regret bounds by designing a reference-function-based algorithm with a novel capped-doubling reference update schedule. Lastly, we also provide lower bounds to complement our upper bounds.
更多查看译文
关键词
reinforcement learning,stochastic,deterministic environments,variance-dependent
AI 理解论文
溯源树
样例
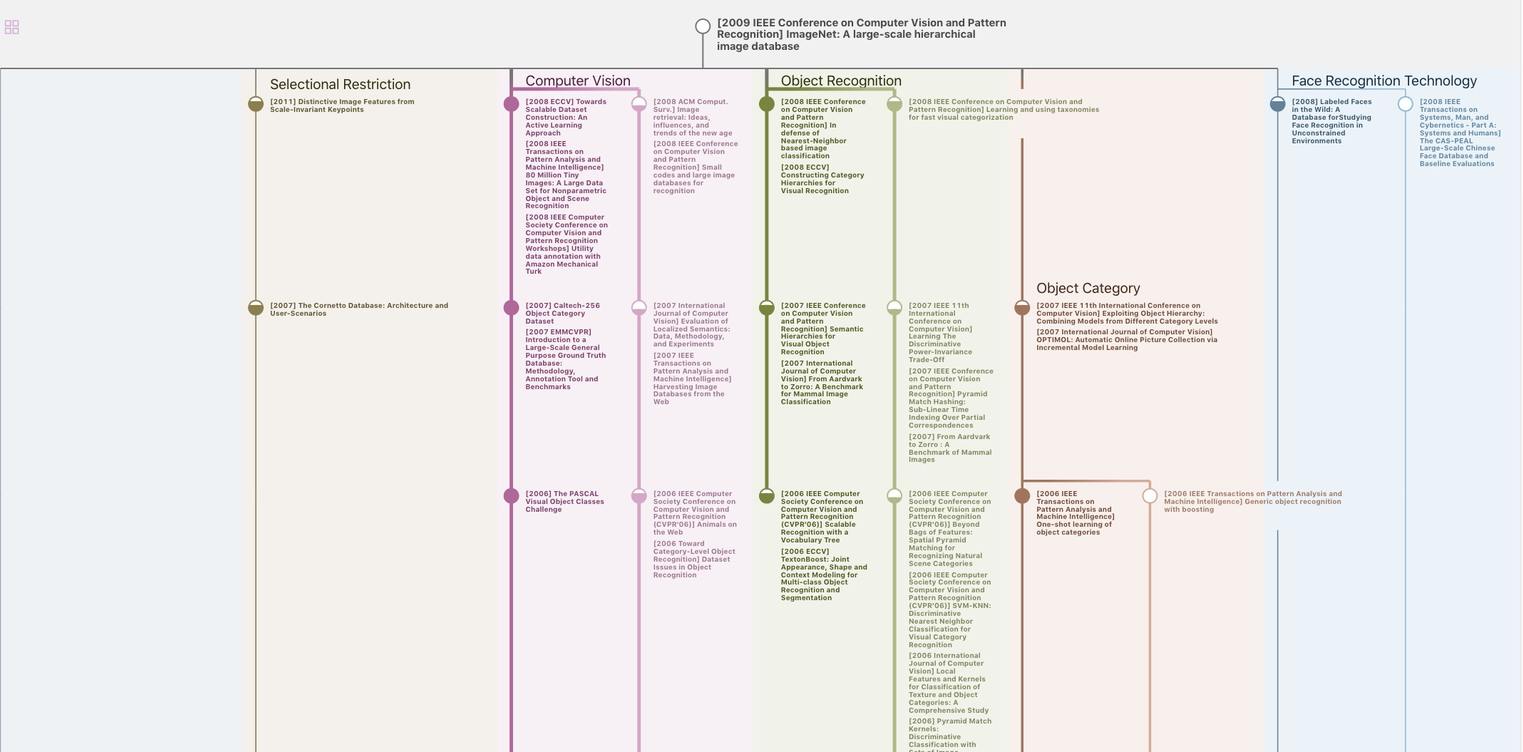
生成溯源树,研究论文发展脉络
Chat Paper
正在生成论文摘要